Forecasting Medium-Term F10.7 Using the Deep-Learning Informer Model
Solar Physics(2024)
摘要
The daily 10.7-cm solar radio flux (F10.7) is one of the most important solar activity indices and has been widely applied in various space environment modeling as a crucial parameter. In this study, we adopt a deep-learning Informer model, based on the transformer architecture to predict the medium-term F10.7 index, which uses 48 historical daily F10.7 indices as input to directly forecast the following 1 – 27 days’ F10.7 index. The model is demonstrated to be effective and to have superior performance compared with other widely-used forecasting techniques: two statistical methods provided by British Geological Survey (BGS), Space Weather Prediction Center (SWPC), and a multiflux neural network method provided by Collecte Localisation Satellites (CLS). In comparison, the Informer model significantly improves the forecast accuracy for the prediction horizon larger than 6 days, especially during the solar activity descending phase and at the solar activity minimum. For its effectiveness, accurate prediction capability and the advantage in F10.7 forecasting with longer horizon, the Informer could be potentially used as a candidate model for space weather operational forecasting.
更多查看译文
关键词
Space weather,F10.7 index,Deep learning,Informer
AI 理解论文
溯源树
样例
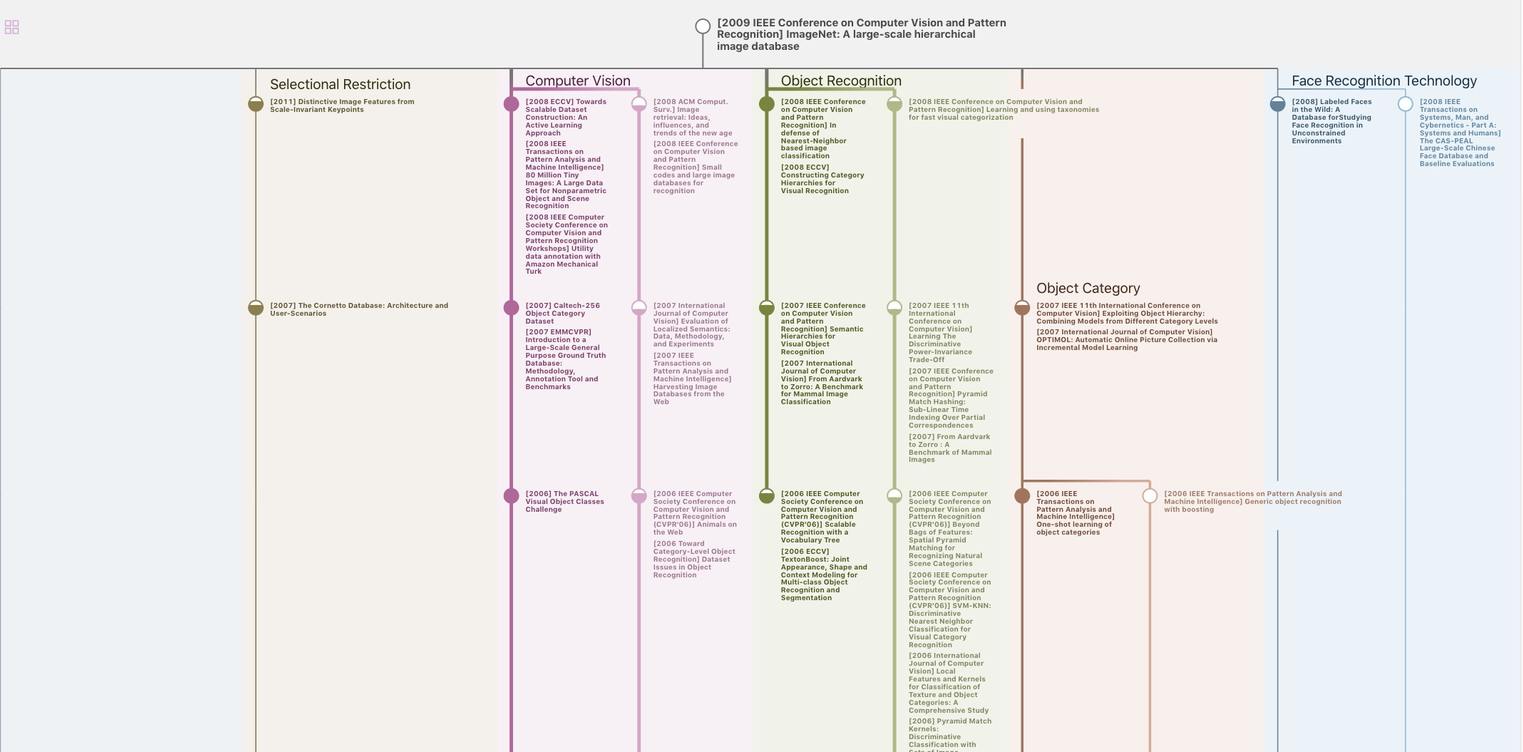
生成溯源树,研究论文发展脉络
Chat Paper
正在生成论文摘要