Statistical sampling of missing environmental variables improves biophysical genomic prediction in wheat
Theoretical and Applied Genetics(2024)
摘要
The integration of genomic prediction with crop growth models enabled the estimation of missing environmental variables which improved the prediction accuracy of grain yield. Since the invention of whole-genome prediction (WGP) more than two decades ago, breeding programmes have established extensive reference populations that are cultivated under diverse environmental conditions. The introduction of the CGM–WGP model, which integrates crop growth models (CGM) with WGP, has expanded the applications of WGP to the prediction of unphenotyped traits in untested environments, including future climates. However, CGMs require multiple seasonal environmental records, unlike WGP, which makes CGM–WGP less accurate when applied to historical reference populations that lack crucial environmental inputs. Here, we investigated the ability of CGM–WGP to approximate missing environmental variables to improve prediction accuracy. Two environmental variables in a wheat CGM, initial soil water content (InitlSoilWCont) and initial nitrate profile, were sampled from different normal distributions separately or jointly in each iteration within the CGM–WGP algorithm. Our results showed that sampling InitlSoilWCont alone gave the best results and improved the prediction accuracy of grain number by 0.07, yield by 0.06 and protein content by 0.03. When using the sampled InitlSoilWCont values as an input for the traditional CGM, the average narrow-sense heritability of the genotype-specific parameters (GSPs) improved by 0.05, with GNSlope, PreAnthRes, and VernSen showing the greatest improvements. Moreover, the root mean square of errors for grain number and yield was reduced by about 7
更多查看译文
AI 理解论文
溯源树
样例
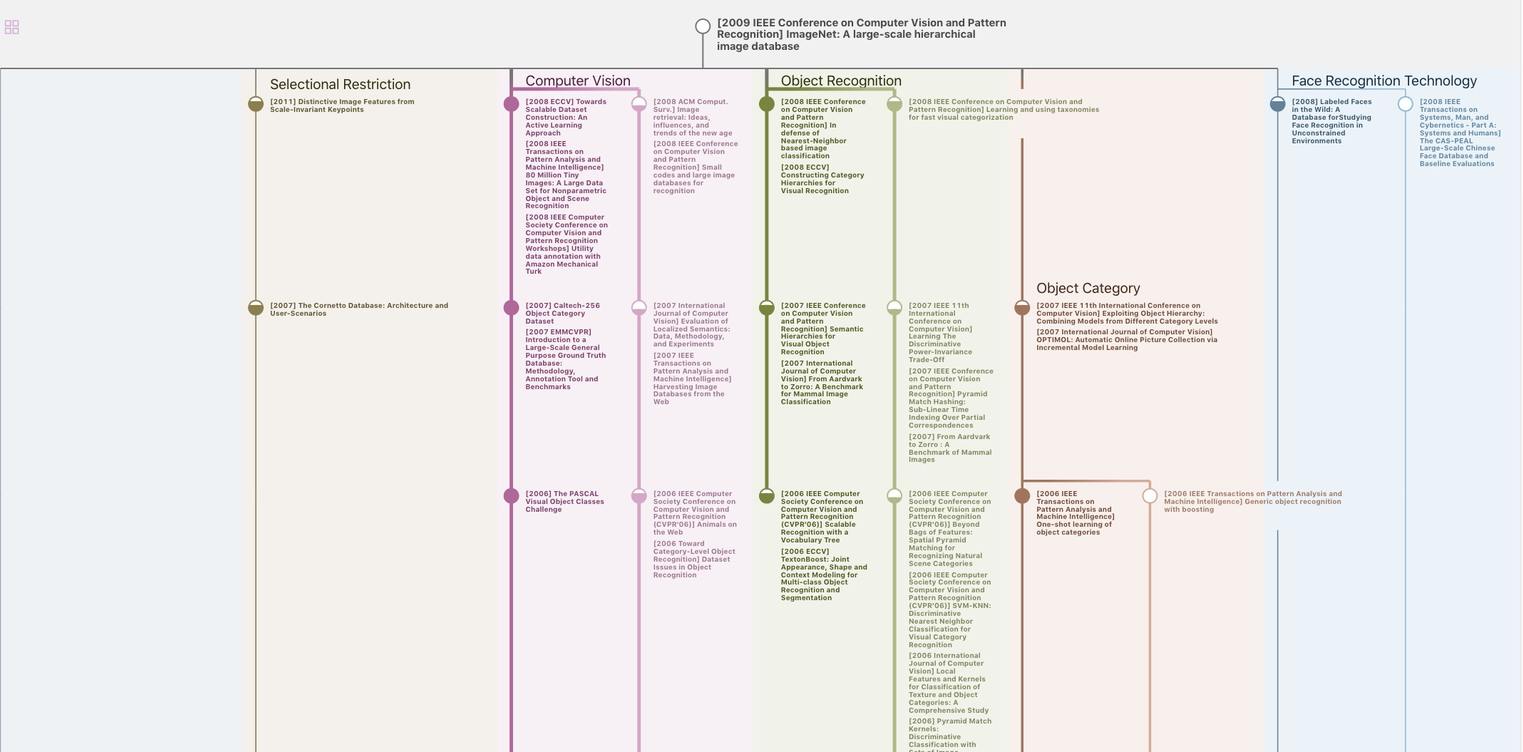
生成溯源树,研究论文发展脉络
Chat Paper
正在生成论文摘要