Deep Reinforcement Learning for Computation Rate Maximization in RIS-Enabled Mobile Edge Computing
IEEE Transactions on Vehicular Technology(2024)
摘要
Reconfigurable intelligent surface (RIS) is a promising technology to enhance the performance of mobile edge computing (MEC) network. Nevertheless, the RIS-enabled MEC network design is a non-trivial problem. This paper investigates the RIS-enabled MEC network, where the Internet of Things devices (IoTDs) with limited energy budgets can offload their partial computation tasks to the base station (BS). We first formulate a sum computation rate maximization problem by jointly designing the RIS phase shifts, and the IoTDs' energy partition strategies for local computing and offloading. Then, to handle the non-convex optimization problem, we propose a deep reinforcement learning (DRL)-based algorithm, in which the twin delayed deep deterministic policy gradient (TD3) algorithm is adopted to optimize the RIS phase shifts and the IoTDs' energy partition strategies. Simulation results show that the proposed TD3 solution can reach a better sum computation rate than the benchmark algorithms.
更多查看译文
关键词
Reconfigurable intelligent surface,mobile edge computing,deep reinforcement learning,computation rate maximization
AI 理解论文
溯源树
样例
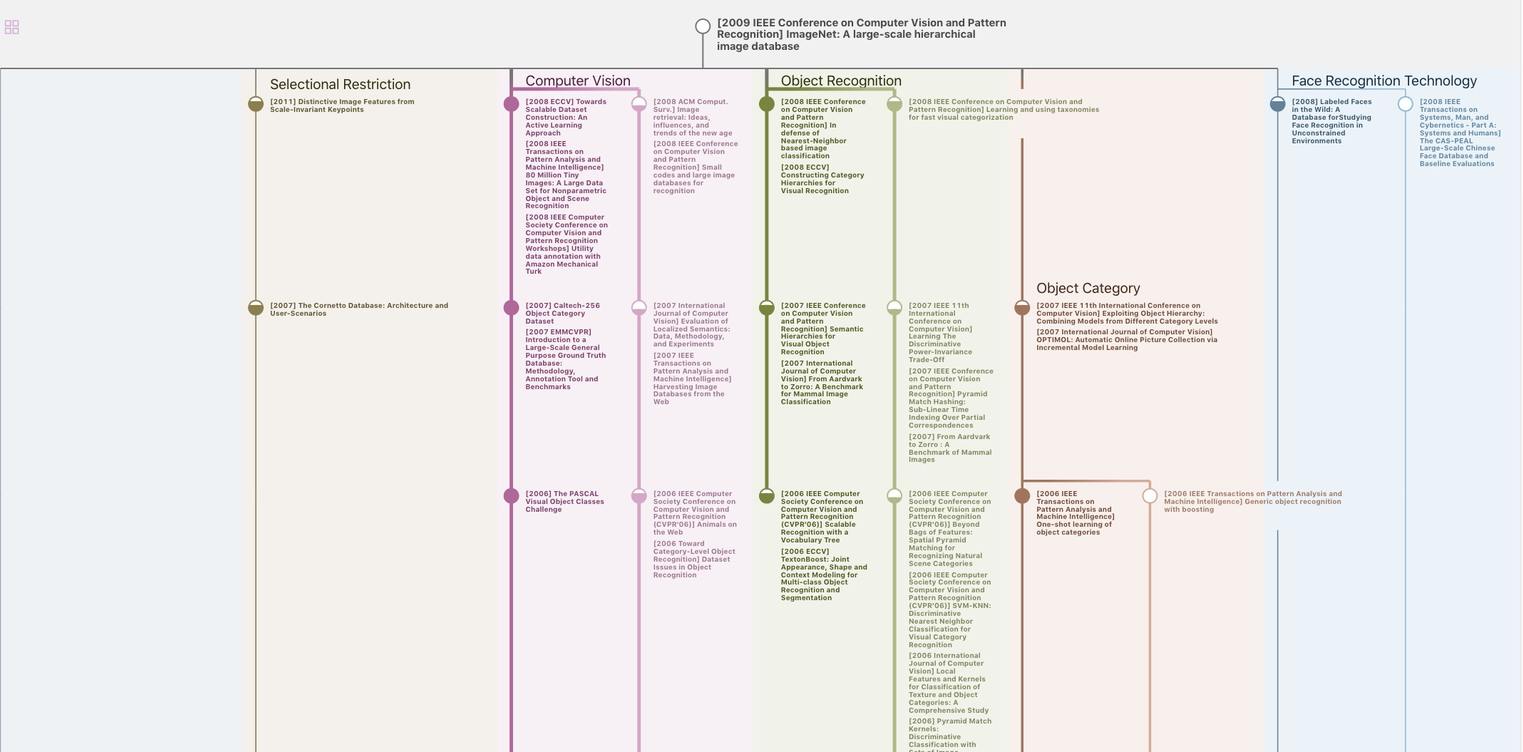
生成溯源树,研究论文发展脉络
Chat Paper
正在生成论文摘要