Quantized Zeroth-Order Gradient Tracking Algorithm for Distributed Nonconvex Optimization Under Polyak- [Formula: see text] ojasiewicz Condition.
IEEE transactions on cybernetics(2024)
摘要
This article focuses on distributed nonconvex optimization by exchanging information between agents to minimize the average of local nonconvex cost functions. The communication channel between agents is normally constrained by limited bandwidth, and the gradient information is typically unavailable. To overcome these limitations, we propose a quantized distributed zeroth-order algorithm, which integrates the deterministic gradient estimator, the standard uniform quantizer, and the distributed gradient tracking algorithm. We establish linear convergence to a global optimal point for the proposed algorithm by assuming Polyak- [Formula: see text] ojasiewicz condition for the global cost function and smoothness condition for the local cost functions. Moreover, the proposed algorithm maintains linear convergence at low-data rates with a proper selection of algorithm parameters. Numerical simulations validate the theoretical results.
更多查看译文
AI 理解论文
溯源树
样例
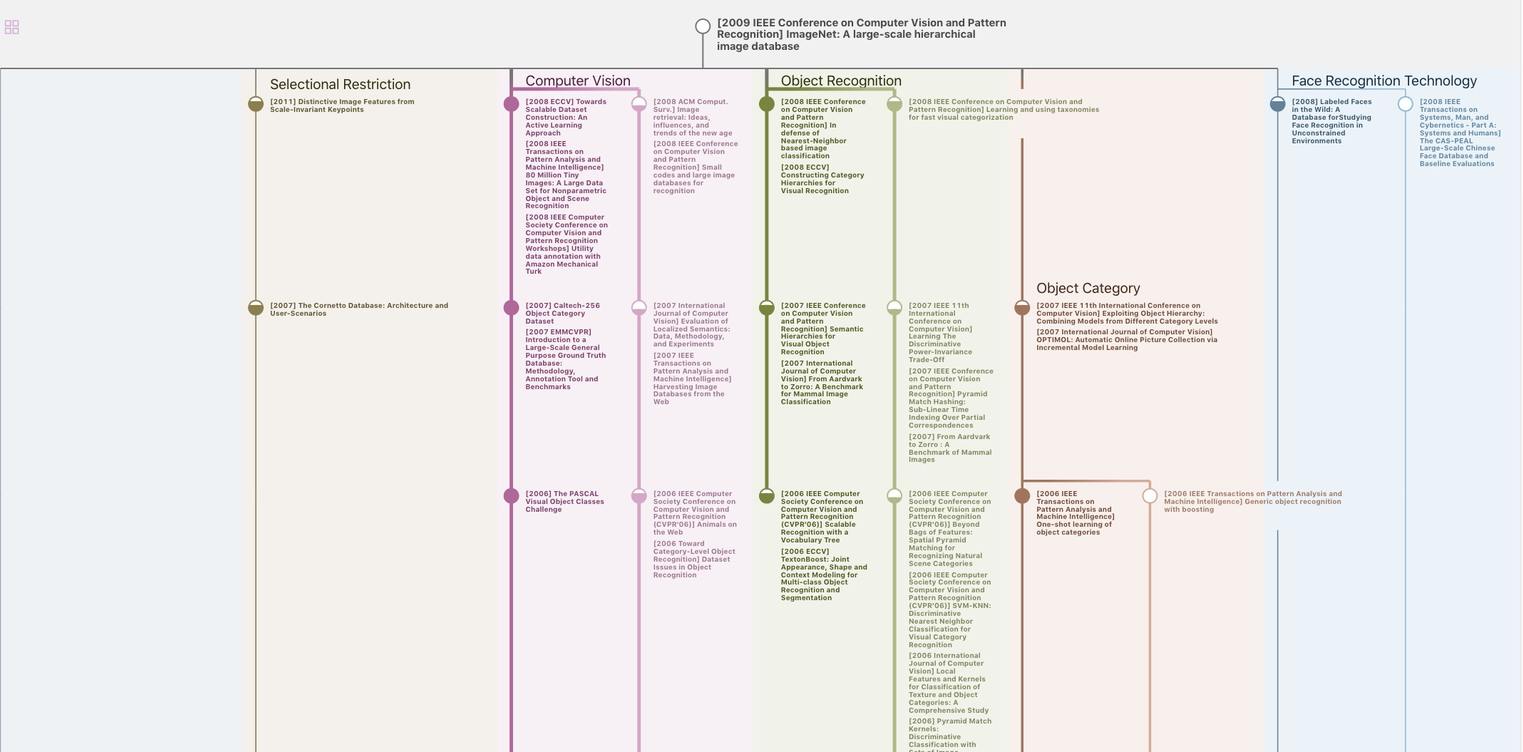
生成溯源树,研究论文发展脉络
Chat Paper
正在生成论文摘要