TL-CCL: Two-level causal contrastive learning for multi-label ocular disease diagnosis with fundus images
Biomedical Signal Processing and Control(2024)
摘要
Objective:
Given the practical clinical demands, multi-label classification to screen multiple retinal diseases is of greater practical significance than diagnosis of a single disease. During the construction of contrastive learning network for classification, it is challenging to distinctly define the positive and negative samples for an anchor image with multiple labels when only using a single image-level representation of the image. Moreover, the spurious correlations in the dependencies of deep learning models are urgent to be removed.
Methods:
We propose a novel two-level causal contrastive learning framework, called TL-CCL, for multi-label ocular disease diagnosis. TL-CCL consists of two branches linked by cumulative learning strategy: a causal contrastive learning branch for feature representation learning and a robust classifier branch for disease classification. For the feature level, we have designed a causal intervention module that focuses on capturing representations causally associated with labels within the feature space of images, which helps distinguish various objects or concepts for multi-label scenarios. For the discrimination level, we adopt a masked entropy regularization loss to reduce the model’s excessive reliance on spurious correlated features.
Results:
The experimental results on the OIA-ODIR dataset demonstrate TL-CCL outperforms other competitive methods.
Conclusion and Significance:
Our framework utilizes a two-level causal design to achieve better classification ability, when the model gradually transitions from feature learning to classifier learning as training epochs increase. This study provides a novel and effective approach for the application of contrastive learning in multi-label medical image classification scenarios.
更多查看译文
关键词
Ocular disease diagnosis,Causal representation learning,Contrastive learning,Multi-label image classification
AI 理解论文
溯源树
样例
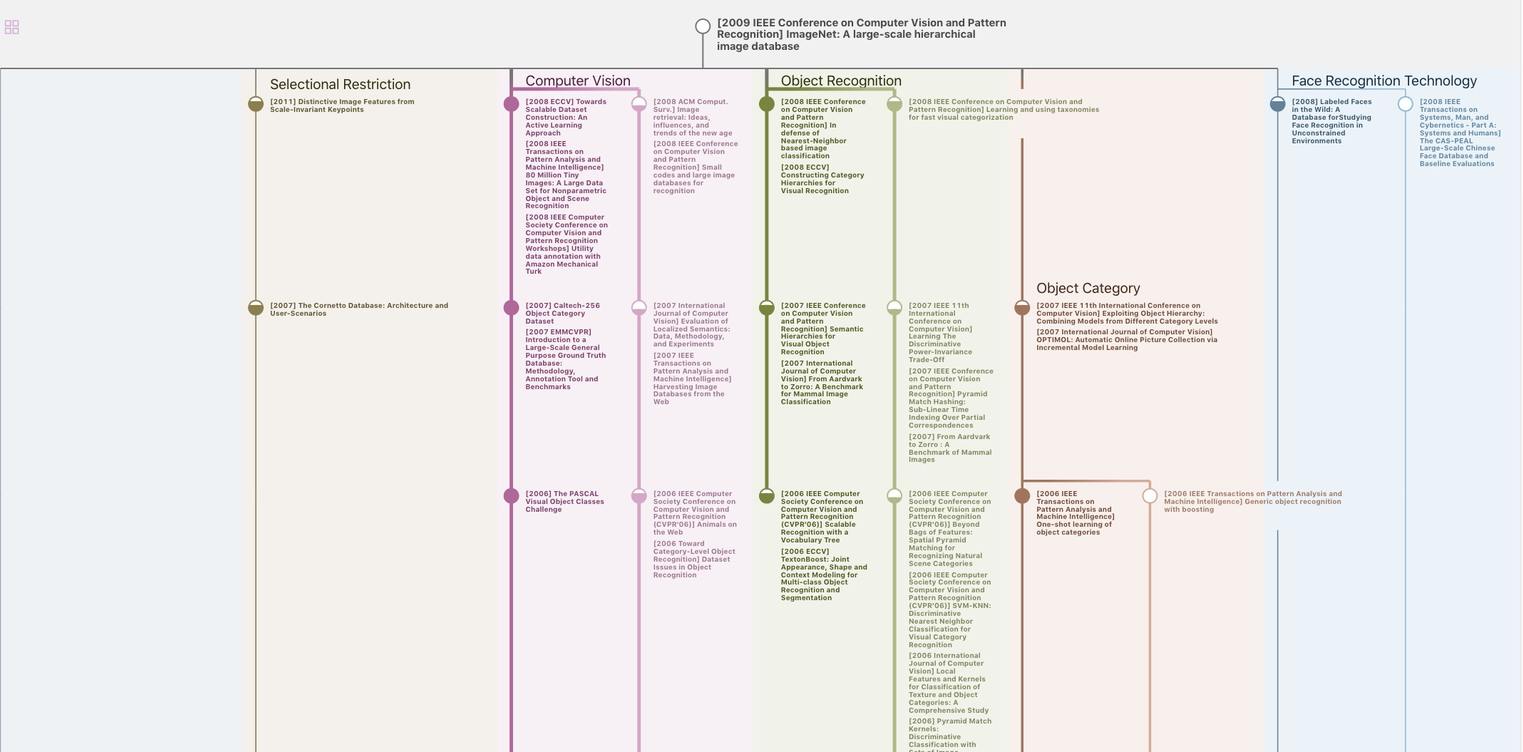
生成溯源树,研究论文发展脉络
Chat Paper
正在生成论文摘要