Machine learning developed a macrophage signature for predicting prognosis, immune infiltration and immunotherapy features in head and neck squamous cell carcinoma
crossref(2024)
摘要
Abstract Macrophages played an important role in the progression and treatment of cancer. Nevertheless, there is a limited amount of research that has comprehensively elucidated the characteristics of macrophages associated genes in head and neck squamous cell carcinoma (HNSCC). We employed weighted gene co-expression network analysis (WGCNA) to identify macrophage-related genes (MRGs) and classify patients with HNSCC into two distinct subtypes. A macrophage-related risk signature (MRS) model, comprising nine genes: IGF2BP2, PPP1R14C, SLC7A5, KRT9, RAC2, NTN4, CTLA4, APOC1, and CYP27A1, was formulated by integrating 101 machine learning algorithm combinations. We observed lower overall survival (OS) in the high-risk group and the high-risk group showed elevated expression levels in most of the differentially expressed immune checkpoint and human leukocyte antigen (HLA) genes, suggesting a strong immune evasion capacity in these tumors. Correspondingly, TIDE score positively correlated with risk score, implying that high-risk tumors may resist immunotherapy more effectively. At the single-cell level, we noted macrophages in the TME predominantly stalled in the G2/M phase, potentially hindering epithelial-mesenchymal transition and playing a crucial role in the inhibition of tumor progression. Additionally, we validated MRS gene expression levels using RT-qPCR and immunohistochemistry (IHC). The current study constructed a novel MRS for HNSCC, which could serve as an indicator for predicting the prognosis, immune infiltration and immunotherapy benefits for HNSCC patients.
更多查看译文
AI 理解论文
溯源树
样例
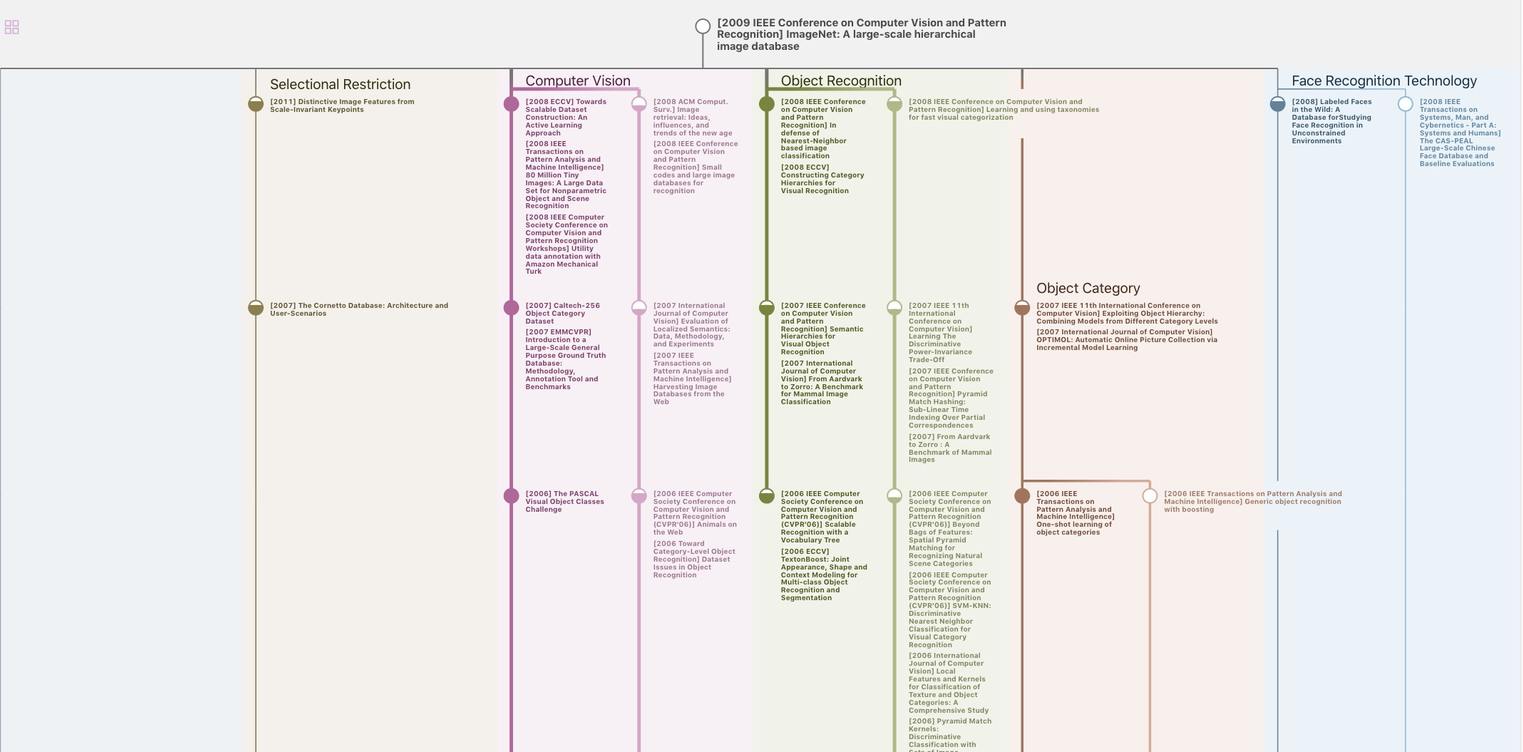
生成溯源树,研究论文发展脉络
Chat Paper
正在生成论文摘要