MalGNE: Enhancing the Performance and Efficiency of CFG-based Malware Detector by Graph Node Embedding in Low Dimension Space
IEEE Transactions on Information Forensics and Security(2024)
摘要
The rich semantic information in Control Flow Graphs (CFGs) of executable programs has made Graph Neural Networks (GNNs) a key focus for malware detection. However, existing CFG-based detection techniques face limitations in node feature extraction, such as information loss, neglect of execution sequence information, and redundancy in representation vectors. These limitations compromise the balance between high efficiency and precision when training detectors. Addressing this, we introduce an innovative Malware CFG Node Embedding (MalGNE) method. This approach utilizes a novel instruction encoding rule to address the Out-Of-Vocabulary(OOV) problem, generates high-quality initial vectors. Then, it employs aggregation layer and sequence layer to extract node aggregation feature and execution sequence feature, in conjunction with GNNs to develop a pre-trained node embedding model. The model maps the semantic information of node assembly instruction sequences into a compact, low-dimensional continuous space, ensuring high-quality feature extraction, and enhancing the performance and efficiency of the detector. We trained the MalGNE model using the BIG 2015 dataset and validated MalGNE-enhanced detector on the SOREL-20M and BODMAS datasets. MalGNE-enhanced detector demonstrates outstanding performance and efficiency in low-dimensional spaces, especially when the dimensionality of the node feature vector is reduced to 16. MalGNE-enhanced detector not only maintains a high detection accuracy of 95.49%. sacrificing only about 1.7% of accuracy to save approximately 73% of training time compared to 128 dimensions.
更多查看译文
关键词
Malware Detection,Node Embedding,Control Flow Graph,Graph Neural Network
AI 理解论文
溯源树
样例
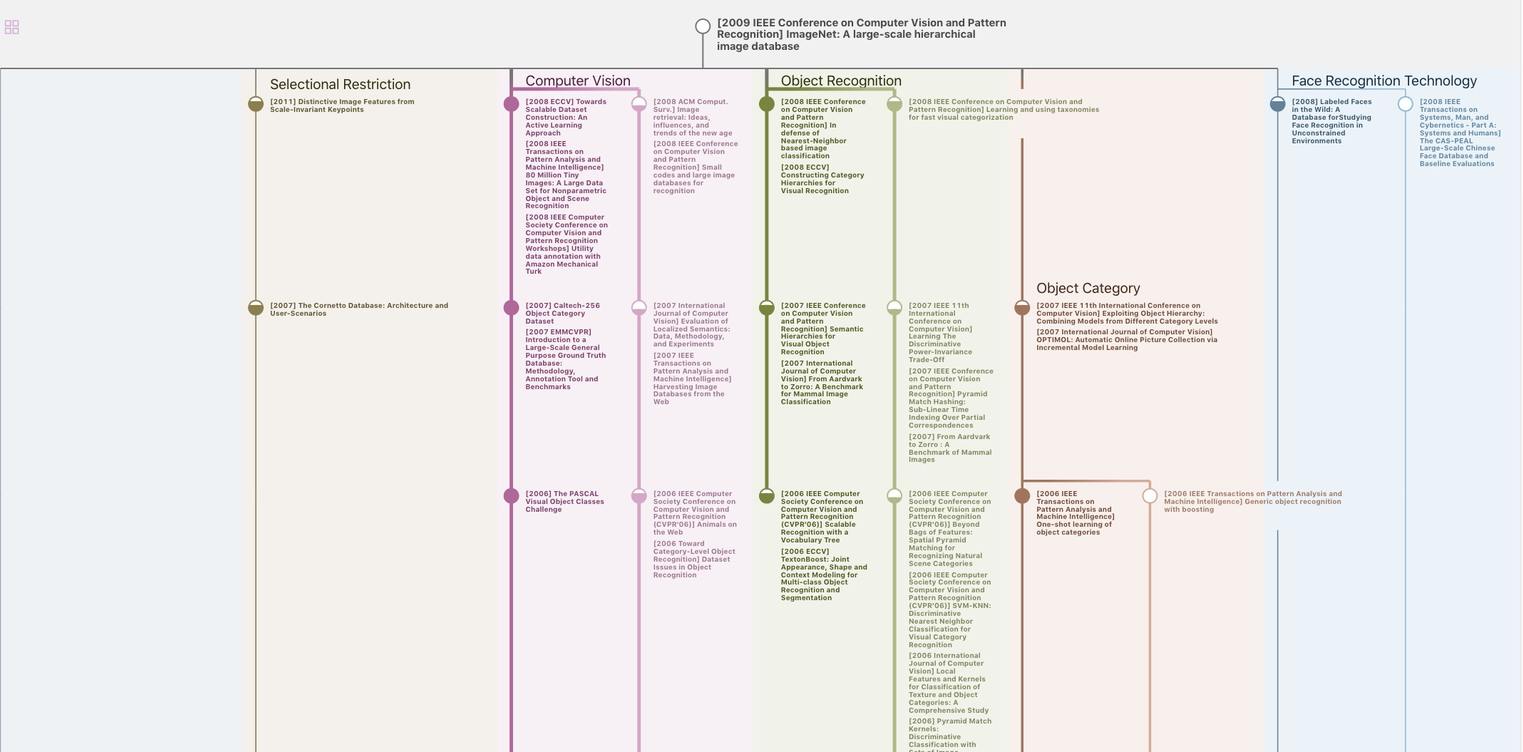
生成溯源树,研究论文发展脉络
Chat Paper
正在生成论文摘要