SYRFA: SYnthetic-to-Real adaptation via Feature Alignment for Video Anomaly Detection
IEEE Access(2024)
摘要
Video Anomaly Detection (VAD) has garnered significant attention in computer vision, especially with the exponential growth of surveillance videos. Recently, the synthetic dataset has been released to address the imbalance problem between normal and abnormal scenarios in real-world datasets by providing various combinations of events. Motivated by the release of synthetic datasets, many studies have attempted to handle domain shifts by generating synthetic-real or real-synthetic abnormal scenarios. However, these approaches still suffer from a substantial computation burden due to the generation model. In this paper, we aim to alleviate the domain gap without relying on any generation model. We propose a novel framework named the SYnthetic-to-Real via Feature Alignment (SYRFA) for VAD. The SYRFA consists of two learning phases: learning synthetic knowledge and adaptation to the real-world domain. These two learning phases facilitate the incorporation of rich synthetic knowledge into the real-world domain. To address the domain shift between synthetic and real domains, we introduce consistency learning, aligning feature representations to map closely between the synthetic and real-world domains. Additionally, in the adaptation phase, we propose the Residual Additional Parameters (RAP), a simple yet effective approach for handling domain gaps. RAP is designed with a residual path for learning local patterns, crucial in VAD due to circumstantial feature representation. It contributes to obtaining transferable feature representations with fewer additional computations. The proposed framework demonstrates superior performance on VAD benchmark datasets. Especially, Our framework outperforms other methods by a margin of 0.8% on ShanghaiTech. Moreover, the ablation study highlights the effectiveness of the proposed framework and RAP.
更多查看译文
关键词
Video Anomaly Detection,Domain Adaptation,Synthetic-to-Real
AI 理解论文
溯源树
样例
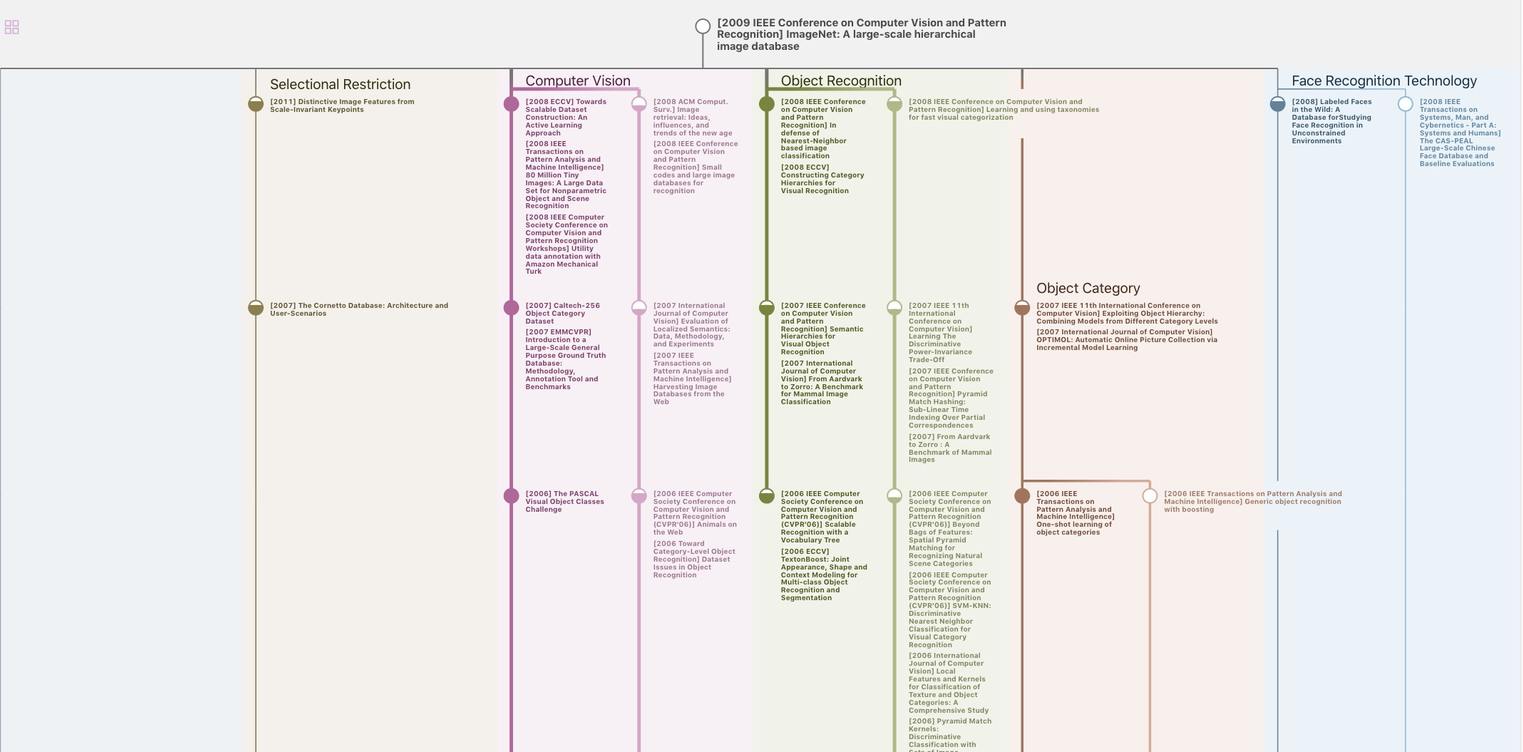
生成溯源树,研究论文发展脉络
Chat Paper
正在生成论文摘要