On enhanced kkt optimality conditions for smooth nonlinear optimization
SIAM JOURNAL ON OPTIMIZATION(2024)
摘要
The Fritz John (FJ) and Karush-Kuhn-Tucker (KKT) conditions are fundamental tools for characterizing minimizers and form the basis of almost all methods for constrained optimization. Since the seminal works of Fritz John, Karush, Kuhn, and Tucker, FJ/KKT conditions have been enhanced by adding extra necessary conditions. Such an extension was initially proposed by Hestenes in the 1970s and later extensively studied by Bertsekas and collaborators. In this work, we revisit enhanced KKT stationarity for standard (smooth) nonlinear programming. We argue that every KKT point satisfies the usual enhanced versions found in the literature. Therefore, enhanced KKT stationarity only concerns the Lagrange multipliers. We then analyze some properties of the corresponding multipliers under the quasi -normality constraint qualification (QNCQ), showing in particular that the set of so-called quasinormal multipliers is compact under QNCQ. Also, we report some consequences of introducing an extra abstract constraint to the problem. Given that enhanced FJ/KKT concepts are obtained by aggregating sequential conditions to FJ/KKT, we discuss the relevance of our findings with respect to the well-known sequential optimality conditions, which have been crucial in generalizing the global convergence of a well -established safeguarded augmented Lagrangian method. Finally, we apply our theory to mathematical programs with complementarity constraints and multiobjective problems, improving and elucidating previous results in the literature.
更多查看译文
关键词
enhanced Fritz John,enhanced KKT,quasinormal multipliers,enhanced multipliers,quasi-normality,augmented Lagrangian method
AI 理解论文
溯源树
样例
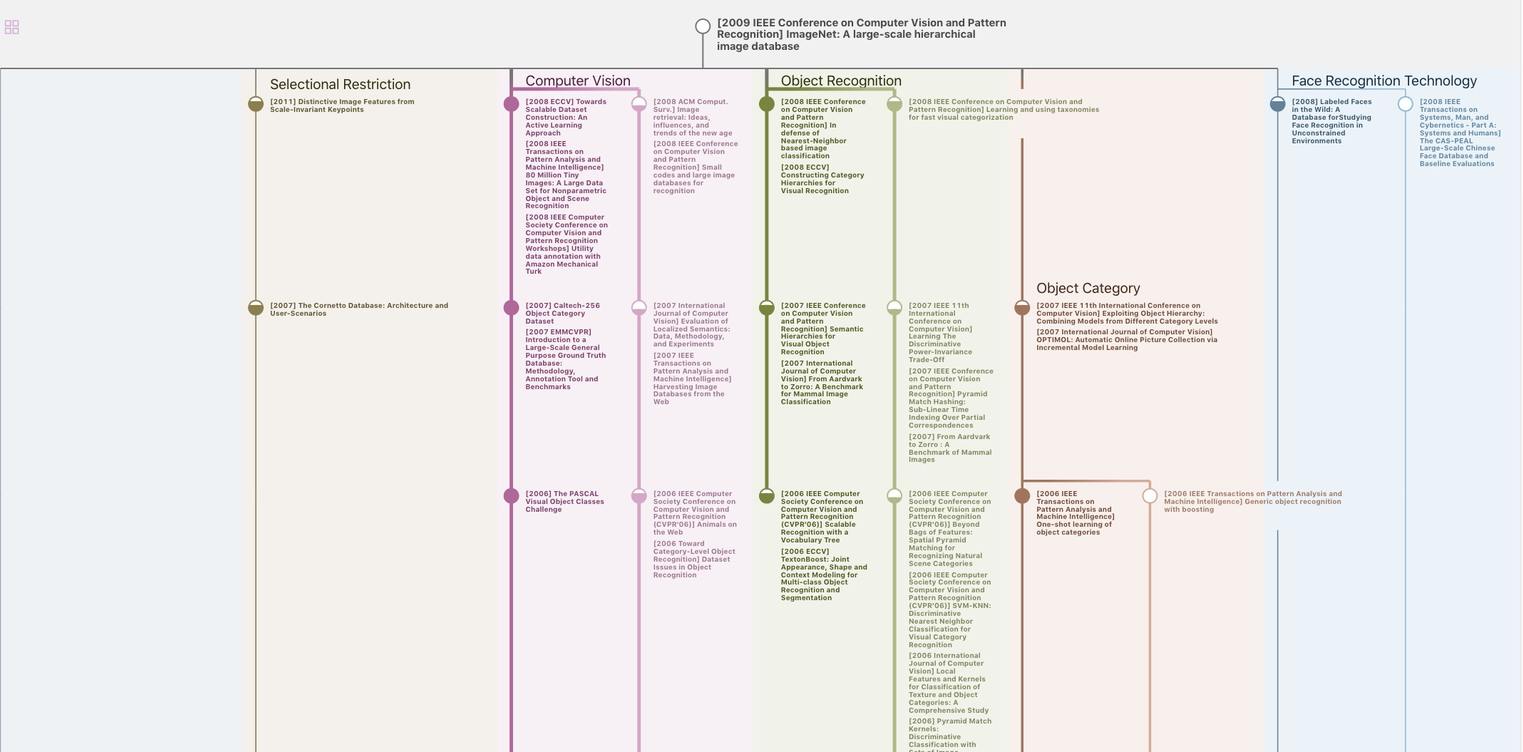
生成溯源树,研究论文发展脉络
Chat Paper
正在生成论文摘要