Visual Geometry Group Network for Flexible Printed Circuit Board Surface Defect Classification Réseau de groupes de géométrie visuelle pour la classification des défauts de surface des cartes de circuits imprimés flexibles
IEEE Canadian Journal of Electrical and Computer Engineering(2024)
摘要
Convolutional neural networks (CNNs) have drawn huge interest in the field of surface defect classification. During the production of flexible printed circuit boards (FPCBs), only a limited number of images of surface defects can be obtained. FPCB surface defect datasets have small samples and severe imbalances, which can significantly affect defect classification accuracy. Hence, this article presented a lightweight visual geometry group (L-VGG), developed by modifying the classical VGG16 network structure. The L-VGG network was optimized using L2 regularization and sample weighting, which alleviated the over-fitting phenomenon caused by small samples and improved validation accuracy. In addition, the differences among the classification accuracies of different defect images caused by imbalanced datasets were significantly reduced. The training time of the proposed L-VGG network was equivalent to 83.84% and 91.94% compression of the traditional VGG16 and ResNet18 networks, respectively. The dataset augmentation with generated images further mitigates the overfitting phenomenon caused by the small sample problem to some extent, and finally achieves a validation accuracy of 94.20%.
更多查看译文
关键词
Flexible printed circuit boards (FPCBs),regularization,sample weighting,surface defect classification,visual geometry group
AI 理解论文
溯源树
样例
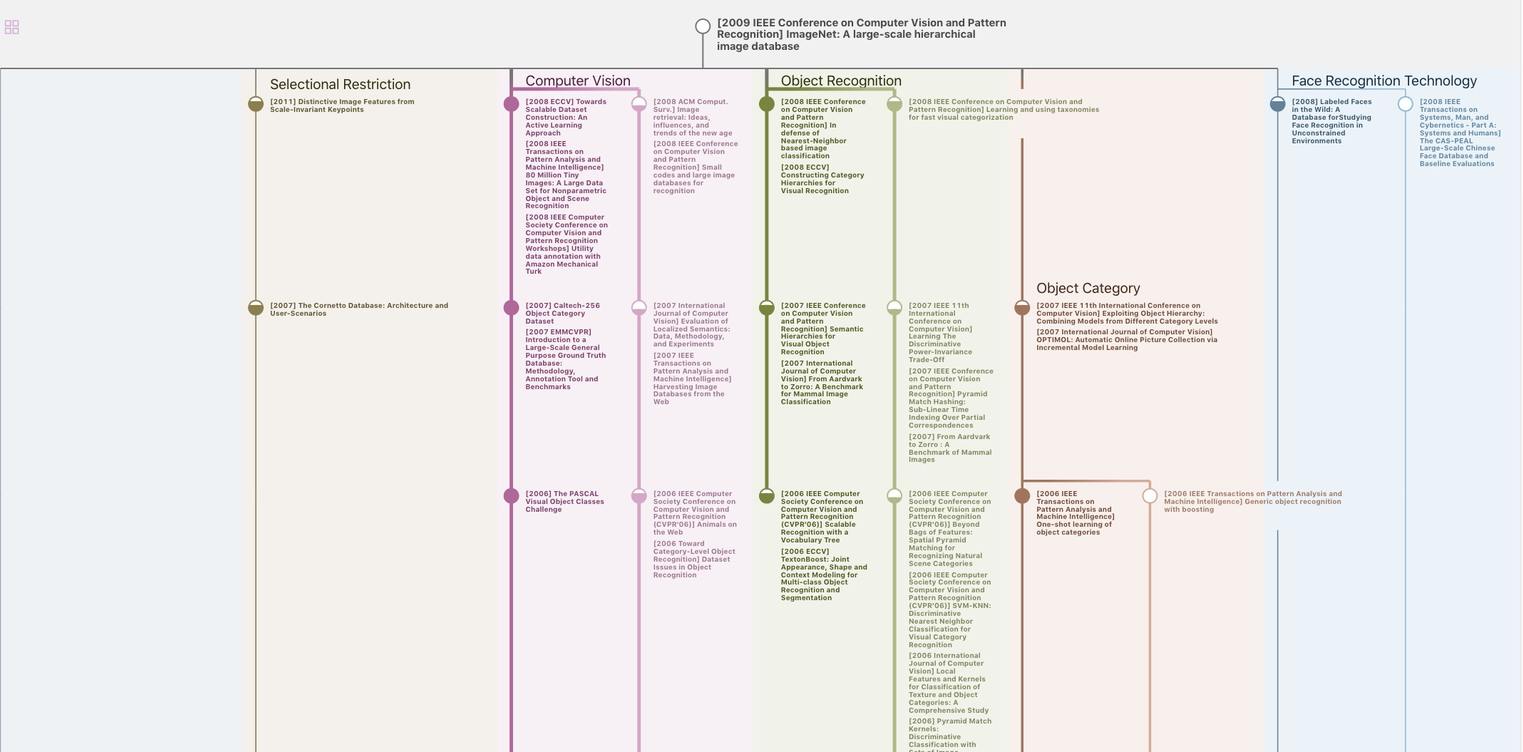
生成溯源树,研究论文发展脉络
Chat Paper
正在生成论文摘要