A communication-efficient distributed deep learning remote sensing image change detection framework
International Journal of Applied Earth Observation and Geoinformation(2024)
摘要
With the introduction of deep learning methods, the computation required for remote sensing change detection has significantly increased, and distributed computing is applied to remote sensing change detection to improve computational efficiency. However, due to the large size of deep learning models, the time-consuming gradient transfer during distributed model training weakens the acceleration effectiveness in change detection. Data communication and updates can be the bottlenecks in distributed change detection systems with limited network resources. To address the interrelated problems, we propose a communication-efficient distributed deep learning remote sensing change detection framework (CEDD-CD) based on the synchronous update architecture. The CEDD-CD integrates change detection with communication-efficient distributed gradient compression approaches, which can efficiently reduce the data volume to be transferred. In addition, for the implicit effect caused by the delay of compressed gradient update, a momentum compensation mechanism under theoretical analysis was constructed to reduce the time consumption required for model convergence and strengthen the stability of distributed training. We also designed a unified distributed change detection system architecture to reduce the complexity of distributed modeling. Experiments were conducted on three datasets; the qualitative and quantitative results demonstrate that the CEDD-CD was effective for massive remote sensing image change detection.
更多查看译文
关键词
Change detection,Distributed deep learning,Parallel computing,Communication compression,Staleness compensation
AI 理解论文
溯源树
样例
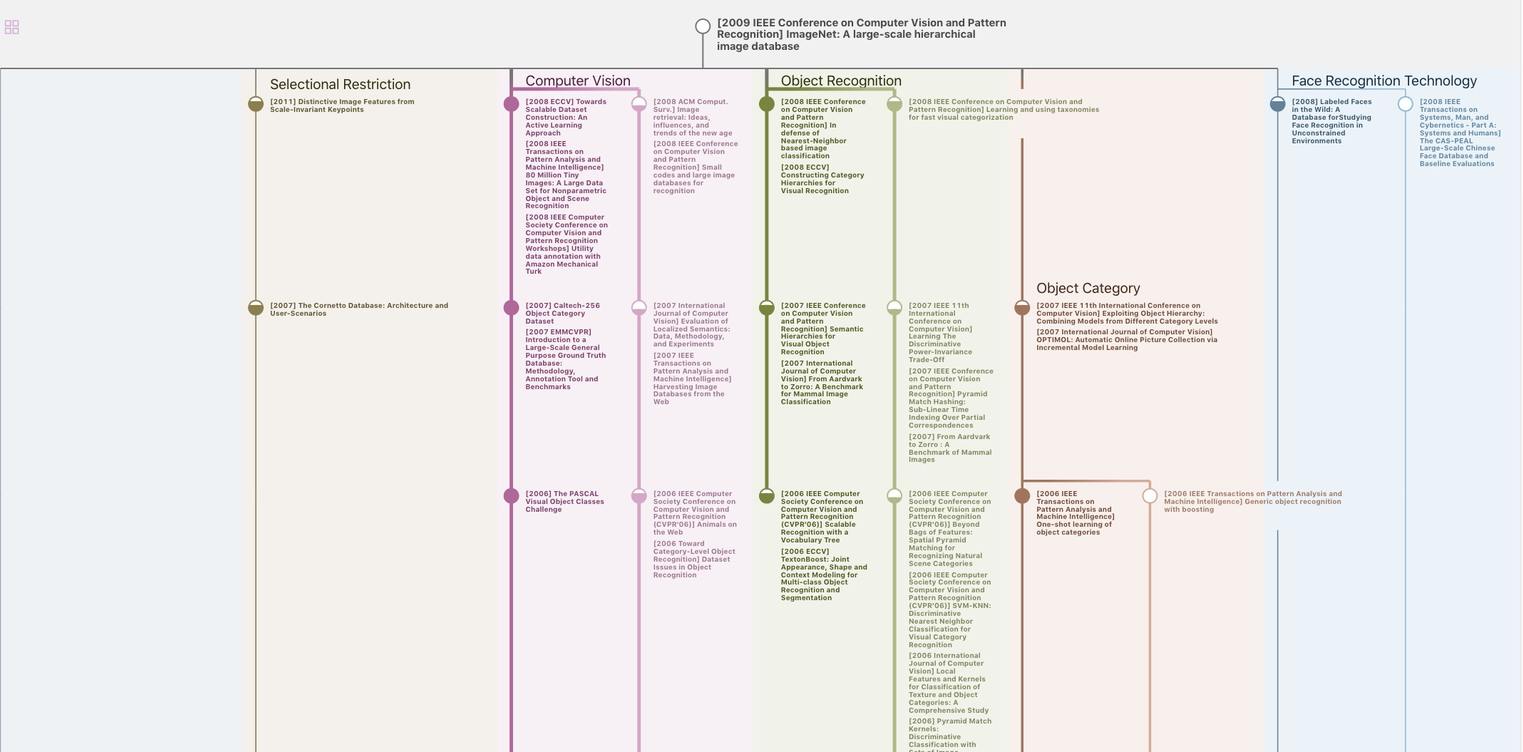
生成溯源树,研究论文发展脉络
Chat Paper
正在生成论文摘要