An Efficient 6G Federated Learning-enabled Energy-Efficient Scheme for UAV Deployment
IEEE Transactions on Vehicular Technology(2024)
摘要
Unmanned Aerial Vehicles (UAVs) are widely used for commercial transportation and data collection in many applications. Recently, UAVs have been used as flying relays to support terrestrial cellular networks for enhancing coverage and connectivity. Multiple UAVs serve as data collectors at a common altitude in a UAV-aided Internet of Things (IoT) network. Data collected is processed by Federated Learning (FL) before being sent to central servers to protect users’ privacy and reduce communication costs. Batteries in UAVs are tiny and efficient only for a short duration, making FL challenging to execute for many iterations. Therefore, a Multi-UAV Energy Efficient Coverage Deployment algorithm based on a Spatial Adaptive Play (MUECD-SAP) is proposed in this article. MUECD uses a modified Particle Swarm Optimization (PSO) to optimize the accurate location of deployed UAVs based on the Signal-toInterference Ratio (SINR) to increase the data collection rate. Also, to make the FL run for multiple iterations, SAP dynamically allocates resources using Deep Deterministic Policy Gradient (DDPG) to optimize the energy consumed and the link latency between the user and the UAV system. The proposed MUECD algorithm outperforms all the state-of-the-art algorithms by achieving an improved data rate and balanced SINR value. The proposed SAP resource allocation strategy has improved the FL execution by 66.67% and attains a very low latency and energy consumed compared to other resource allocation techniques.
更多查看译文
关键词
Unmanned Aerial Vehicles,Coverage Optimization,Resource Allocation,Internet of Things,Federated Learning,Deep Reinforcement Learning
AI 理解论文
溯源树
样例
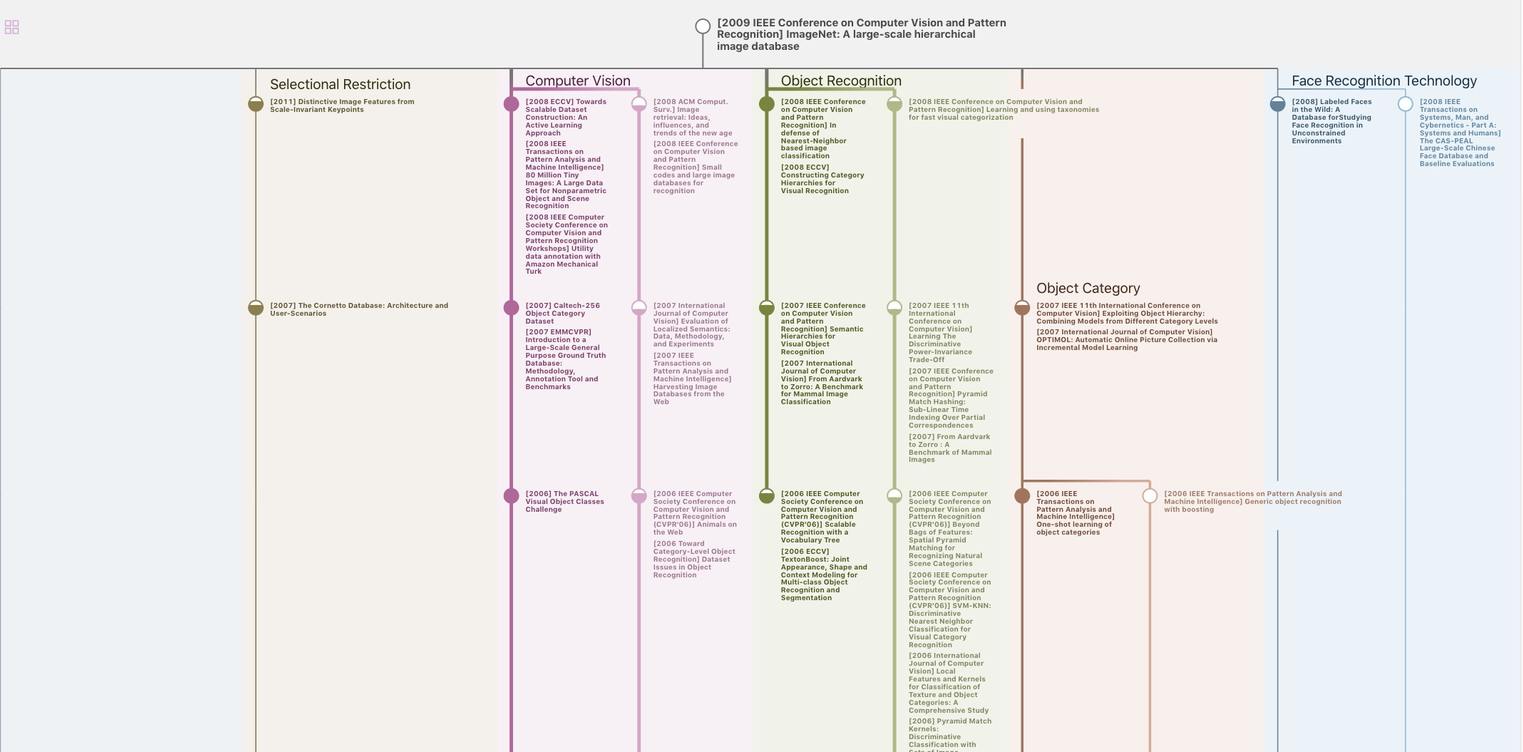
生成溯源树,研究论文发展脉络
Chat Paper
正在生成论文摘要