0319 Using Generative AI as a Tool for Simulating EEG During Nocturnal Polysomnography
SLEEP(2024)
摘要
Abstract Introduction Artificial Intelligence based Generative Networks based synthetic data generation has become increasingly popular. Deep Learning Generative models can be trained to model complex data distributions and generate diverse and realistic outputs. We aim to present a generative model that can generate an artificial 30-second epoch of non-REM sleep whose power spectrum is identical to that of a real sleep EEG. Methods The data used to train the network was obtained from multiple healthy subjects of the Multi-Ethnic Study of Atherosclerosis (MESA), an NHLBI-sponsored 6-center collaborative longitudinal investigation made available through the National Sleep Research Resource (NSRR). We selected 660 subjects deemed healthy and devoid of any sleep disorders. The EEG was initially recorded at 256 Hz and down sampled to 64 Hz for this study. Only the Fz-Cz channel from the recorded EEG channels was used to train the Generative model. A Deep Learning Generative Adversarial Network was trained using 30s epochs of the NREM Stage 3 EEG data from 660 subjects. Both visual inspection and quantitative metrics were used to evaluate the synthetic signals. The metric to assess Generative Model performance was the relative spectral power (%) in different frequency bands compared to real EEG signals. The averages for the real EEG signals were calculated after taking the average frequency band power across all epochs used for training. The band-power of 100 generated samples, visually deemed good quality, was used to calculate the average for generated EEG signals. Results Across Alpha, Delta, Beta, Theta and Sigma bands, there was minimal difference in % band power between synthetic and real EEG signals: Alpha real[0.5±0.14] / Alpha synthetic[2.46±0.52], Beta real[0.1±0.03] / Beta synthetic[0.058±0.03] , Theta real[1.8±0.78] / Theta synthetic[4.18±0.9], Delta real[97.0±1.26] / Delta synthetic[93.2.0±2.25] and Sigma real[0.6±0.04] / Sigma synthetic[0.084±0.01] Conclusion The developed system has the potential to be a sleep EEG simulator used as an educational tool to train fellows and technicians across the field of sleep medicine. Future studies are needed to accommodate multichannel EEG signals and other PSG signals (e.g., respiratory, Spo2 etc.) to simulate an entire nocturnal polysomnography. Support (if any) This work is supported by NIH R21HL165320, NIH K25HL151912 and NIH R21HL165320
更多查看译文
AI 理解论文
溯源树
样例
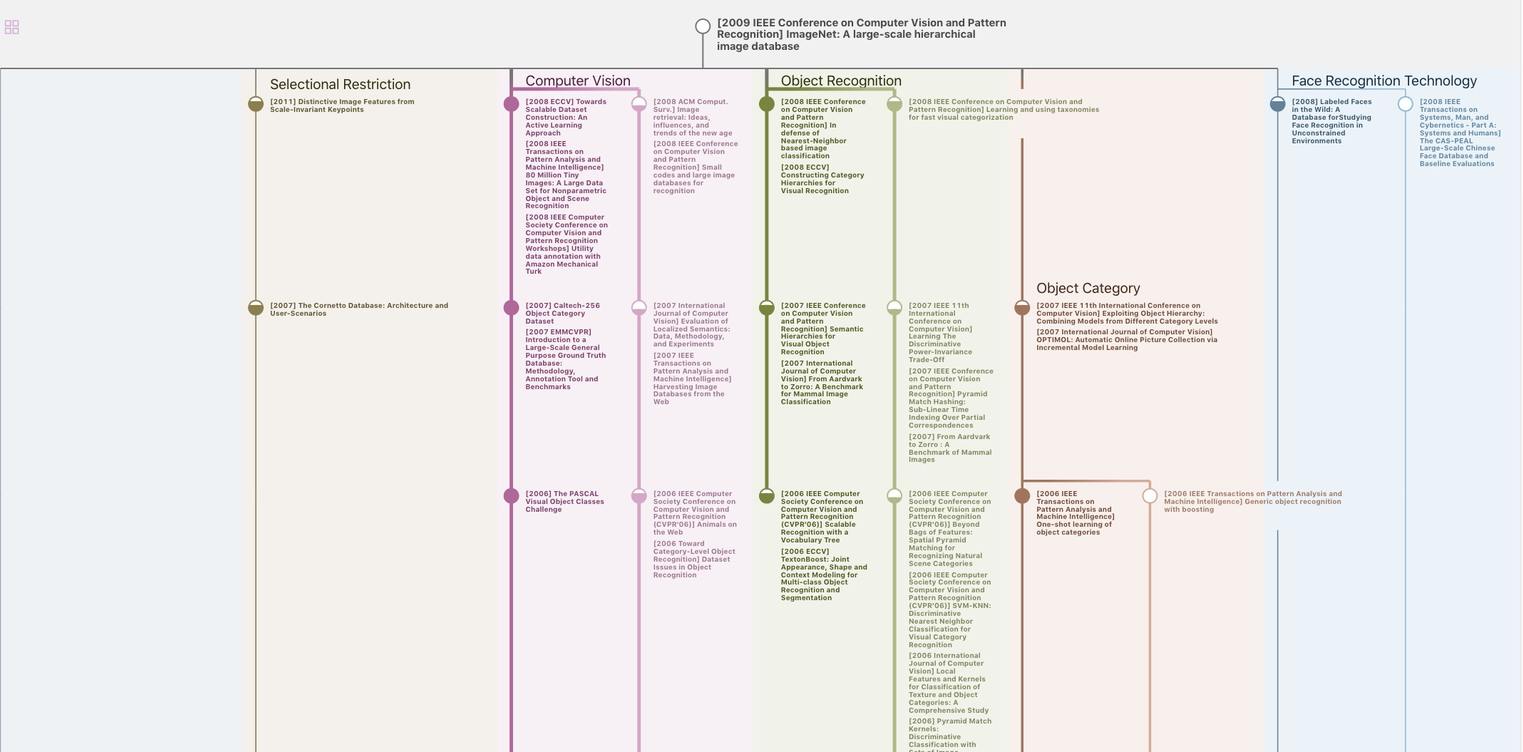
生成溯源树,研究论文发展脉络
Chat Paper
正在生成论文摘要