Affective Relevance
IEEE Intelligent Systems(2024)
摘要
Modeling information relevance aims to construct a conceptual understanding of information significant for users’ goals. Today, a myriad of relevance estimation methods are extensively used in various systems and services, mostly using behavioral signals such as dwell-time and clickthrough data and computational models of visual or textual correspondence to these behavioral signals. Consequently, these signals have become integral for personalizing social media, search engine results, recommender systems, and even supporting critical decision-making. However, behavioral signals are only capable of producing rough estimations of the actual underlying affective states that the users experience. Here, we provide an overview of recent alternative approaches for measuring and modeling more nuanced relevance based on physiological and neurophysiological sensing. Physiological and neurophysiological signals have the advantage of directly measuring users’ affective responses to information and provide rich data that are not accessible via behavioral measurements. With these data, it is possible to account for users’ affective experience and attentional correlates toward information.
更多查看译文
AI 理解论文
溯源树
样例
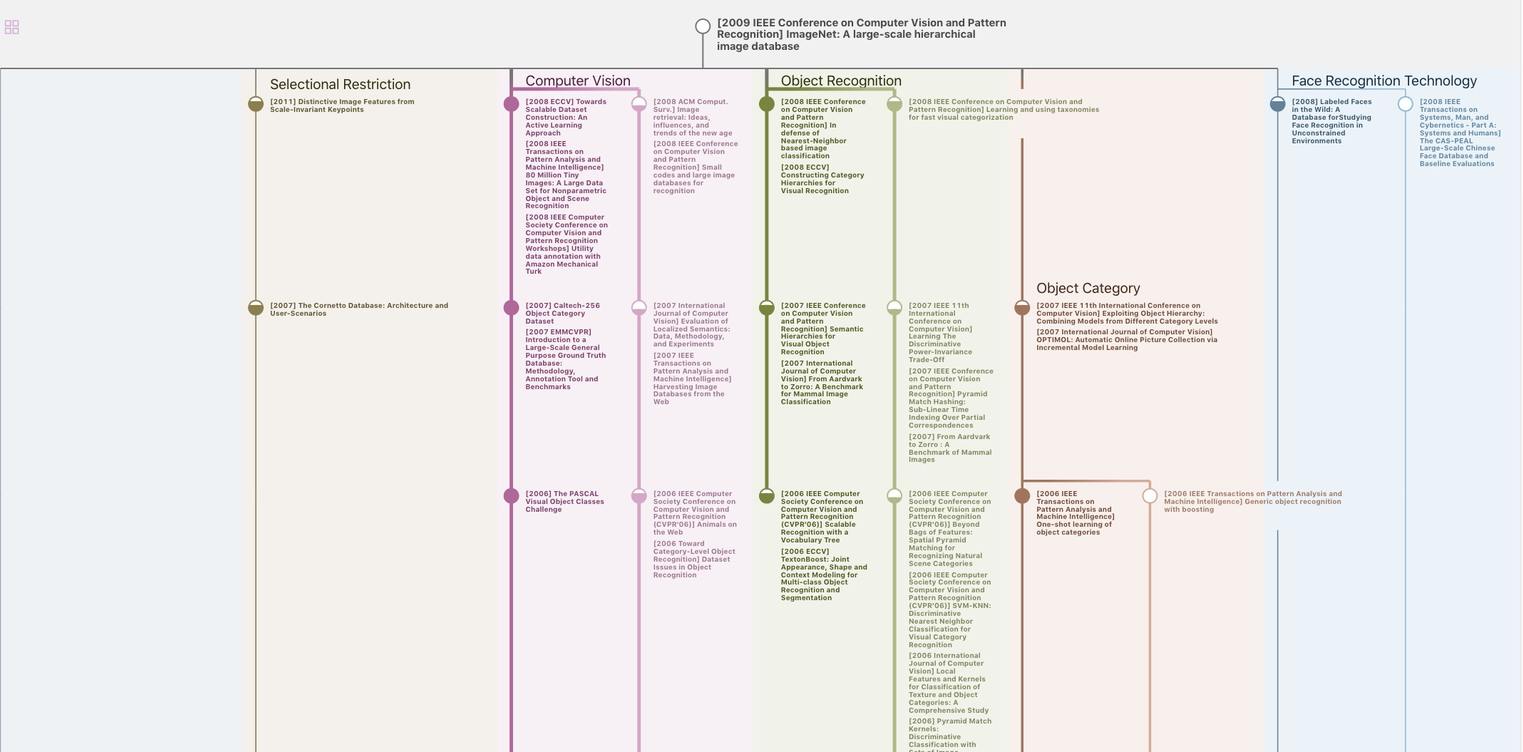
生成溯源树,研究论文发展脉络
Chat Paper
正在生成论文摘要