Discovering Nuclear Models from Symbolic Machine Learning
arxiv(2024)
摘要
Numerous phenomenological nuclear models have been proposed to describe
specific observables within different regions of the nuclear chart. However,
developing a unified model that describes the complex behavior of all nuclei
remains an open challenge. Here, we explore whether novel symbolic Machine
Learning (ML) can rediscover traditional nuclear physics models or identify
alternatives with improved simplicity, fidelity, and predictive power. To
address this challenge, we developed a Multi-objective Iterated Symbolic
Regression approach that handles symbolic regressions over multiple target
observables, accounts for experimental uncertainties and is robust against
high-dimensional problems. As a proof of principle, we applied this method to
describe the nuclear binding energies and charge radii of light and medium mass
nuclei. Our approach identified simple analytical relationships based on the
number of protons and neutrons, providing interpretable models with precision
comparable to state-of-the-art nuclear models. Additionally, we integrated this
ML-discovered model with an existing complementary model to estimate the limits
of nuclear stability. These results highlight the potential of symbolic ML to
develop accurate nuclear models and guide our description of complex many-body
problems.
更多查看译文
AI 理解论文
溯源树
样例
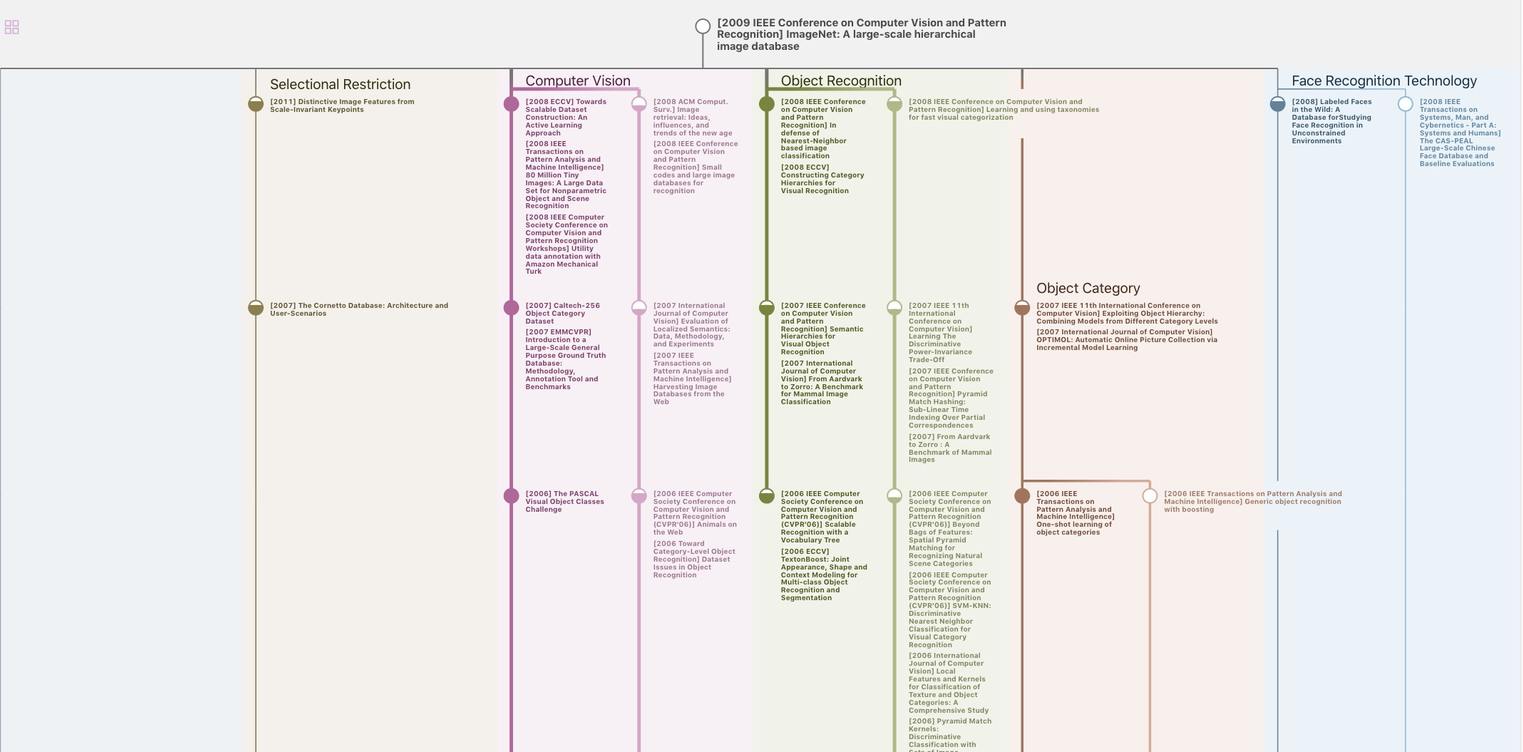
生成溯源树,研究论文发展脉络
Chat Paper
正在生成论文摘要