Detection and identification of plant leaf diseases using YOLOv4.
Frontiers in plant science(2024)
摘要
Detecting plant leaf diseases accurately and promptly is essential for reducing economic consequences and maximizing crop yield. However, farmers' dependence on conventional manual techniques presents a difficulty in accurately pinpointing particular diseases. This research investigates the utilization of the YOLOv4 algorithm for detecting and identifying plant leaf diseases. This study uses the comprehensive Plant Village Dataset, which includes over fifty thousand photos of healthy and diseased plant leaves from fourteen different species, to develop advanced disease prediction systems in agriculture. Data augmentation techniques including histogram equalization and horizontal flip were used to improve the dataset and strengthen the model's resilience. A comprehensive assessment of the YOLOv4 algorithm was conducted, which involved comparing its performance with established target identification methods including Densenet, Alexanet, and neural networks. When YOLOv4 was used on the Plant Village dataset, it achieved an impressive accuracy of 99.99%. The evaluation criteria, including accuracy, precision, recall, and f1-score, consistently showed high performance with a value of 0.99, confirming the effectiveness of the proposed methodology. This study's results demonstrate substantial advancements in plant disease detection and underscore the capabilities of YOLOv4 as a sophisticated tool for accurate disease prediction. These developments have significant significance for everyone involved in agriculture, researchers, and farmers, providing improved capacities for disease control and crop protection.
更多查看译文
关键词
plant leaf disease,leaf disease detection,object detection,deep-learning,YOLO v4,darknet
AI 理解论文
溯源树
样例
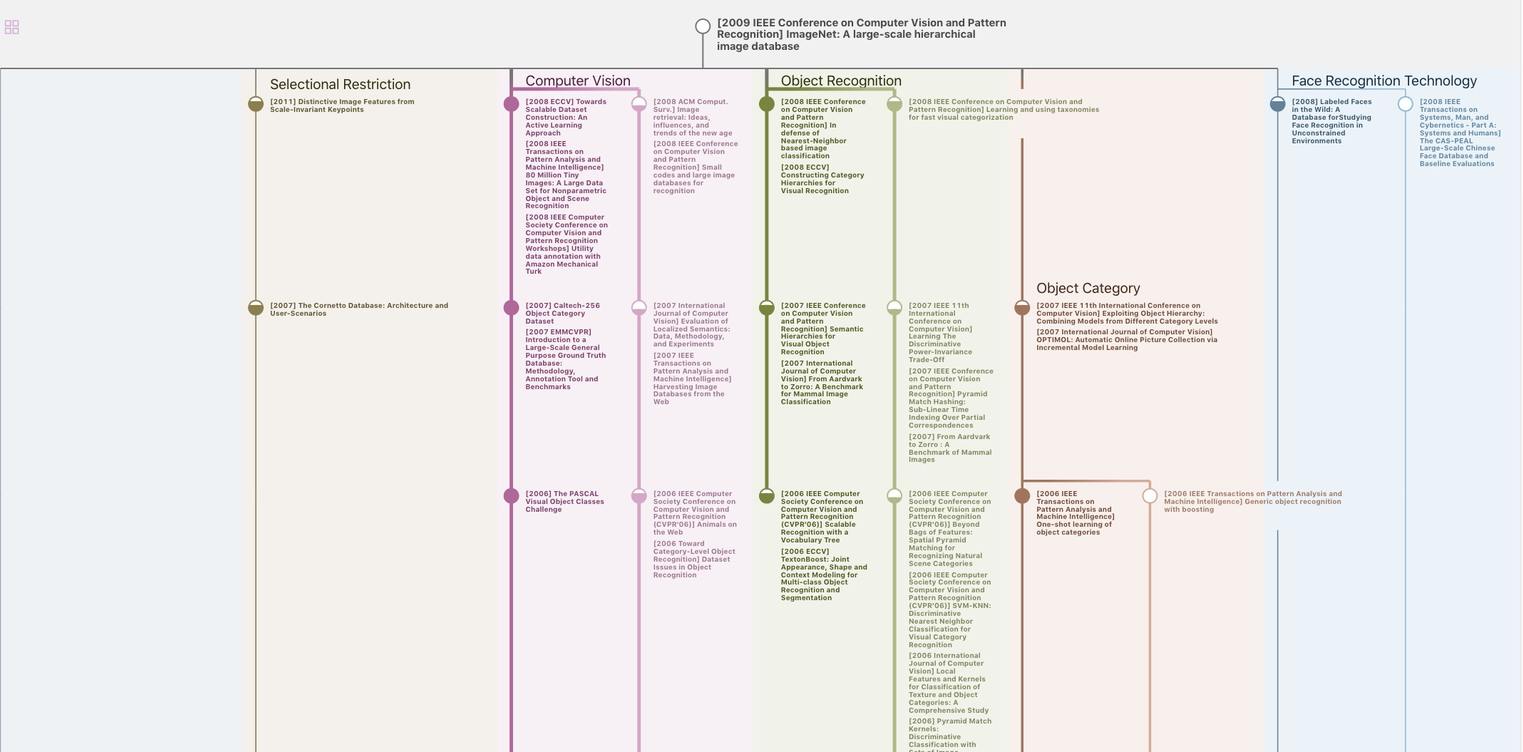
生成溯源树,研究论文发展脉络
Chat Paper
正在生成论文摘要