0521 Validation of Deep Learning Algorithm Model in Automatic Scoring of the Respiratory Events in Adult Polysomnography
SLEEP(2024)
摘要
Abstract Introduction Background: Polysomnography (PSG) has several benefits as a conventional diagnostic tool for identifying and assessing a range of sleep disorders, with particular emphasis on obstructive sleep apnea (OSA). Nevertheless, the process of scoring raw data requires a significant amount of effort and time. Hence, the objective of this research was to assess the precision of a recently devised automated scoring system for respiratory events, using a deep learning algorithm model, in the context of people afflicted with sleep-disordered breathing (SDB). Methods A total of 1,000 case PSG data were enrolled to develop a deep learning algorithm. Of the 1,000 data, 700 were distributed for training, 200 for validation, and 100 (simple snoring, mild, moderate, and severe OSA; n = 25 in each group) for testing. The respiratory events scoring deep learning model is composed of five sequential layers: an initial layer of perceptrons, followed by three consecutive layers of long short-term memory cells, and ultimately, an additional two layers of perceptrons. Results The PSG data of 100 patients (mean age 50.59 ± 14.01 years; 55 men and 45 women; body mass index 26.30 ± 3.98) were selected for validation and testing of the deep learning model, which included simple snoring, mild, moderate, and severe OSA groups (n = 25 each). Our deep learning algorithm had a sensitivity (95% confidence interval [CI]) of 98.06 (96.64-99.53), 98.12 (96.68-99.87), 98.23 (96.75-99.72), and 98.51 (96.2-95.91) for identifying apnea or hypopnea events. The specificity (95% CI) of simple snoring, mild OSA, moderate OSA, and severe OSA was 97.79 (92.84-99.95), 96.84 (93.6-99.95), 96.7 (93.32-99.59), and 95.46 (93.71-99.08) compared to a manually graded PSG report. The deep learning model's area under the curve values for predicting OSA in apnea-hypopnea index ≥ 5, 15, and 30 groups were 0.9402, 0.9388, and 0.9442, respectively, showing no significant differences between each group. Conclusion The deep learning algorithm used in our work demonstrated a notable accuracy in recognizing apnea/hypopnea episodes and subsequently categorizing the severity of OSA. Support (if any) This work was supported by the Technology Development Program (S2718665), which is funded by the Ministry of SMEs and Startups of Korea and Soonchunhyang University Research Fund.
更多查看译文
AI 理解论文
溯源树
样例
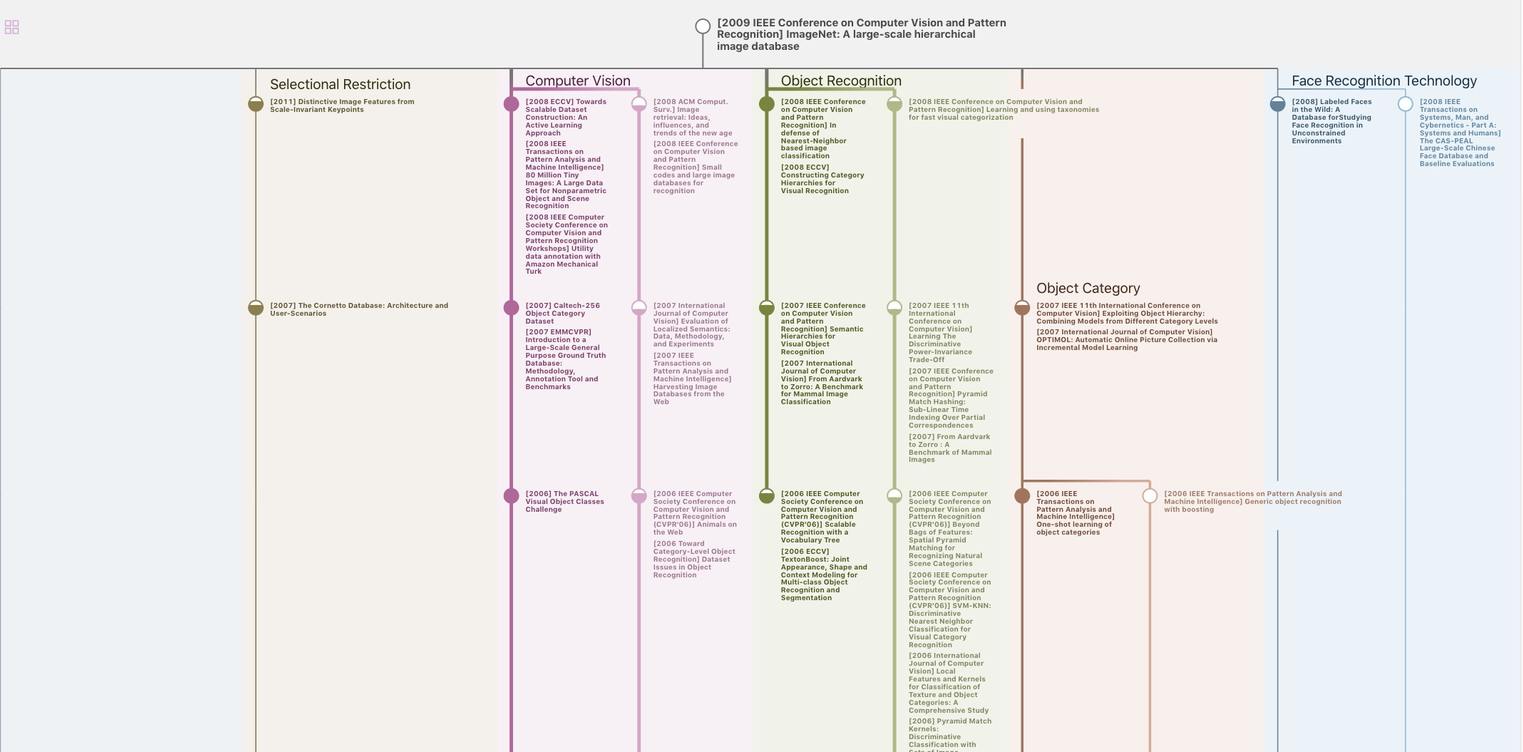
生成溯源树,研究论文发展脉络
Chat Paper
正在生成论文摘要