Graph Structure Learning With Automatic Search of Hyperparameters Based on Genetic Programming
IEEE TRANSACTIONS ON EMERGING TOPICS IN COMPUTATIONAL INTELLIGENCE(2024)
摘要
Graph neural networks (GNNs) rely heavily on graph structures and artificial hyperparameters, which may increase computation and affect performance. Most GNNs use original graphs, but the original graph data has problems with noise and incomplete information, which easily leads to poor GNN performance. For this kind of problem, recent graph structure learning methods consider how to generate graph structures containing label information. The settings of some hyperparameters will also affect the expression of the GNN model. This paper proposes a genetic graph structure learning method (Genetic-GSL). Different from the existing graph structure learning methods, this paper not only optimizes the graph structure but also the hyperparameters. Specifically, different graph structures and different hyperparameters are used as parents; the offspring are cross-mutated through the parents; and then excellent offspring are selected through evaluation to achieve dynamic fitting of the graph structure and hyperparameters. Experiments show that, compared with other methods, Genetic-GSL basically improves the performance of node classification tasks by 1.2%. With the increase in evolution algebra, Genetic-GSL has good performance on node classification tasks and resistance to adversarial attacks.
更多查看译文
关键词
Graph neural networks,hyperparameters optimization,graph structure learning,genetic model
AI 理解论文
溯源树
样例
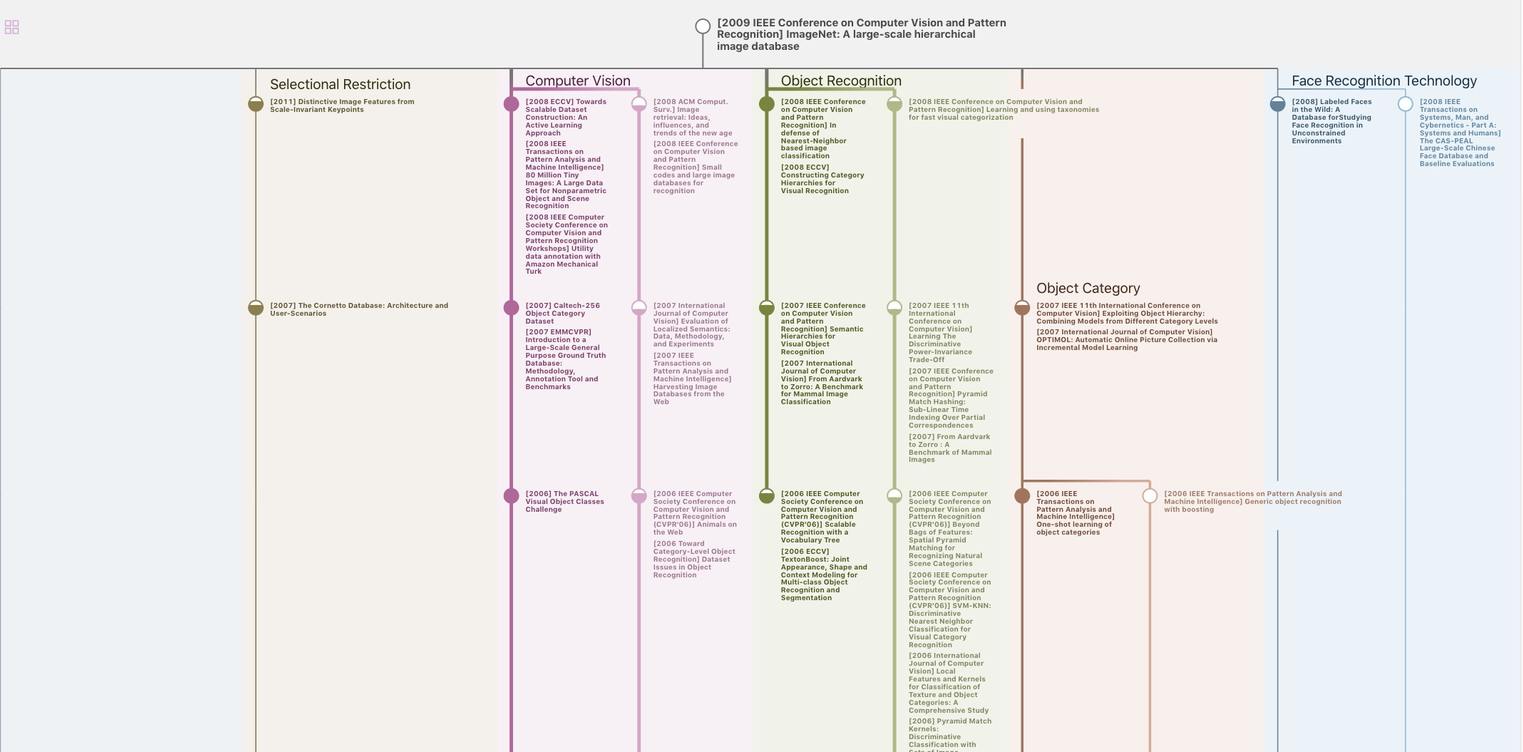
生成溯源树,研究论文发展脉络
Chat Paper
正在生成论文摘要