Multi-Agent Reinforcement Learning-Based Joint Caching and Routing in Heterogeneous Networks
IEEE Transactions on Cognitive Communications and Networking(2024)
摘要
In this paper, we explore the problem of minimizing transmission cost among cooperative nodes by jointly optimizing caching and routing in a hybrid network with vital support of service differentiation. We show that the optimal routing policy is a route-to-least cost-cache (RLC) policy for fixed caching policy. We formulate the cooperative caching problem as a multi-agent Markov decision process (MDP) with the goal of maximizing the long-term expected caching reward, which is NP-complete even when assuming users’ demand is perfectly known. To solve this problem, we propose C-MAAC, a partially decentralized multi-agent deep reinforcement learning (MADRL)-based collaborative caching algorithm employing actor-critic learning model. C-MAAC has a key characteristic of centralized training and decentralized execution, with which the challenge from unstable training process caused by simultaneous decision made by all agents can be addressed. Furthermore, we develop an optimization method as a criterion for our MADRL framework when assuming the content popularity is stationary and prior known. Our experimental results demonstrate that compared with the prior art, C-MAAC increases an average of 21.7% caching reward in dynamic environment when user request traffic changes rapidly.
更多查看译文
关键词
Caching,dynamic popularity distribution,deep reinforcement learning,multi-agent,routing
AI 理解论文
溯源树
样例
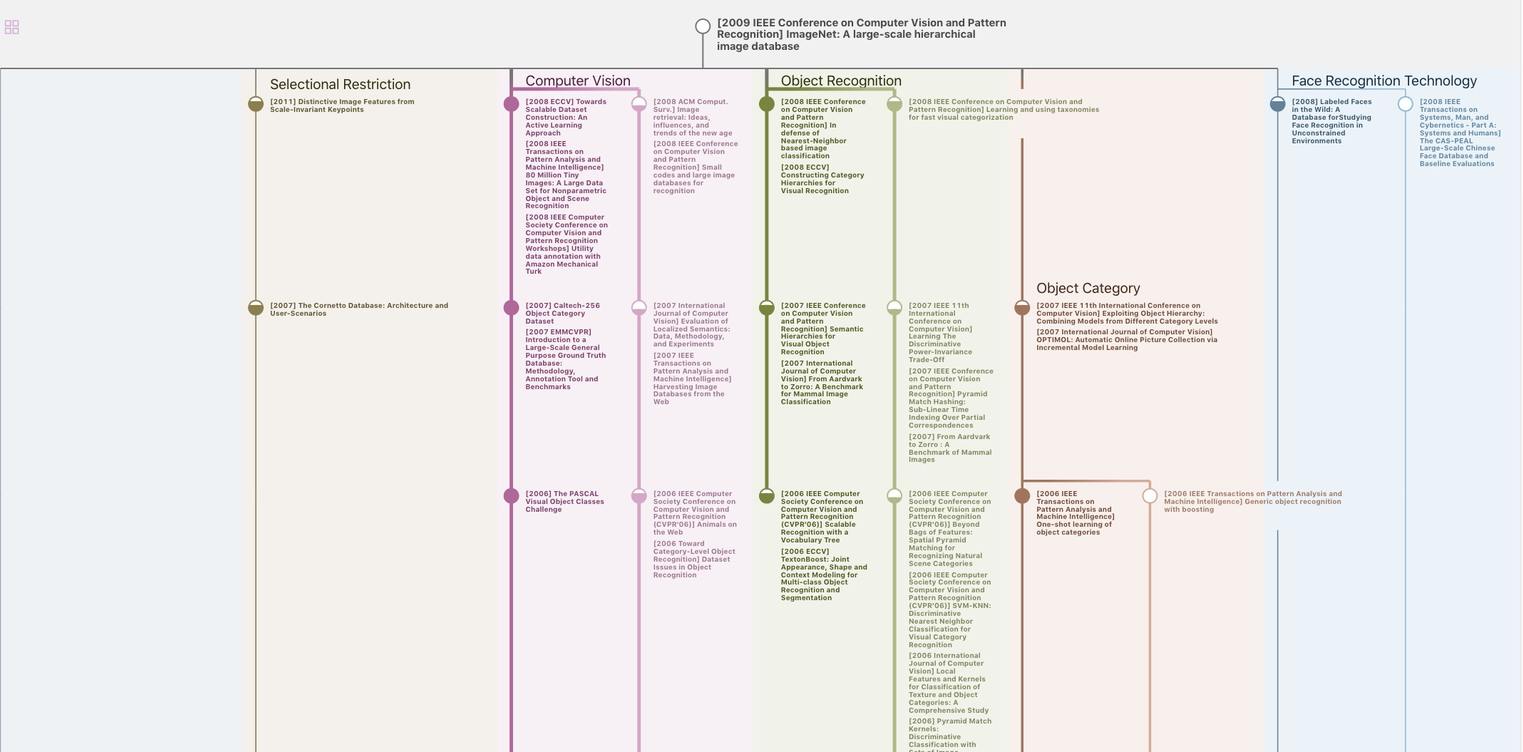
生成溯源树,研究论文发展脉络
Chat Paper
正在生成论文摘要