An Effective EEG-Based Image Classification Methodology Under Low Data and Resource Requirements Using Machine Learning
2024 International Conference on Computer, Electrical & Communication Engineering (ICCECE)(2024)
摘要
Deep Learning (DL) methods such as Convolutional Neural Network (CNN) learning approaches are used to extract features from images through convolution operation. These features are widely used for image classification, object detection or segmentations tasks. But a high testing accuracy needs lot of input images for training, whereas a human brain can do similar tasks with a few training samples. Present study is aiming to establish the potential of extracted features from electroencephalogram (EEG) signals acquired from brain superior to CNN extracted features for image classification tasks. For this, EEG signals are collected while seeing an image and thinking about its attributes through a questionnaire. A five-channel EEG headset has been used for data collection. Four standard statistical features, namely mean, standard deviation, kurtosis and skewness have been extracted from signals emitted from each electrode. A total of twenty features extracted from five-channel EEG data have been used as input in training against the output labels through four different machine learning (ML) classifiers such as random forest (RF), support vector machine (SVM), multi-layer perceptron (MLP), extreme gradient boosting algorithm (XGBoost). Same images have been used for training, and inferenced through a CNN based binary classifier. Results show a lower performance metrics with CNN than EEG feature-based classifiers. Such concept can overcome the high data related constraint in areas like manufacturing.
更多查看译文
关键词
EEG,CNN,Machine learning,Deep learning,Image classification,Human brain
AI 理解论文
溯源树
样例
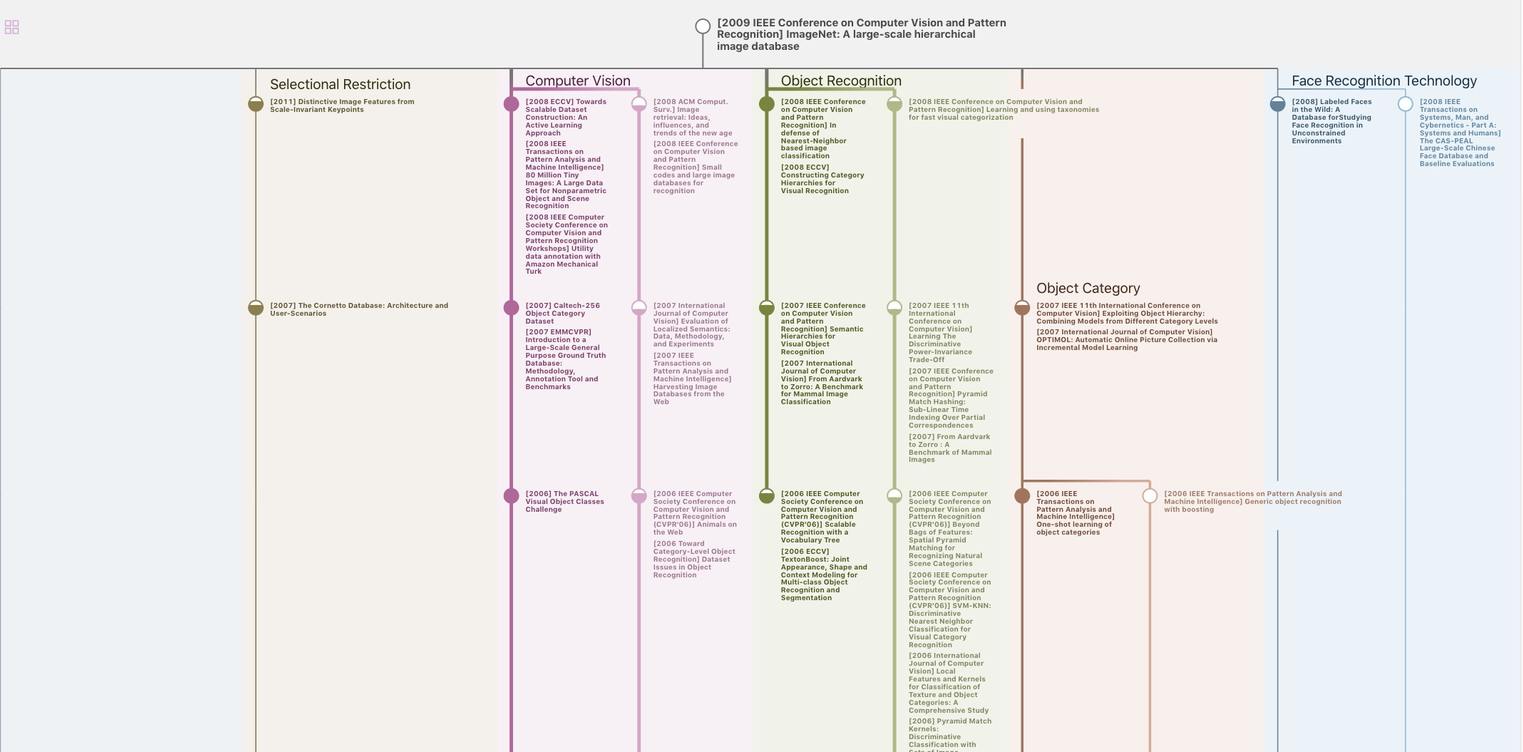
生成溯源树,研究论文发展脉络
Chat Paper
正在生成论文摘要