Causal Effect Estimation on Imaging and Clinical Data for Treatment Decision Support of Aneurysmal Subarachnoid Hemorrhage.
IEEE transactions on medical imaging(2024)
摘要
Aneurysmal subarachnoid hemorrhage is a serious medical emergency of brain that has high mortality and poor prognosis. Treatment effect estimation is of high clinical significance to support the treatment decision-making for aneurysmal subarachnoid hemorrhage. However, most existing studies on treatment decision support of this disease are unable to simultaneously compare the potential outcomes of different treatments for a patient. Furthermore, these studies fail to harmoniously integrate the imaging data with non-imaging clinical data, both of which are significant in clinical scenarios. In this paper, the key challenges we address are: how to effectively estimate the treatment effect for aneurysmal subarachnoid hemorrhage; and how to utilize multi-modality data to perform this estimation. Specifically, we first propose a novel scheme that uses multi-modality confounders distillation architecture to predict the treatment outcome and treatment assignment simultaneously. Notably, with these distilled confounder features, we design an imaging and non-imaging interaction representation learning strategy to use the complementary information extracted from different modalities to balance the feature distribution of different treatment groups. We have conducted extensive experiments using a clinical dataset of 656 subarachnoid hemorrhage cases, which was collected from the Hospital Authority of Hong Kong. Our method shows consistent improvements on the evaluation metrics of treatment effect estimation, achieving state-of-the-art results over strong competitors. Code is released at https://github.com/med-air/TOP-aSAH.
更多查看译文
关键词
Imaging and non-imaging data,treatment effect estimation,aneurysmal subarachnoid hemorrhage
AI 理解论文
溯源树
样例
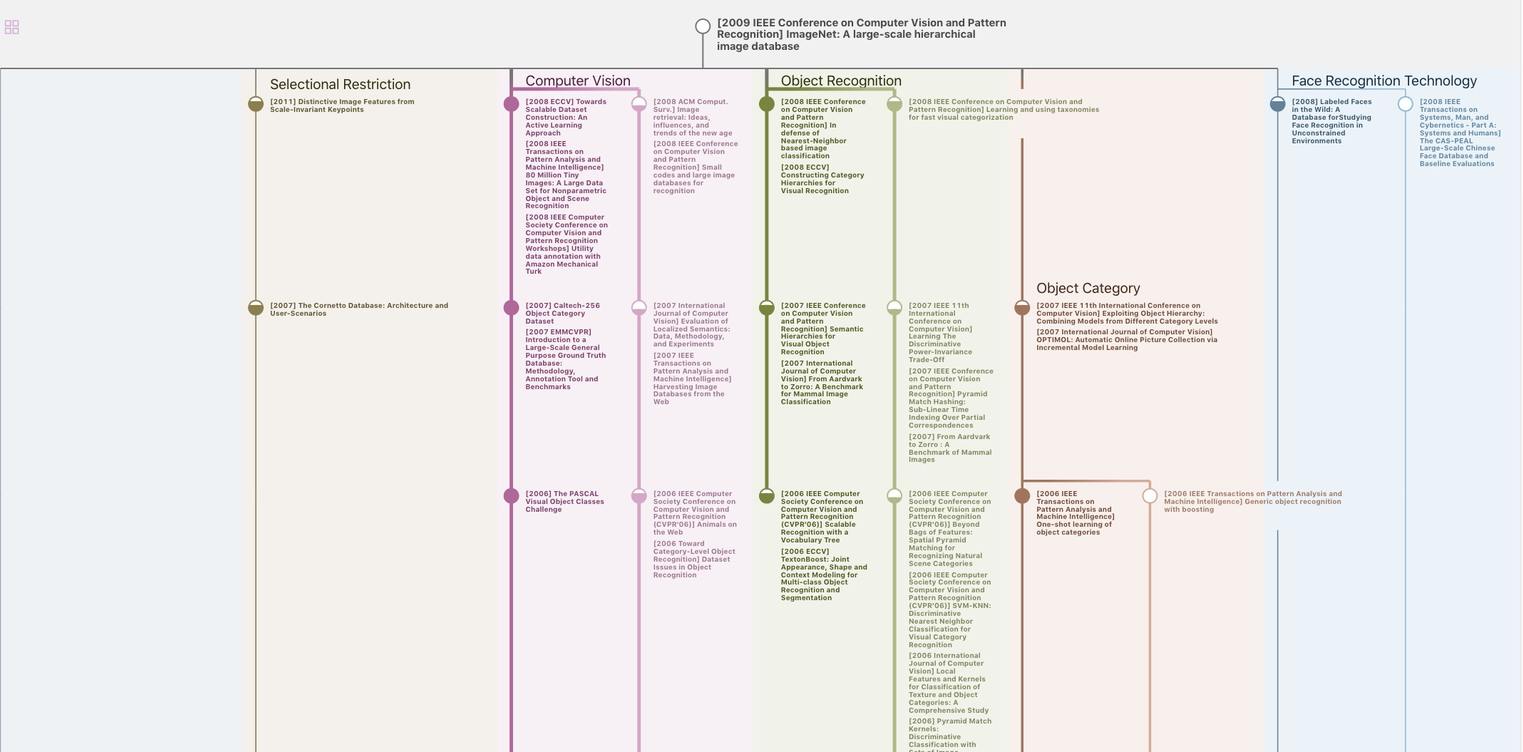
生成溯源树,研究论文发展脉络
Chat Paper
正在生成论文摘要