Nonlinear Slow Feature Analysis for Oscillating Characteristics Under Deep Encoder-Decoder Framework
IEEE Transactions on Industrial Informatics(2024)
摘要
Slow feature analysis aims to linearly transform measured data into uncorrelated signals that vary from slow to fast. While earlier extensions successfully extracted slow features from nonlinear sequential data, they lacked a modeling preference for nonstationary and oscillating features due to constraints on the prior distribution. To address this limitation, a semisupervised encoder-decoder architecture is proposed in this article, integrating a statistical preference for such characteristics. This regularization is achieved by introducing a first-order autoregressive Gaussian prior within a regular variational auto-encoder framework, as opposed to the standard Gaussian distribution. The evidence lower bound associated with the proposed model is derived using the variational Bayesian inference, and the model parameters are estimated iteratively. The effectiveness of the proposed approach is evaluated on both simulated and real industrial processes.
更多查看译文
关键词
Bayesian inference,nonstationarity,semisupervised learning,slow feature analysis,soft-sensing,variational autoencoder
AI 理解论文
溯源树
样例
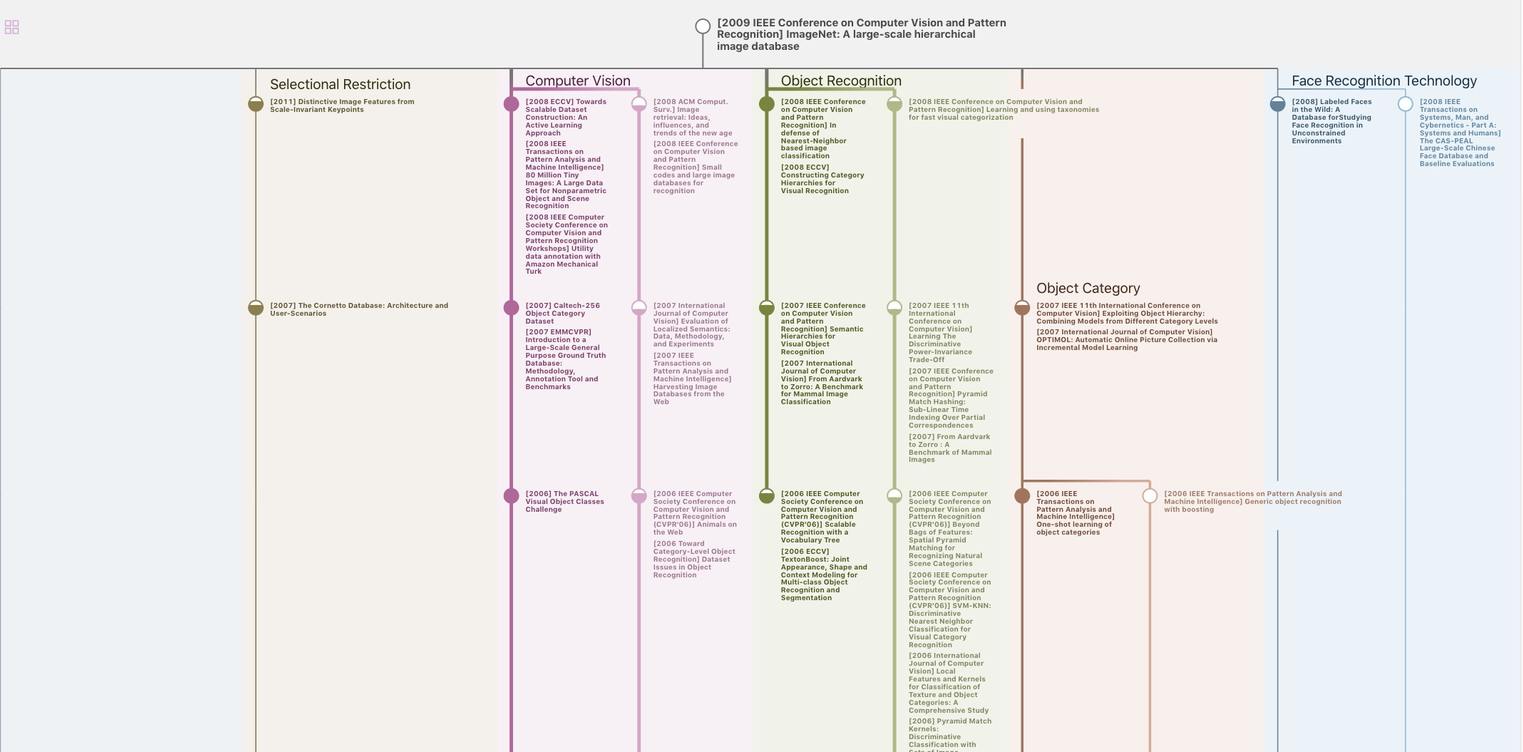
生成溯源树,研究论文发展脉络
Chat Paper
正在生成论文摘要