Methods for neural network based prediction of roughness-induced crossflow-like vorticity in re-entry scenarios
International Journal of Heat and Fluid Flow(2024)
摘要
Laminar-turbulent transition plays a critical role in the design of re-entry vehicles. This study investigates roughness-induced transition caused by a randomly distributed roughness patch. In the wake of the roughness patch, a cross-flow-like vortex is formed by the distributed roughness. The cross-flow-like vortex generates regions of strong wall-normal and spanwise gradients in the streamwise velocity where unstable acoustic modes are strongly amplified leading to transition. This study is using a database of Direct Numerical Simulations (DNS) to predict the magnitude of the streamwise vorticity by the roughness patch with the usage of two machine-learning methods: A feed-forwarding Deep Neural Network (DNN) and a Convolutional Neural Network (CNN). The study shows how the training and validation database size influences the prediction performance of the DNN. Further, a parameter study revealed the most influential input parameters for the DNN linking the shape of the roughness with the strength of the vortex. The CNN shows a better prediction performance compared to the DNN. The area relevant to the CNN prediction is displayed with a saliency map and is overlapping with the area of the vortex origin. A patch was selected with the machine-learning algorithms and the generation of the cross-flow-like vortex and its effect on the stability of the flow was investigated.
更多查看译文
关键词
Machine learning,Laminar-turbulent transition,High-enthalpy boundary-layer flows,Saliency maps,Distributed roughness
AI 理解论文
溯源树
样例
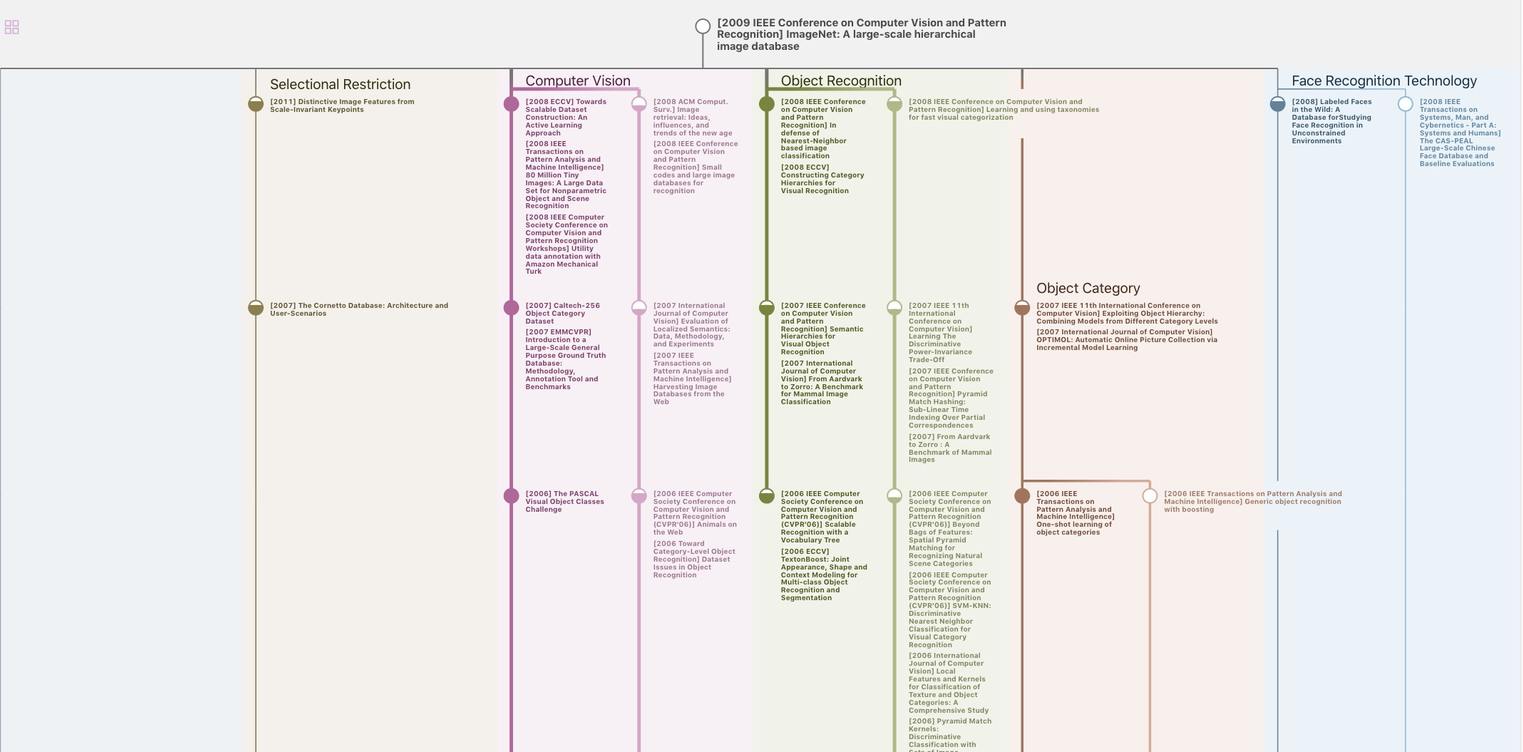
生成溯源树,研究论文发展脉络
Chat Paper
正在生成论文摘要