Sparseness and Correntropy-Based Block Diagonal Representation for Robust Subspace Clustering
IEEE SIGNAL PROCESSING LETTERS(2024)
摘要
Block diagonal representation, which aims to compel the desired representation coefficient to have a block diagonal structure directly, has extensive applications in the domains of computer vision and machine learning. However, single residual modeling in existing works is not robust enough when handling complex noise (i.e., sparse noise and impulsive noise) in reality. To overcome this challenge, a novel Sparseness and Correntropy-based Block Diagonal Representation (SC-BDR) model is proposed, which is able to pursue ideal block diagonal representation and effectively deal with various types of noise. Furthermore, the corresponding optimization algorithm is designed for the proposed problem, and we also conduct extensive experiments to demonstrate the robustness and effectiveness of the SC-BDR model on real-world data.
更多查看译文
关键词
Noise,Sparse matrices,Robustness,Clustering algorithms,Vectors,Optimization,Minimization,Block diagonal representation,low-rank representation,subspace clustering,noise
AI 理解论文
溯源树
样例
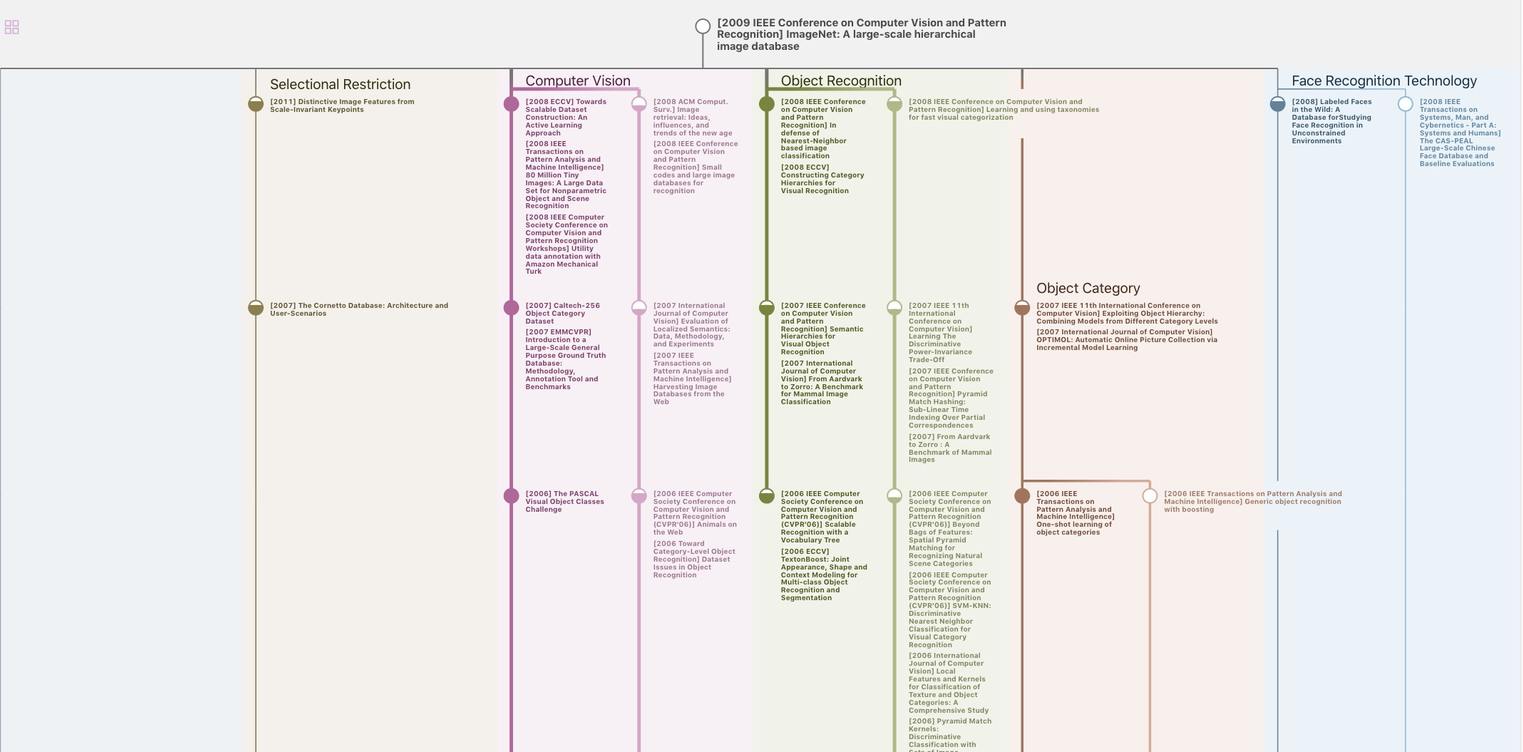
生成溯源树,研究论文发展脉络
Chat Paper
正在生成论文摘要