Forecasting Solar Power Generation Using Evolutionary Mating Algorithm-Deep Neural Networks
Energy and AI(2024)
摘要
This paper proposes an integration of recent metaheuristic algorithm namely Evolutionary Mating Algorithm (EMA) in optimizing the weights and biases of deep neural networks (DNN) for forecasting the solar power generation. The study employs a Feed Forward Neural Network (FFNN) to forecast AC power output using real solar power plant measurements spanning a 34-day period, recorded at 15-minute intervals. The intricate nonlinear relationship between solar irradiation, ambient temperature, and module temperature is captured for accurate prediction. Additionally, the paper conducts a comprehensive comparison with established algorithms, including Differential Evolution (DE-DNN), Barnacles Mating Optimizer (BMO-DNN), Particle Swarm Optimization (PSO-DNN), Harmony Search Algorithm (HSA-DNN), DNN with Adaptive Moment Estimation optimizer (ADAM) and Nonlinear AutoRegressive with eXogenous inputs (NARX). The experimental results distinctly highlight the exceptional performance of EMA-DNN by attaining the lowest Root Mean Squared Error (RMSE) during testing. This contribution not only advances solar power forecasting methodologies but also underscores the potential of merging metaheuristic algorithms with contemporary neural networks for improved accuracy and reliability.
更多查看译文
关键词
Deep Learning Neural Networks,Evolutionary Mating Algorithm, Feed Forward Neural Networks,Metaheuristic Optimizers,Solar PV
AI 理解论文
溯源树
样例
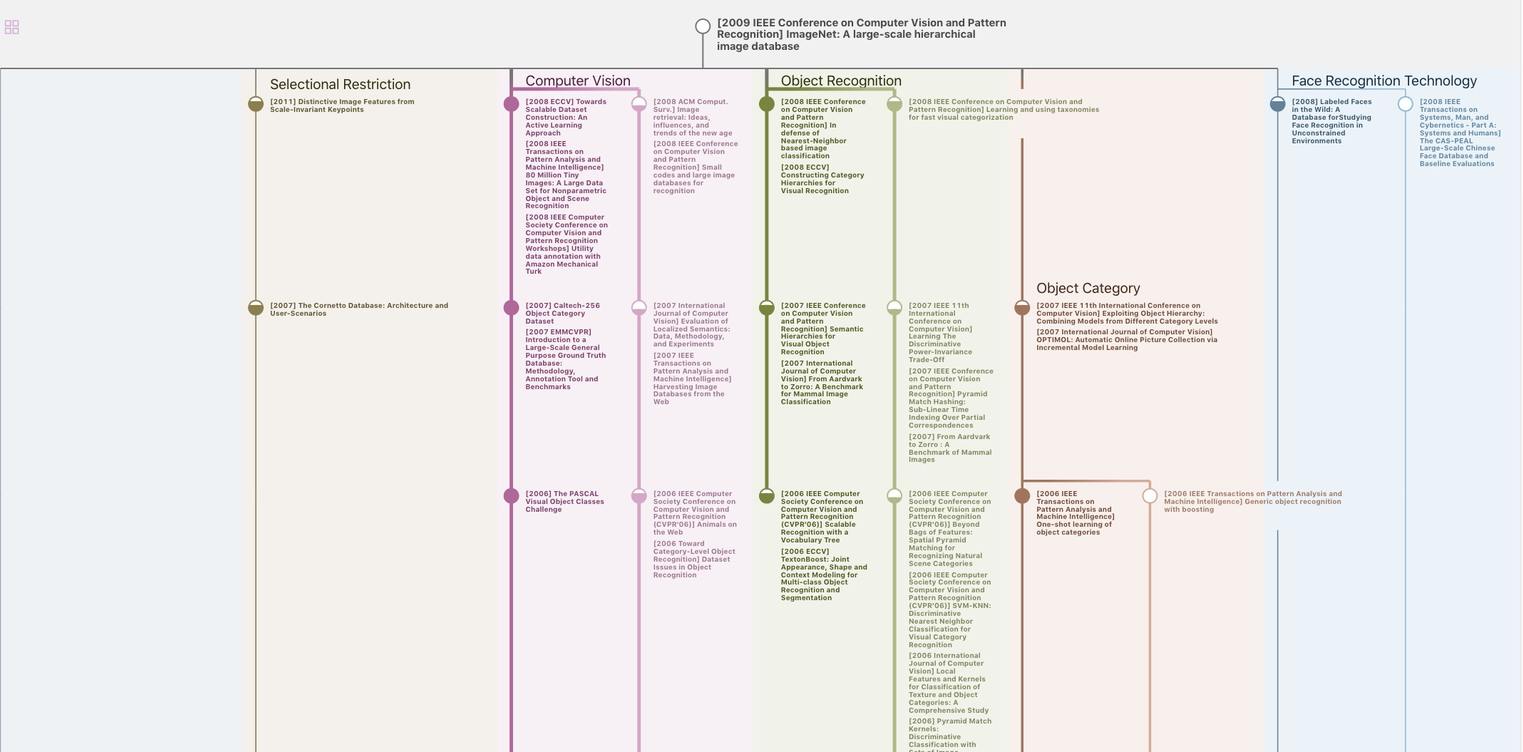
生成溯源树,研究论文发展脉络
Chat Paper
正在生成论文摘要