Data from Association between Receipt of Guideline-Concordant Lung Cancer Treatment and Individual- and Area-Level Factors: A Spatio-Temporal Analysis
crossref(2024)
Guideline-concordant treatment (GCT) of lung cancer has been observed to vary across geographic regions over the years. However, there is little evidence as to what extent this variation is explained by differences in patients’ clinical characteristics versus contextual factors, including socioeconomic inequalities.
Methods:This study evaluated the independent effects of individual- and area-level risk factors on geographic and temporal variation in receipt of GCT among patients with lung cancer. Receipt of GCT was defined on the basis of the National Comprehensive Cancer Network guidelines. We used Bayesian spatial-temporal multilevel models to combine individual and areal predictors and outcomes while accounting for geographically structured and unstructured correlation and linear and nonlinear trends.
Results:Our study included 4,854 non–small cell lung cancer (NSCLC) and small cell lung cancer (SCLC) cases, reported to the Victorian Lung Cancer Registry between 2011 and 2018. Area-level data comprised socioeconomic disadvantage and remoteness data at the local government area level in Victoria, Australia. Around 60.36% of patients received GCT, and the rates varied across geographic areas over time. This variation was mainly associated with poor performance status, advanced clinical stages, NSCLC types, public hospital insurance, area-level deprivation, and comorbidities.
Conclusions:This study highlights the need to address disparities in receipt of GCT among patients with lung cancer with poor performance status, NSCLC, advanced clinical stage, stage I–III SCLC, stage III NSCLC, public hospital insurance, and comorbidities, and living in socioeconomically disadvantaged areas.
Impact:Two-year mortality outcomes significantly improved with GCT. Interventions aimed at reducing these inequalities could help to improve lung cancer outcomes.
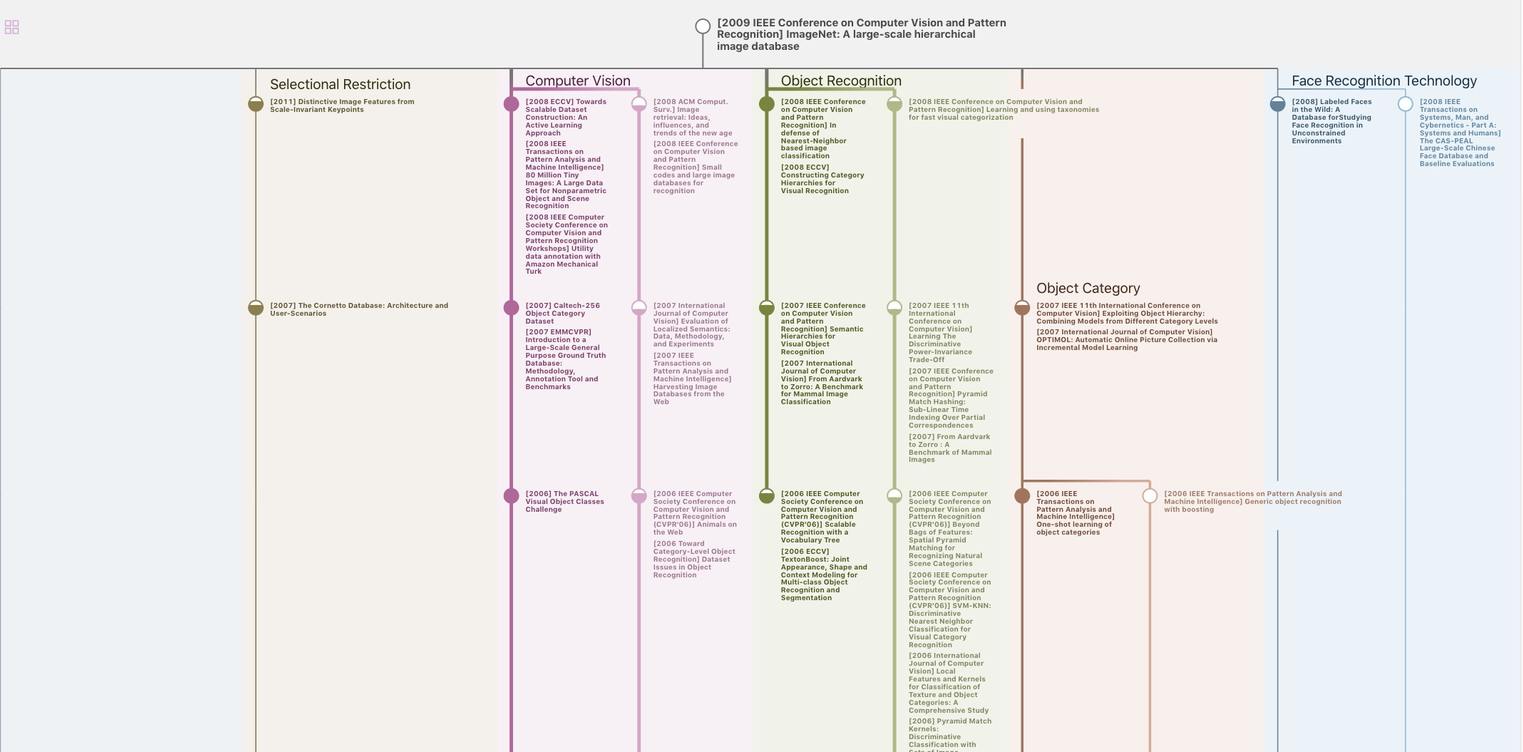