Mirrored X-Net: Joint Classification and Contrastive Learning for Weakly Supervised GA Segmentation in SD-OCT★
Pattern Recognition(2024)
摘要
Deep learning achieves impressive performance in medical image segmentation, but the training phase requires a large amount of annotated data with precise clinical definitions. Weakly supervised lesion segmentation aims to produce pixel-level masks by learning discriminatory information from weak annotations. In this paper, Mirrored X-Net, a novel segmentation model only supervised by image-level category labels, is proposed to segment the Geographic Atrophy (GA) regions in en-face projection of Spectral-Domain Optical Coherence Tomography (SD-OCT) data. Characterized by the dimension-asymmetric information in SD-OCT images, a novel Anisotropic Downsampling (ADS) is proposed to augment feature shapes. To extract the regions of normal retina for both images with and without lesions, we propose a contrastive learning module to assimilate the deep representation of normal tissue in the SD-OCT images and improve the class-wise difference of deep representation. Based on the contrastive learning module, Anomalous Probability Map (APM) can be obtained to draw the distribution of difference with normal tissue in images. We jointly train the image classification and contrastive learning module, and the final GA segmentation is refined based on the en-face projection of APM. The experimental results on two independent GA datasets demonstrate that the proposed weakly supervised model can produce satisfactory results, and obtain even higher accuracy than fully supervised approaches. The source code is available at https://github.com/maxiao0234/Mirrored-X-Net-pytorch.
更多查看译文
关键词
Age-related macular degeneration,Geographic atrophy segmentation,SD-OCT images,Weakly supervised learning,Contrastive learning,Multitask learning
AI 理解论文
溯源树
样例
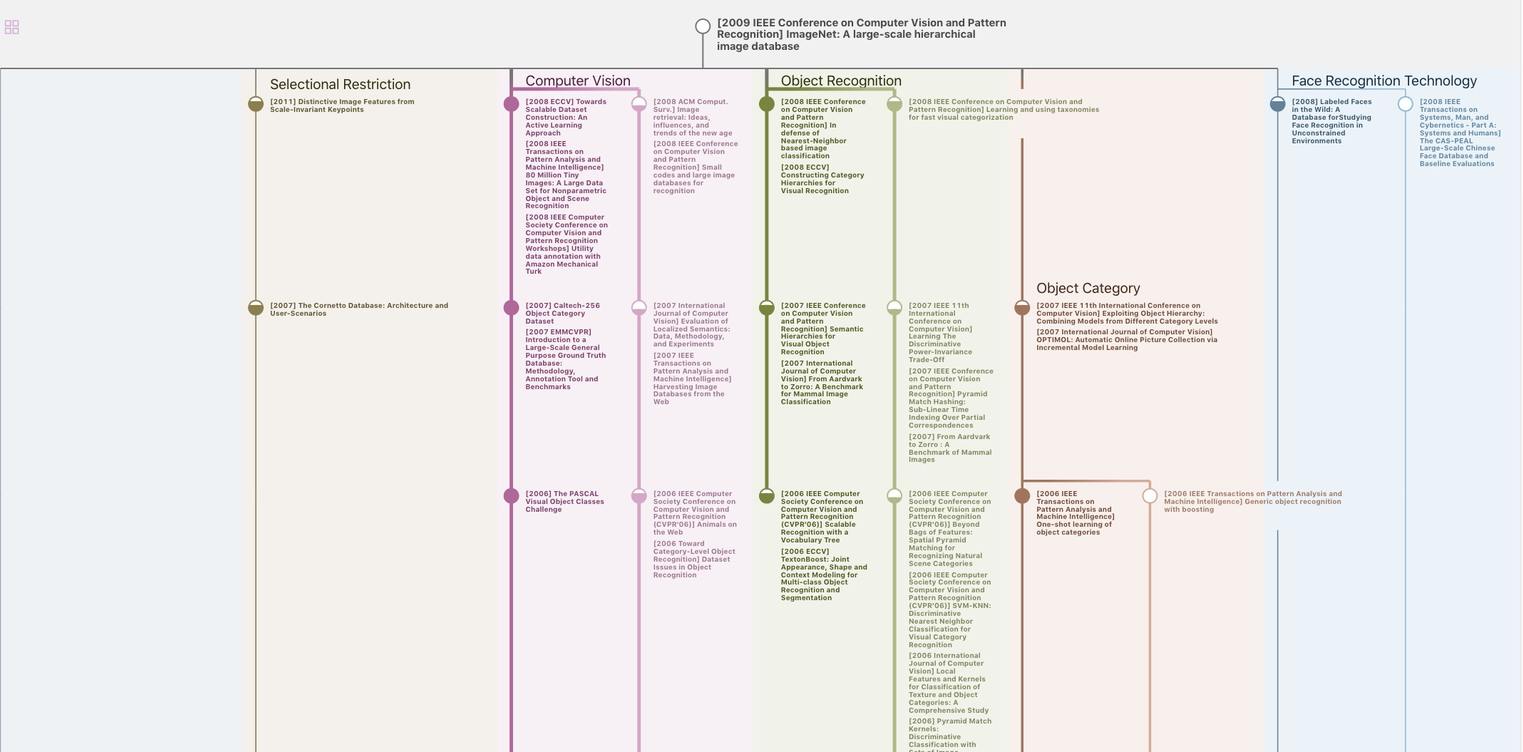
生成溯源树,研究论文发展脉络
Chat Paper
正在生成论文摘要