Matrix Wasserstein distance generative adversarial network with gradient penalty for fast low-carbon economic dispatch of novel power systems
Energy(2024)
摘要
For novel power systems, access to sustainable energy sources will undoubtedly increase the uncertainty of power systems significantly. Consequently, to guarantee efficient, secure, smooth, and expedited functioning, this work proposes a matrix Wasserstein distance generative adversarial network with gradient penalty (MWGAN-GP), which considers the minimization of generation cost and carbon emission simultaneously for low-carbon economic dispatch (ED) of novel power systems. The proposed MWGAN-GP generates a series of matrix data by a trained generator, equalizes load values and the sum of unit outputs at each period by relaxation operation, and rapidly generates numerous ED solutions. The speed of ED based on MWGAN-GP is greatly faster than the speed of ED based on a traditional genetic algorithm (GA). Furthermore, the introduction of the relaxation operation decreases the average cost of ED based on MWGAN-GP by 7.94% compared to GA according to the case with 4000 sets of load data.
更多查看译文
关键词
Economic dispatch,generative adversarial networks,deep learning,relaxation operation
AI 理解论文
溯源树
样例
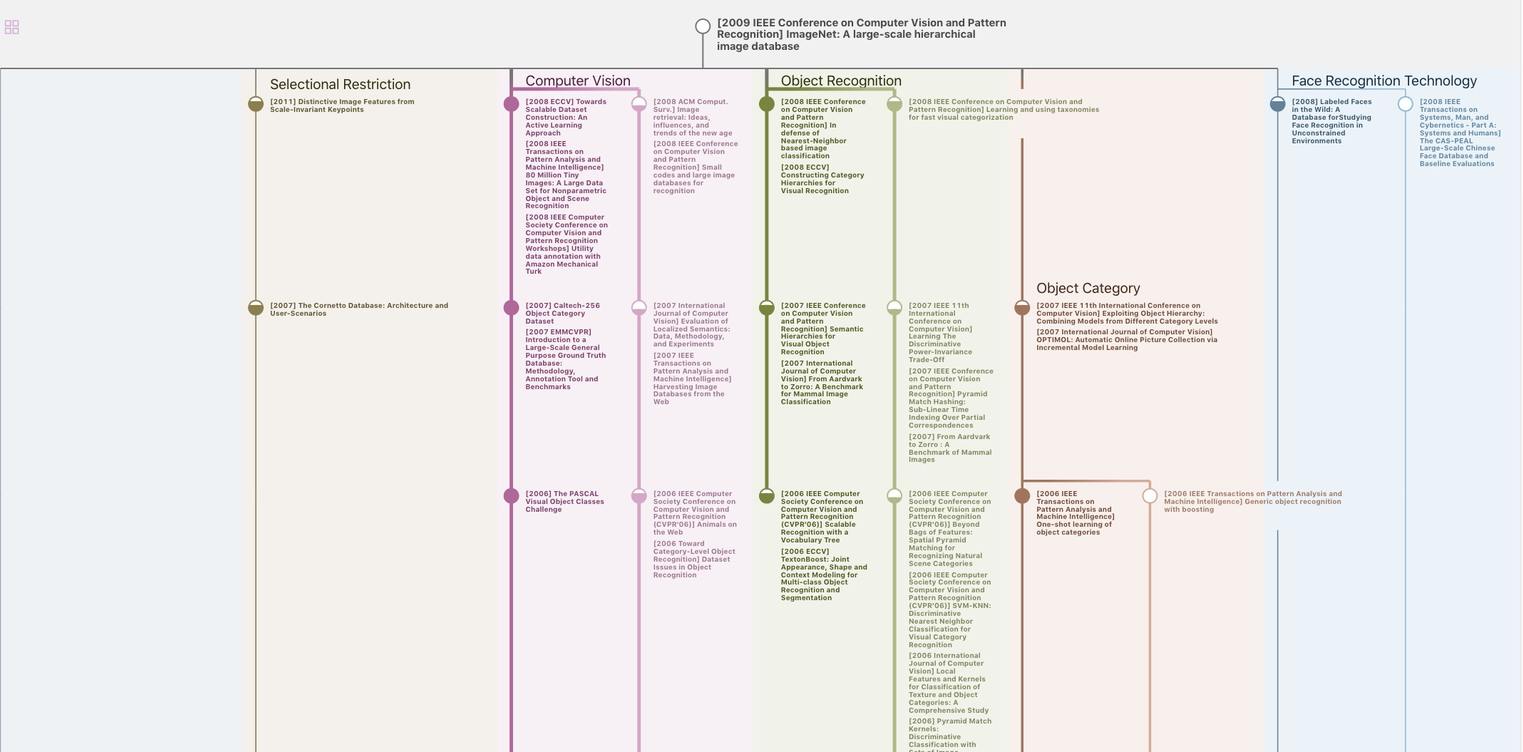
生成溯源树,研究论文发展脉络
Chat Paper
正在生成论文摘要