0303 Predicting Subjective Sleep Impairment and Disturbance from Wearable Sleep Data
SLEEP(2024)
摘要
Abstract Introduction Wearables offer scalable, passive and objective measures of sleep, but how well do they capture feelings of sleep disturbance and impairment? We studied a large, diverse group (n=2992 adults) using wearables and compared sleep metrics to self-reported sleep disturbance and impairment. Methods Participants in the Digital Wellbeing Study wore one of several wearable devices (Fitbit) capable of tracking objective sleep metrics such as Total Sleep Time (TST), bedtime, wake-up time, estimated sleep stage durations etc. over a 4-week period under normal free-living conditions. Self-reported sleep questionnaires were administered at enrollment and at study-end. The wearable-derived objective sleep metrics were summarized across the 7 days prior to administration of the study-end PROMIS Sleep Disturbance (SD) and Sleep-Related Impairment (SI) Short Form surveys. Stepwise Ordinary Least Squares (OLS) regressions were then used to test the predictive power of the objective sleep metrics to estimate the raw survey scores (and compared to predictions from a baseline model of age and sex only). Results Sleep variables of TST, resting heart rate, and the variability in total sleep time and restlessness improved both SI and SD above the baseline model. Minutes-in-deep-sleep improved the SI model fit, while longest wake length and total wake minutes improved SD fit. REM percent and normalized nightly heart rate did not improve model fit. The final model combining the set of objective sleep metrics explained 12.9% of the variance of SI (+4.2% over baseline), and 8.4% of the variance of SD (+6.0% over baseline). The most predictive single sleep metric was the variability in total sleep time for SI, and total sleep time for SD. Conclusion Previous studies using polysomnography (PSG) or actigraphy derived objective sleep metrics found only modest predictive power of self-reported sleep satisfaction measures (e.g., correlations of 0.3-0.46). This study showed that longitudinal free-living sleep data from wearables provided a similar moderate level of prediction for two self-reported sleep questionnaires. Composite metrics that include measures of sleep variability may provide some additional insight into sleep-related impairment. Support (if any) This research was funded by Google Inc.
更多查看译文
AI 理解论文
溯源树
样例
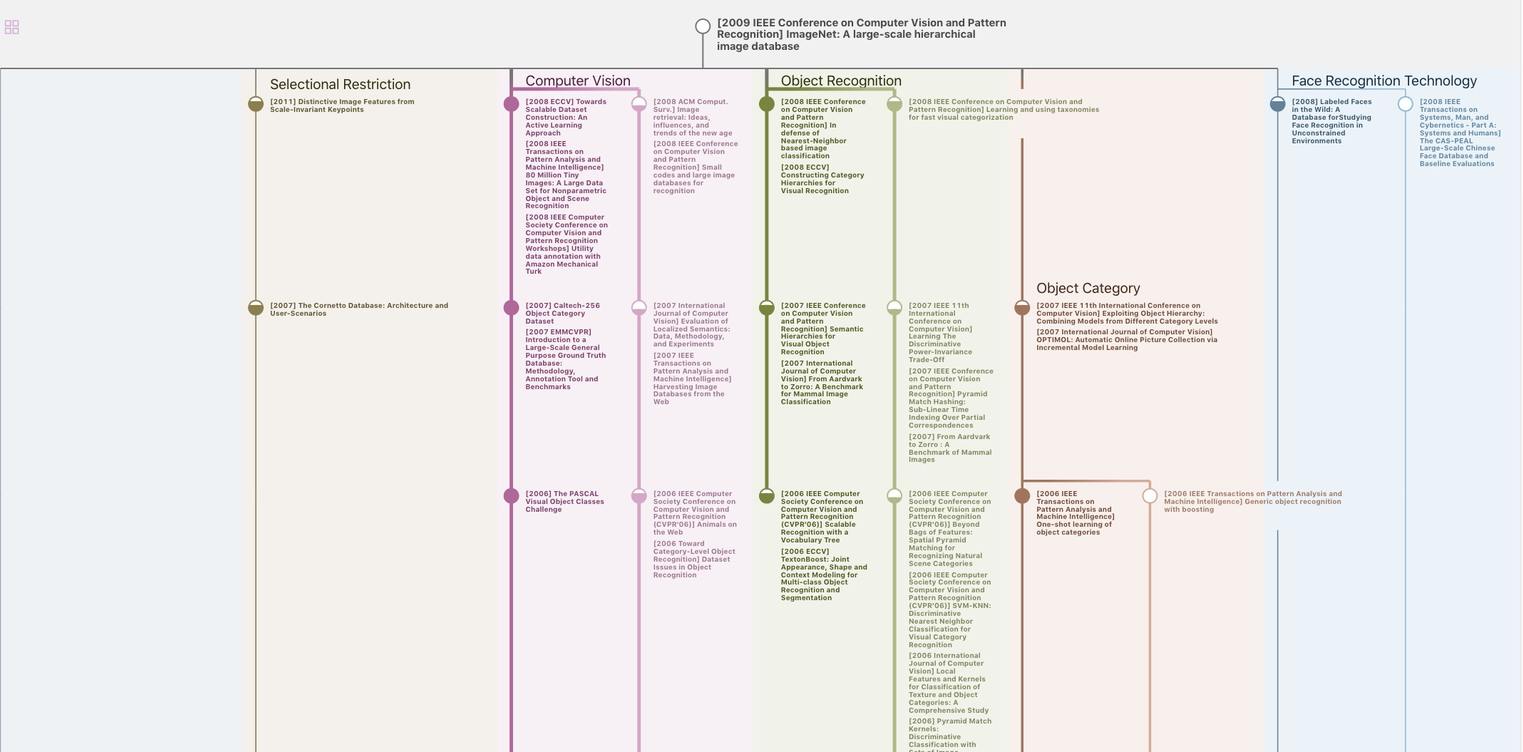
生成溯源树,研究论文发展脉络
Chat Paper
正在生成论文摘要