Rebalancing network with knowledge stability for class incremental learning
Pattern Recognition(2024)
摘要
Class incremental learning (CIL) has been proposed to solve the problem of learning to classify new classes while maintaining the performance on old classes. A typical strategy is to update the old classification model with entire new class data and a few old exemplars, which face serious performance degradation on old classes. The main reasons come down to class imbalance between old and new classes along with catastrophic forgetting towards old classes. Most existing CIL methods have proposed solving the above two issues in classification space, ignoring their adverse effects of overlapping between old and new classes and confusion among old classes in feature space. In this paper, we propose a rebalancing network with knowledge stability (RNKS), aiming to adequately retain the model performance on old classes in CIL by solving class imbalance and catastrophic forgetting in feature and classification space simultaneously. In detail, the proposed RNKS mainly consists of multi-proxies rebalancing (MPR) and hybrid knowledge distillation (HKD). MPR, focusing on class imbalance, employs multi-proxies metric learning to decrease the feature overlapping between old and new classes, together with balanced data sampling to correct the skewed decision boundary. HKD, coping with catastrophic forgetting, encourages the updated model to reproduce identical feature topologies and predictions of old classes as the old model via feature relation-based and response-based distillations. Experiments on CIFAR-100 and ILSVRC datasets demonstrate the effectiveness of this work against the state-of-the-art approaches.
更多查看译文
关键词
Class incremental learning,Catastrophic forgetting,Class imbalance,Proxy-based metric learning,Knowledge distillation
AI 理解论文
溯源树
样例
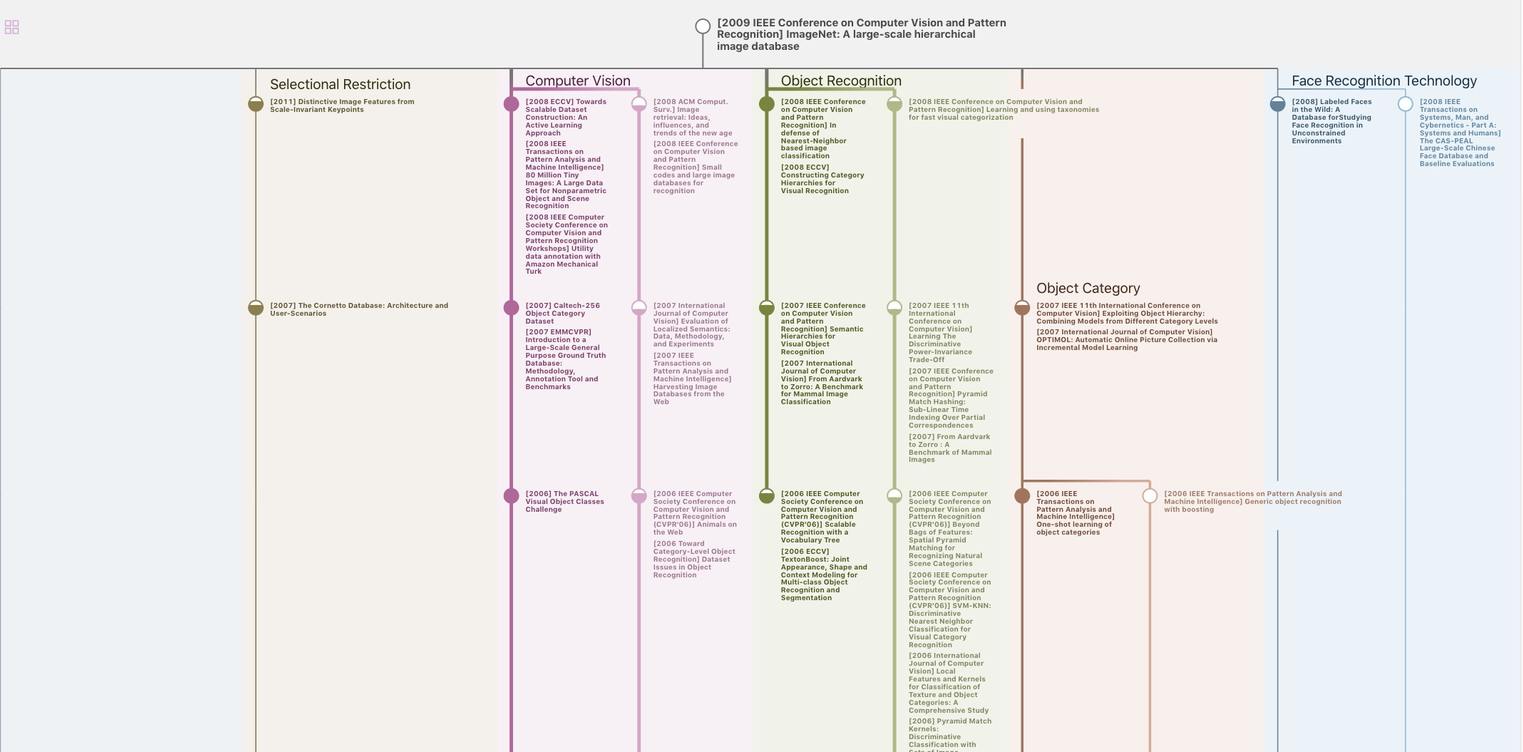
生成溯源树,研究论文发展脉络
Chat Paper
正在生成论文摘要