Production capacity prediction based response conditions optimization of straw reforming using attention-enhanced convolutional LSTM integrating data expansion
Applied Energy(2024)
摘要
As a green and renewable energy source, biomass energy has the potential to solve environmental pollution and resource shortage. The utilization of the straw as a feedstock for the production of clean energy gases and notably methane, via anaerobic processes, has garnered substantial interest within the scientific community. Nevertheless, the intricacies inherent in the biomass synthesis system and the practical constraints associated with experimental operations pose challenges in developing a precise predictive model for the yield estimation when working with limited sample data. Therefore, a novel production prediction method using the synthetic minority oversampling technique (SMOTE) algorithm incorporating an Attention-Enhanced convolutional long short-term memory (SMOTE-ACL) is proposed. The SMOTE algorithm is utilized to extend the original data to build the training and test sets. Then, an embedding layer enhances the local features to higher dimensions, by using a convolutional neural network (CNN) for the feature extraction. Subsequently, the long short-term memory (LSTM) augmented with an attention mechanism is utilized for the temporal prediction and derives the prediction result through the fully-connected layer. Finally, the SMOTE-ACL method is applied to predict the unit production of the straw bioconversion for response conditions optimization. The SMOTE-ACL method integrating local and global information improves the ability to model multidimensional time series data, and achieves the best prediction accuracy than the radial basis function (RBF) neural network, the multilayer perceptron (MLP), the CNN, the recurrent neural network (RNN) and the LSTM. Meanwhile, it is of guiding significance for real-time monitoring of experiments and optimizing the plant production.
更多查看译文
关键词
Straw anaerobic fermentation plant,Yield prediction,Production capacity analysis,Response conditions optimization,Data expansion,Long short-term memory
AI 理解论文
溯源树
样例
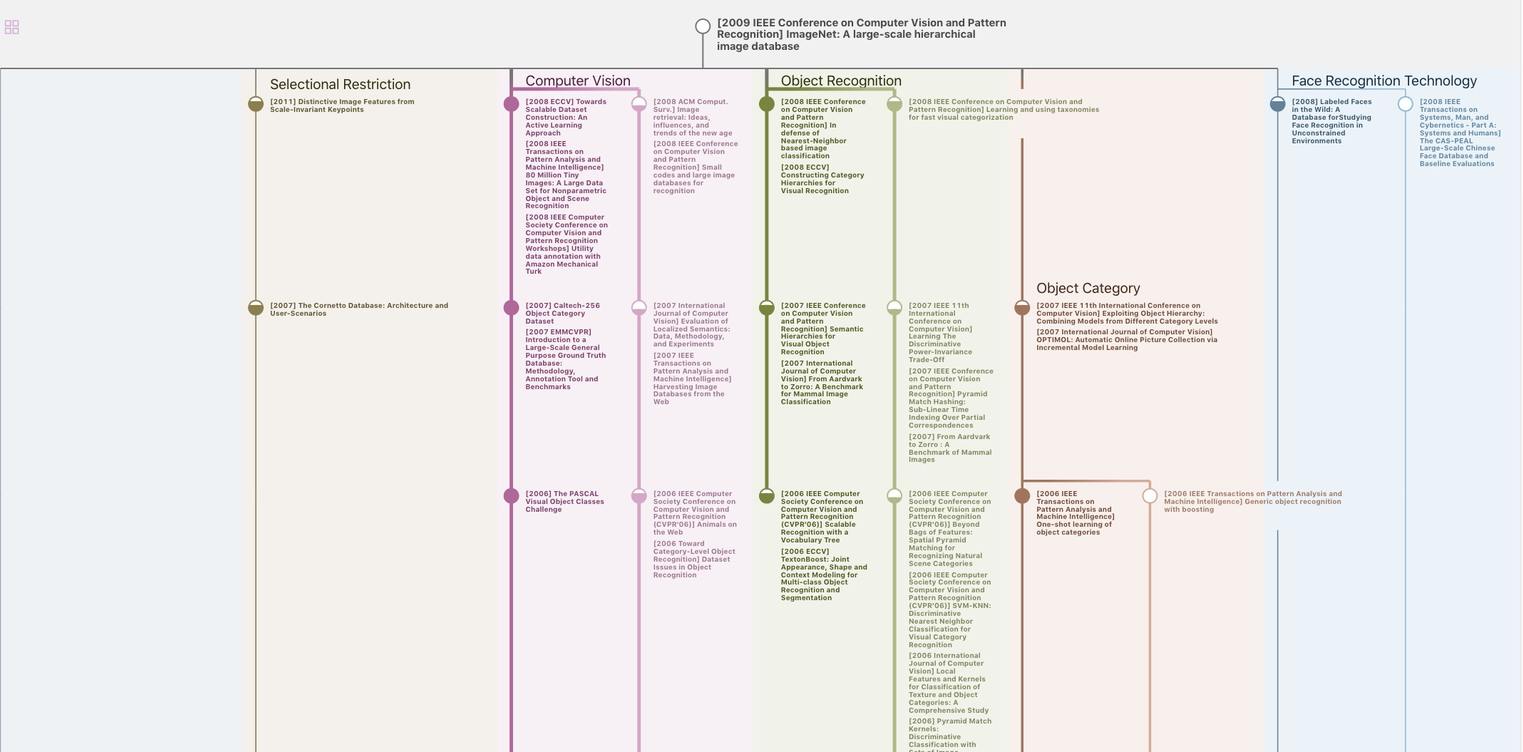
生成溯源树,研究论文发展脉络
Chat Paper
正在生成论文摘要