A Low-Power DNN Accelerator With Mean-Error-Minimized Approximate Signed Multiplier
IEEE Open Journal of Circuits and Systems(2024)
摘要
Approximate computing is an emerging and effective method for reducing energy consumption in digital circuits, which is critical for energy-efficient performance improvement of edge-computing devices. In this paper, we propose a low-power DNN accelerator with novel signed approximate multiplier based on probability-optimized compressor and error compensation. The probability-optimized compressor is customized for partial product matrix (PPM) of signed operands, which gets the optimal logic circuit after probabilistic analysis and optimization. At the same time, we explored the PPM truncation method, found out the impact of different partial product (PP) truncation numbers on circuit benefit and error, and achieved a more ideal performance-error tradeoff through a reasonable error compensation method. In the optimal case of 8 bits, the proposed approximate multiplier saves 49.84% power, 46.41% area and 24.65% delay compared to the exact multiplier. We employed the proposed approximate multiplier in the vector systolic array as the processing element (PE). Under the VGG-16 evaluation, the proposed accelerator achieves performance improvement of energy efficiency
$1.96\times $
, while the error loss was only 0.95%.
更多查看译文
关键词
Approximate computing,multiplier,compressor,probability analysis,truncation,vector,systolic array,accelerator,mean error,DNN
AI 理解论文
溯源树
样例
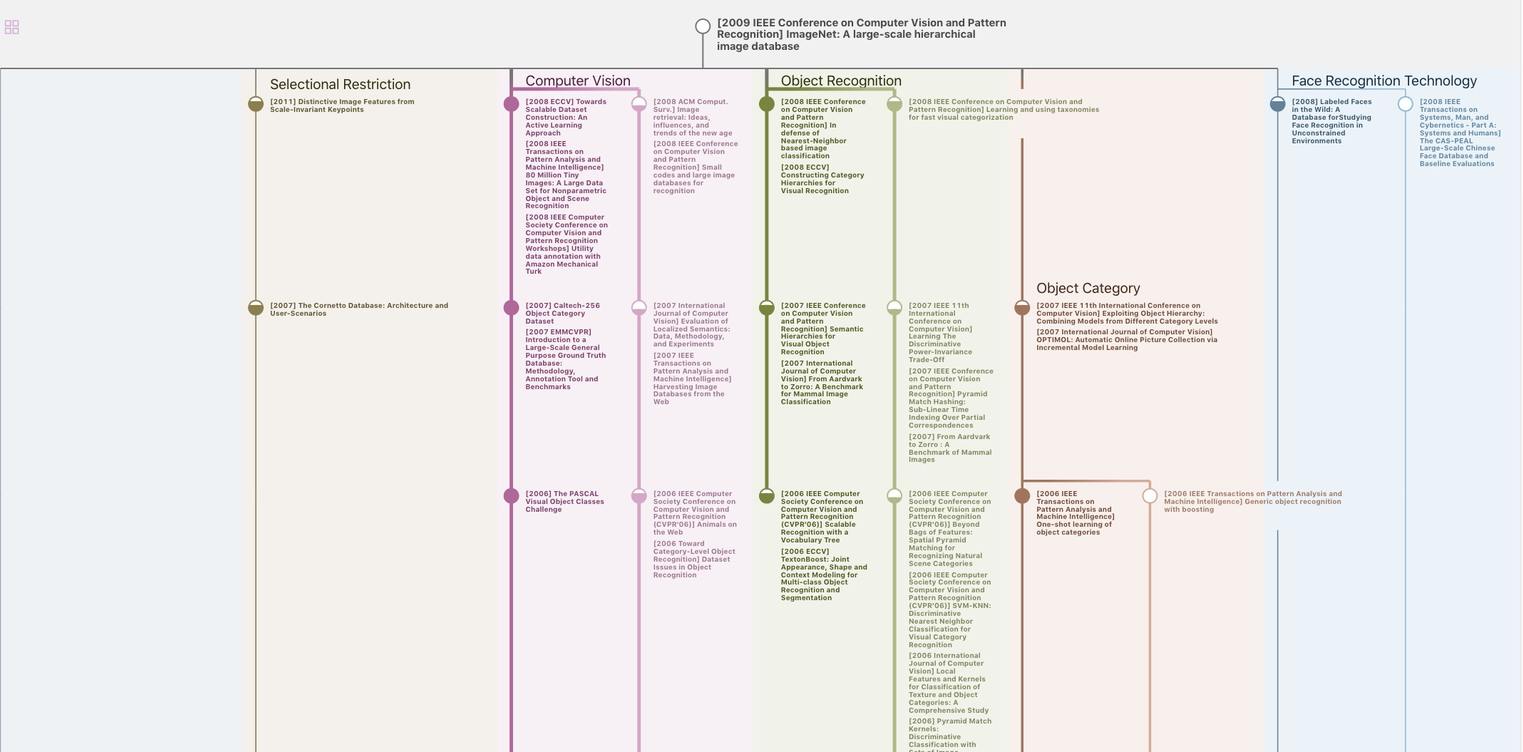
生成溯源树,研究论文发展脉络
Chat Paper
正在生成论文摘要