Demonstration of DB-GPT: Next Generation Data Interaction System Empowered by Large Language Models
arxiv(2024)
摘要
The recent breakthroughs in large language models (LLMs) are positioned to
transition many areas of software. The technologies of interacting with data
particularly have an important entanglement with LLMs as efficient and
intuitive data interactions are paramount. In this paper, we present DB-GPT, a
revolutionary and product-ready Python library that integrates LLMs into
traditional data interaction tasks to enhance user experience and
accessibility. DB-GPT is designed to understand data interaction tasks
described by natural language and provide context-aware responses powered by
LLMs, making it an indispensable tool for users ranging from novice to expert.
Its system design supports deployment across local, distributed, and cloud
environments. Beyond handling basic data interaction tasks like Text-to-SQL
with LLMs, it can handle complex tasks like generative data analysis through a
Multi-Agents framework and the Agentic Workflow Expression Language (AWEL). The
Service-oriented Multi-model Management Framework (SMMF) ensures data privacy
and security, enabling users to employ DB-GPT with private LLMs. Additionally,
DB-GPT offers a series of product-ready features designed to enable users to
integrate DB-GPT within their product environments easily. The code of DB-GPT
is available at Github(https://github.com/eosphoros-ai/DB-GPT) which already
has over 10.7k stars.
更多查看译文
AI 理解论文
溯源树
样例
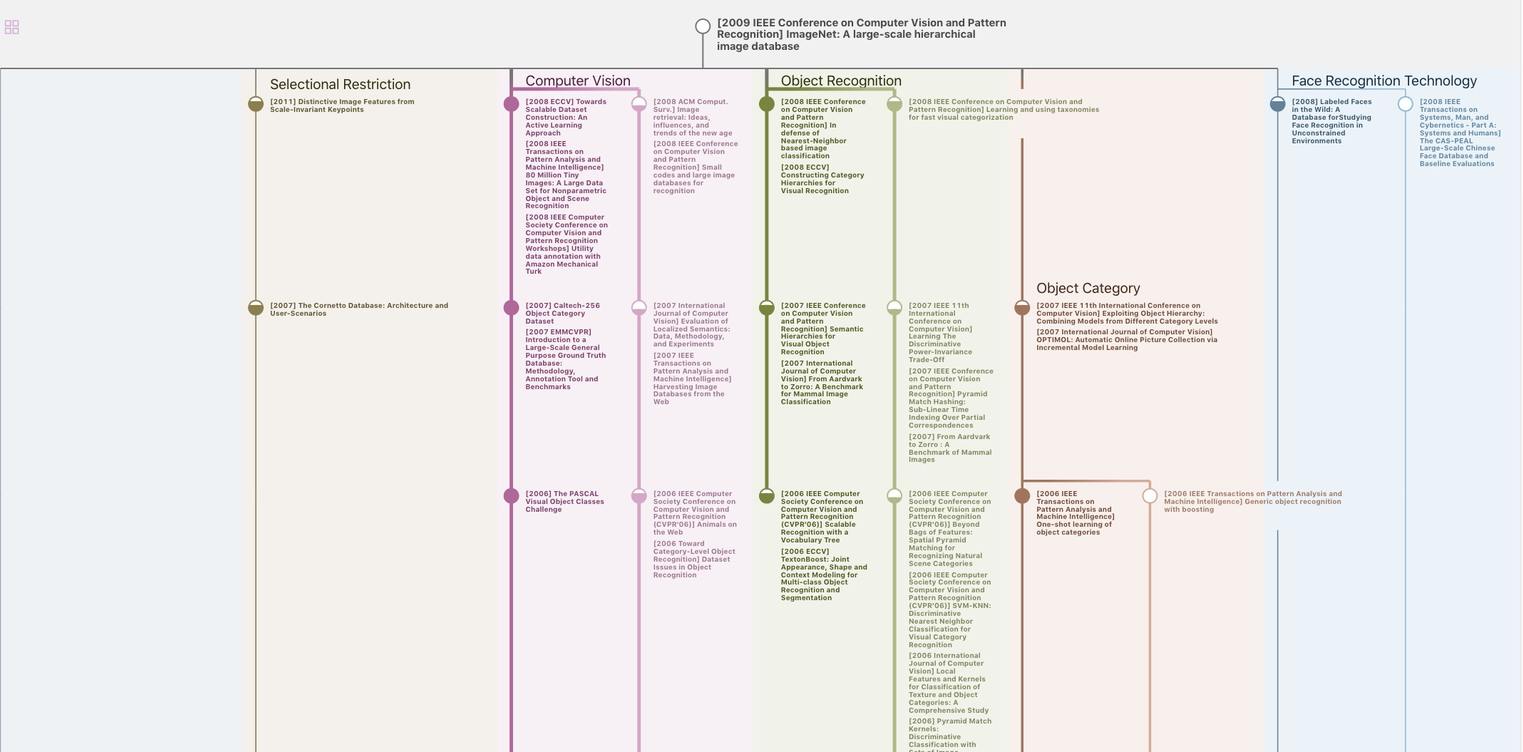
生成溯源树,研究论文发展脉络
Chat Paper
正在生成论文摘要