Constructing synthetic datasets with generative artificial intelligence to train large language models to classify acute renal failure from clinical notes.
Journal of the American Medical Informatics Association : JAMIA(2024)
摘要
OBJECTIVES:To compare performances of a classifier that leverages language models when trained on synthetic versus authentic clinical notes.
MATERIALS AND METHODS:A classifier using language models was developed to identify acute renal failure. Four types of training data were compared: (1) notes from MIMIC-III; and (2, 3, and 4) synthetic notes generated by ChatGPT of varied text lengths of 15 (GPT-15 sentences), 30 (GPT-30 sentences), and 45 (GPT-45 sentences) sentences, respectively. The area under the receiver operating characteristics curve (AUC) was calculated from a test set from MIMIC-III.
RESULTS:With RoBERTa, the AUCs were 0.84, 0.80, 0.84, and 0.76 for the MIMIC-III, GPT-15, GPT-30- and GPT-45 sentences training sets, respectively.
DISCUSSION:Training language models to detect acute renal failure from clinical notes resulted in similar performances when using synthetic versus authentic training data.
CONCLUSION:The use of training data derived from protected health information may not be needed.
更多查看译文
AI 理解论文
溯源树
样例
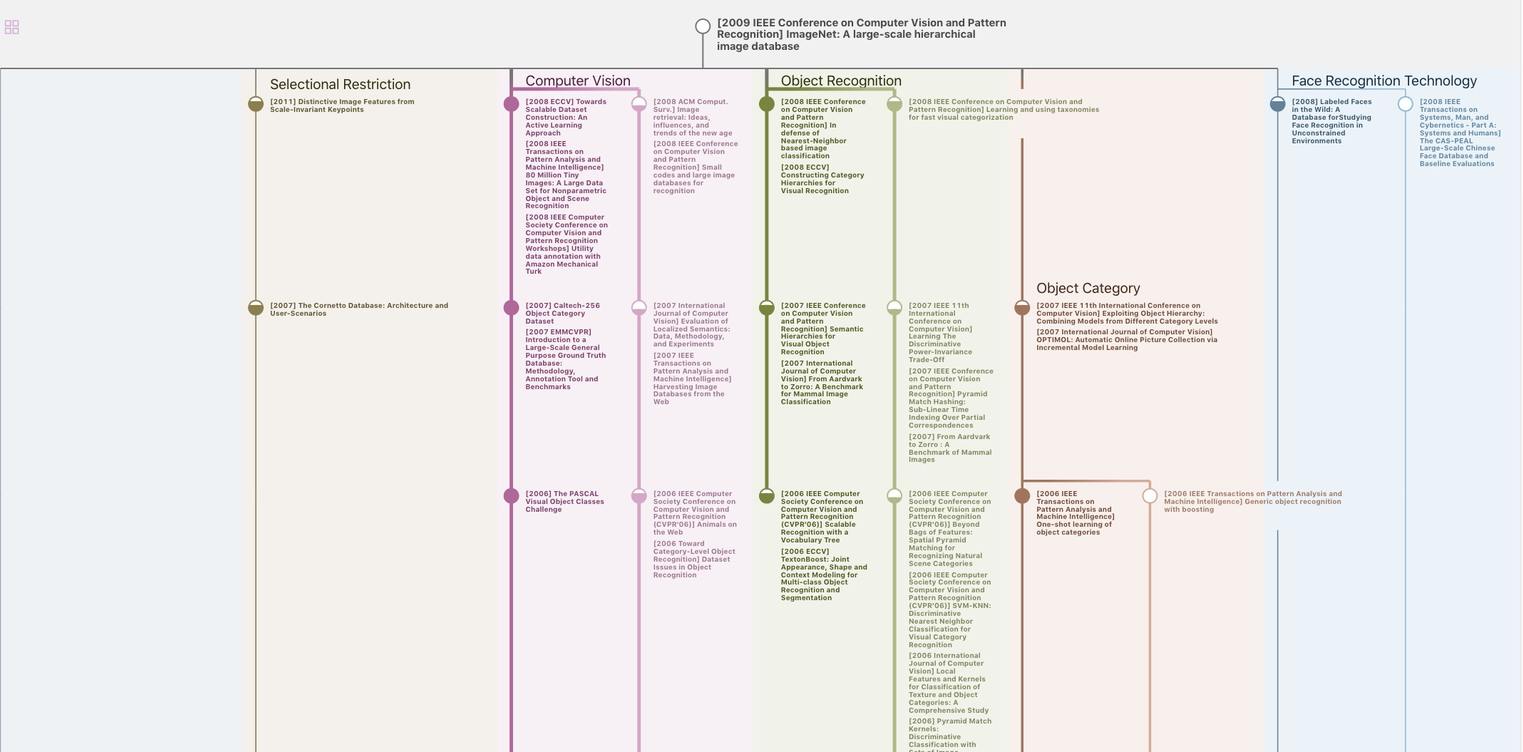
生成溯源树,研究论文发展脉络
Chat Paper
正在生成论文摘要