A novel data-driven reduced order modelling methodology for simulation of humid blowout in wet combustion applications
Energy(2024)
摘要
Computationally inexpensive reduced order models such as Chemical Reactor Networks (CRN) are encouraging tools to obtain fast numerical solutions. However, the accuracy of such models is usually compromised compared to experiments or high-fidelity numerical simulations. Therefore, continued improvement of such models is necessary to ensure reasonable accuracy and fill the need for computationally inexpensive tools. To that end, inputs from computational fluid dynamics simulations have been used to build CRNs in the last 25 years. This can be further improved by the application of data-driven techniques. The present work uses a novel data-driven analysis based on high-fidelity simulation results where the high-dimensional data is first reduced to a low-dimensional manifold and is then clustered into chemically coherent regions. These results along with the high-fidelity simulation results are used to construct a CRN for wet combustion simulations. Wet combustion is a novel clean combustion technique, where an increase in the level of steam dilution distributes the flame, with a risk for flame extinction. This phenomenon, i.e., humid blowout (HBO), was modelled using the above-mentioned CRN. The HBO predicted by the CRN was also tested for thermal power, equivalence ratio, and fuel conditions different from its design point. The error in HBO prediction was quantified by comparing the steam-dilution level at HBO obtained using CRN and that obtained experimentally. The CRN predicted the HBO with an error magnitude less than 5% when the thermal power was unchanged. The maximum error in all tested conditions was 16.65%. Furthermore, a sensitivity analysis revealed that the inclusion of hot gas recirculation through the central recirculation zone, quantified by the mass flow split between the post-flame region and the CRZ, is important in the prediction of HBO, although its accuracy is inconsequential.
更多查看译文
关键词
Data-driven techniques,Reduced-order modelling (ROM),Chemical reactor network,Humid blowout prediction,Wet combustion
AI 理解论文
溯源树
样例
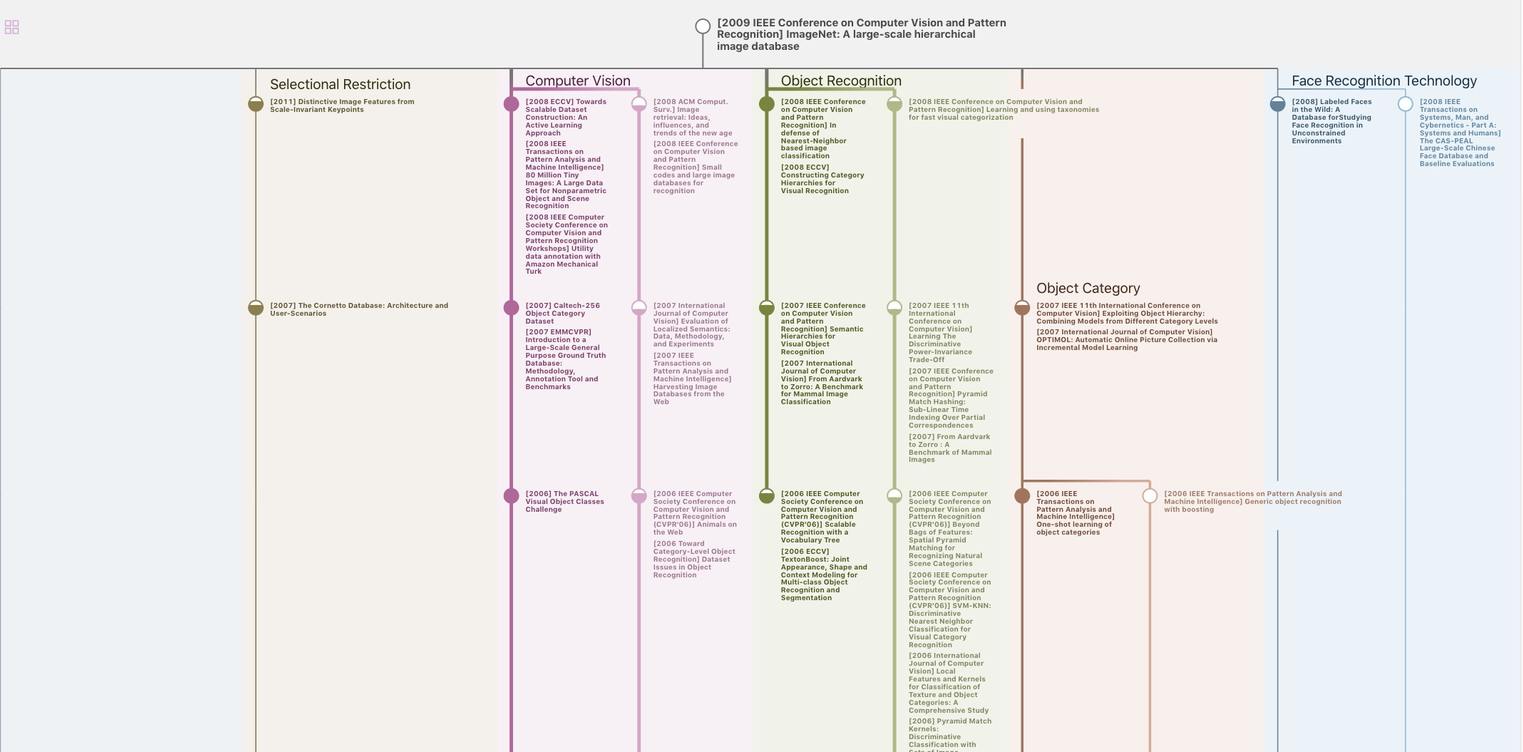
生成溯源树,研究论文发展脉络
Chat Paper
正在生成论文摘要