An efficient improved exponential distribution optimizer: application to the global, engineering and combinatorial optimization problems
Cluster Computing(2024)
摘要
Population-based meta-heuristic optimization algorithms play a vital role in addressing optimization problems. Nowadays, exponential distribution optimizer (EDO) can be considered to be one of the most recent among these algorithms. Although it has achieved many promising results, it has a set of shortcomings, for example, the decelerated convergence, and provides local optima solution as it cannot escape from local regions in addition to imbalance between diversification and intensification. Therefore, in this study, an enhanced variant of EDO called mEDO was proposed to address these shortcomings by combining two efficient search mechanisms named orthogonal learning (OL) and local escaping operator (LEO). In mEDO, the LEO has been exploited to escape local optima and improve the convergence behavior of the EDO by employing random operators to maximize the search process and to effectively discover the globally optima solution. Then the OL has been combined to keep the two phases (i.e., exploration and exploitation) balanced. To validate the effectiveness and performance of the mEDO algorithm, the proposed method has been evaluated over ten functions of the IEEE CEC’2020 test suite as well as eight real-world applications (engineering design optimization problems), Furthermore we test the applicability of the proposed algorithm by tackling 21 instance of the quadratic assignment problem (QAP). The experimental and statistical results of the proposed algorithm have been compared against seven other common metaheuristic algorithms (MAs), including the basic EDO. The results show the supremacy of the mEDO algorithm over the other algorithms and reveal the applicability and effectiveness of the mEDO algorithm compared to well-established metaheuristic algorithms. The experimental results and different statistical measures revealed the reliability and applicability of the mEDO method in solving the global, engineering design, and combinatorial optimization problems by achieving a reasonable solution in terms of scoring a global optima solutions and avoiding premature convergence by increasing the population’s diversity.
更多查看译文
关键词
Metaheuristic algorithms (MAs),Exponential distribution optimizer,Quadratic assignment problem (QAP),Local escaping operator (LEO),Orthogonal learning (OL),IEEE CEC’2020 test suit
AI 理解论文
溯源树
样例
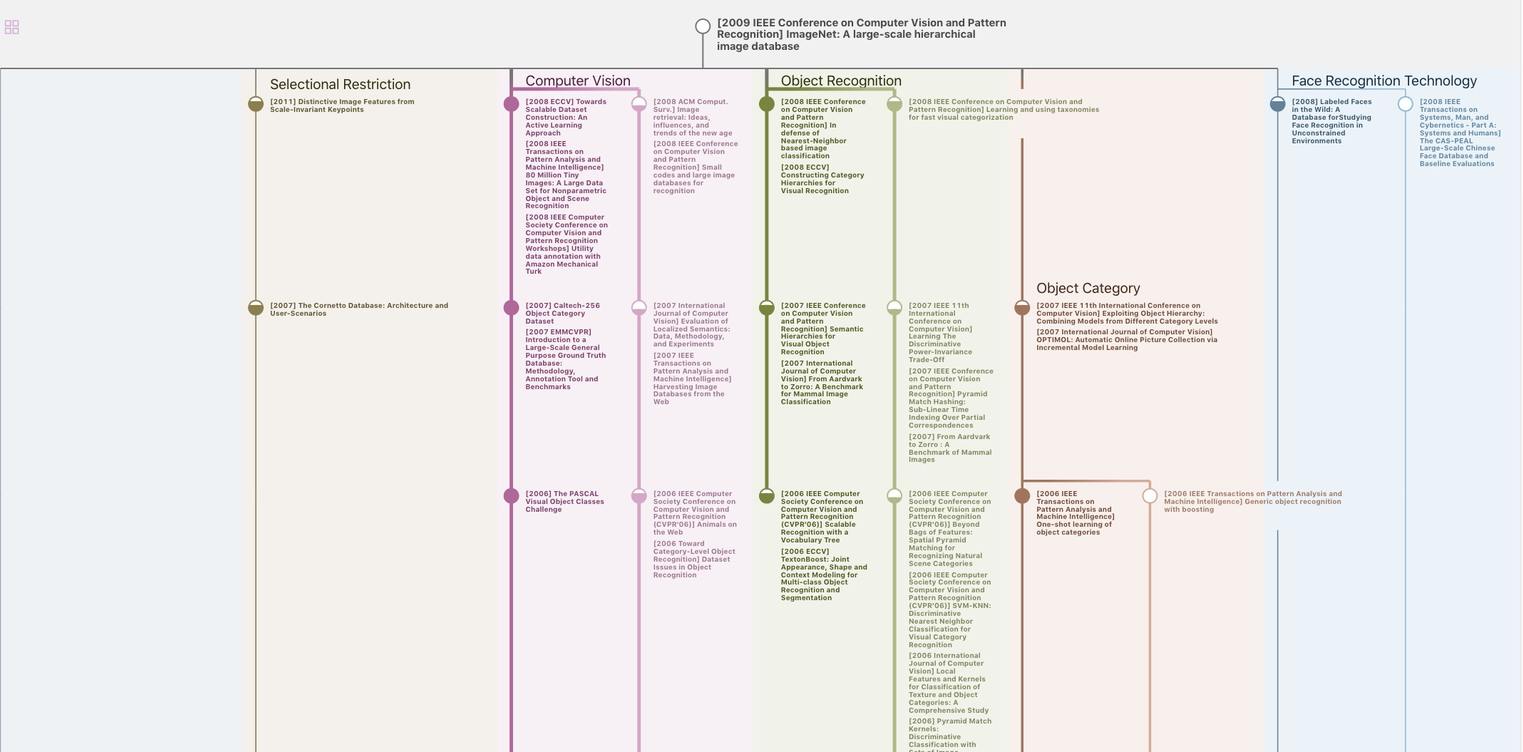
生成溯源树,研究论文发展脉络
Chat Paper
正在生成论文摘要