Detection of distracted driving through the analysis of real-time driver, vehicle, and roadway volatilities
JOURNAL OF TRANSPORTATION SAFETY & SECURITY(2024)
摘要
Distracted driving adversely impacts drivers' decision-making and leads to safety-critical events (SCEs). Early detection of driver distraction is critical to prevent traffic crashes by providing warning messages to drivers and the surrounding vehicles. This study harnesses real-time multidimensional data collected through sensors that examine the variations in driver biometrics, vehicle kinematics, and roadway surroundings in different driving scenarios conducted on a Multimodal Virtual Reality Simulator. The driving behaviors of the study participants were examined under various visual detection response tasks of increasing complexity. The study classifies driving behaviors on a 5-level ordinal scale by estimating a Panel Ordered Logit Model, Random Forest, and Artificial Neural Network, using real-time volatilities in driver biometric signals, vehicle speed and acceleration, and roadway surroundings. The study results reveal that the driver gaze and the coefficients of variation in vehicle speed, driver eye movements, vehicular distances from the lane centerline, and the following vehicle significantly impact distracted driving. The study's findings align with the principles of the safe systems approach by emphasizing the development of proactive safety measures in the form of feedback and warning the driver and surrounding vehicles of a potential distracted driving event, helping to foster safer user behavior and vehicles.
更多查看译文
关键词
Distracted driving,driving impairment,driver biometrics,driving volatilities,machine learning
AI 理解论文
溯源树
样例
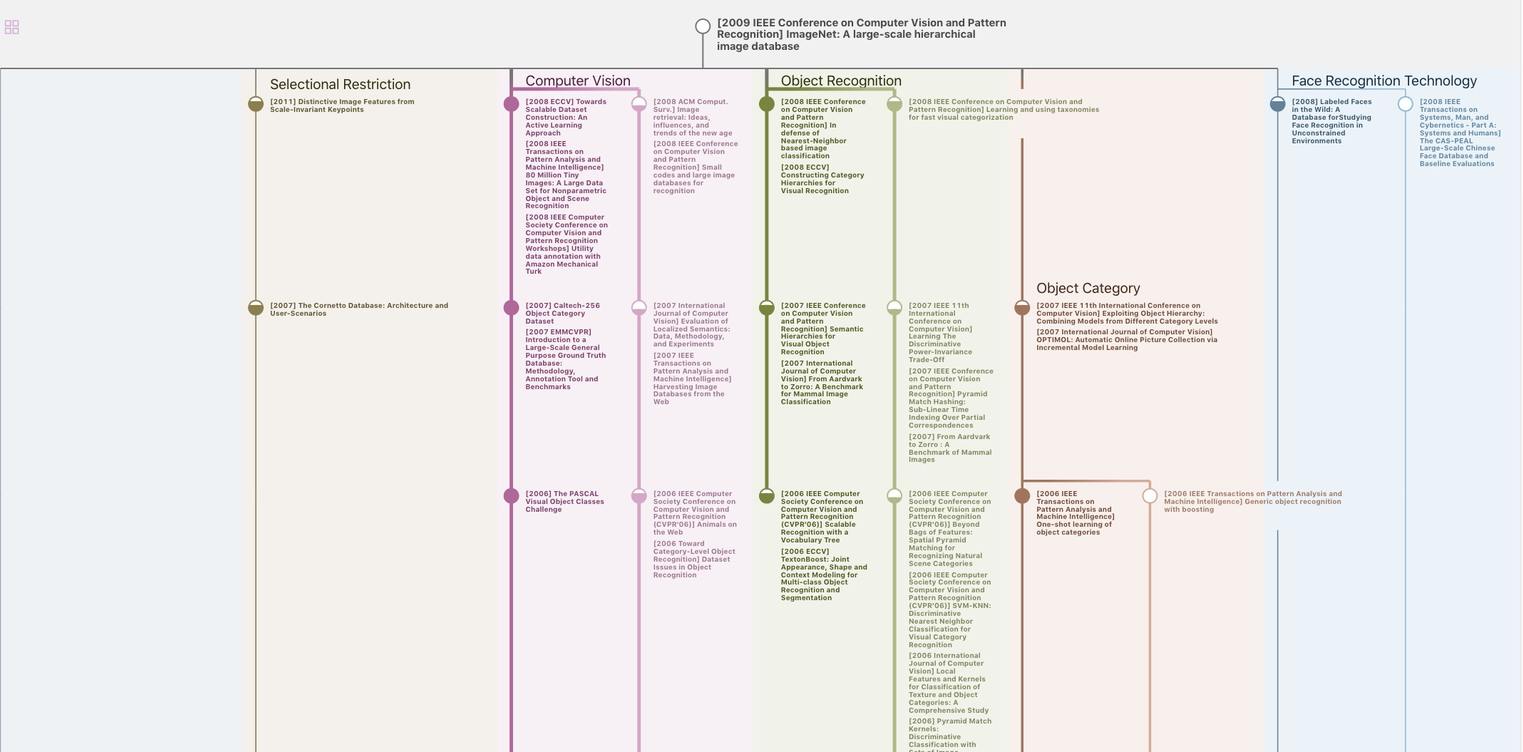
生成溯源树,研究论文发展脉络
Chat Paper
正在生成论文摘要