Spatio-Temporal Activity Detection via Joint Optimization of Spatial and Temporal Localization.
IEEE/CVF Winter Conference on Applications of Computer Vision(2024)
摘要
In this article, we address the problem of spatiotemporal activity detection which requires classifying as well as localizing human activities both in space and time from videos. To this end, we propose a novel single-stage and end-to-end trainable deep learning framework that can jointly optimize spatial and temporal localization of ac-tivities. Leveraging shared spatiotemporal feature maps, the proposed framework performs actor detection, activity tube building, as well as temporal localization of activities, all within a single network. The proposed framework outperforms the current state-of-the-art methods in spatiotemporal activity detection on the challenging UCF101-24 benchmark. By utilizing solely RGB input, it achieves a video-mAP of 60.1%, and further pushes the bar to 61.3% when incorporating both RGB and FLOW inputs. More-over, it attains a highly competitive frame-mAP of 74.9%.
更多查看译文
关键词
Joint Optimization,Temporal Localization,Spatiotemporal Activity,Time And Space,Activity Time,Local Actors,Feature Maps,Shared Features,Spatiotemporal Characteristics,Spatiotemporal Map,Input RGB,Temporal Dimension,Object Detection,Spatial Dimensions,End Time,Start Time,Bounding Box,Confidence Score,Model Configuration,Optical Flow,Non-maximum Suppression,Multi-scale Architecture,Activation Segment,State Of The Art Methods,Activity Classification,Temporal Loss,Mean Average Precision,Channel Dimension,Temporal Classification,Video Segments
AI 理解论文
溯源树
样例
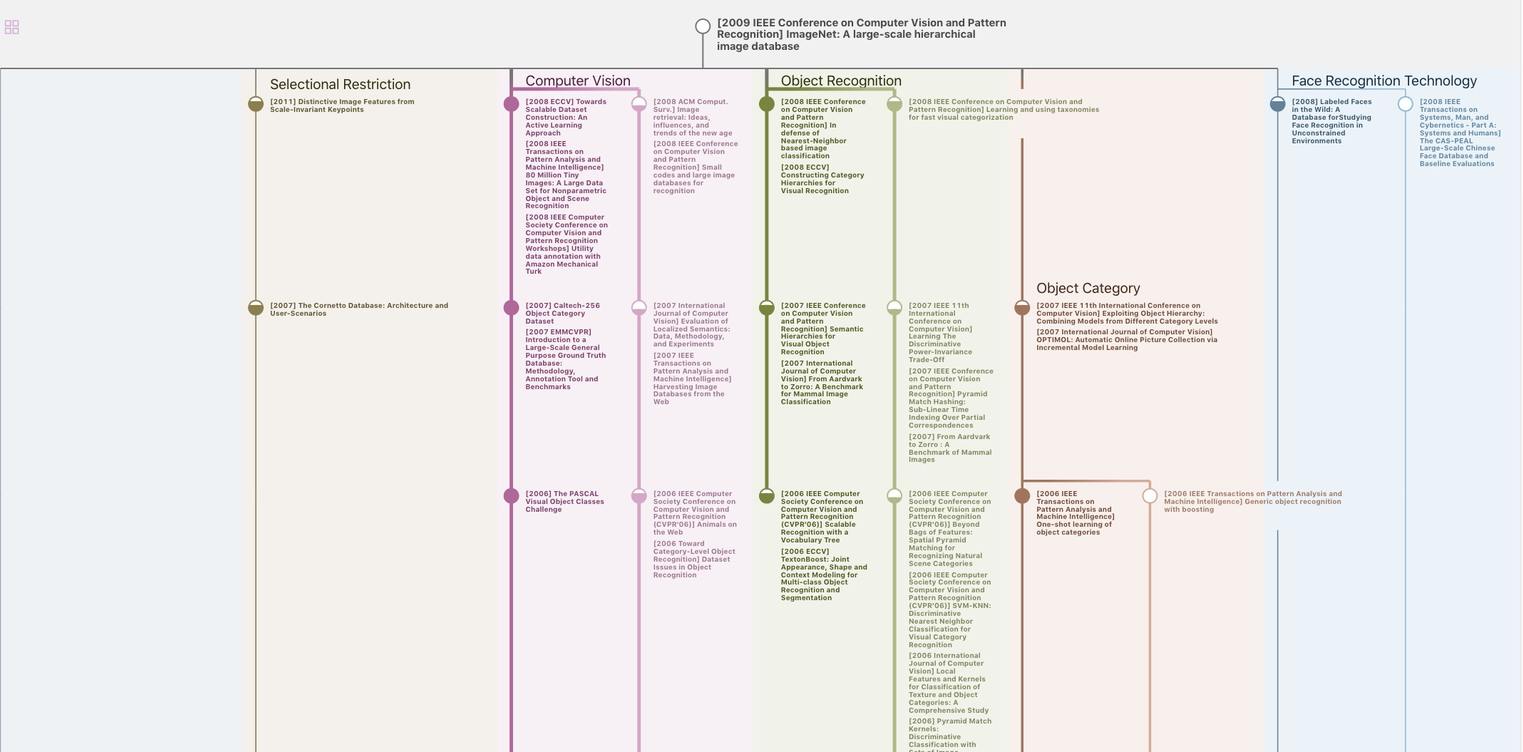
生成溯源树,研究论文发展脉络
Chat Paper
正在生成论文摘要