Time Series Forecasting with Missing Data Using Generative Adversarial Networks and Bayesian Inference
Information(2024)
摘要
This paper tackles the challenge of time series forecasting in the presence of missing data. Traditional methods often struggle with such data, which leads to inaccurate predictions. We propose a novel framework that combines the strengths of Generative Adversarial Networks (GANs) and Bayesian inference. The framework utilizes a Conditional GAN (C-GAN) to realistically impute missing values in the time series data. Subsequently, Bayesian inference is employed to quantify the uncertainty associated with the forecasts due to the missing data. This combined approach improves the robustness and reliability of forecasting compared to traditional methods. The effectiveness of our proposed method is evaluated on a real-world dataset of air pollution data from Mexico City. The results demonstrate the framework’s capability to handle missing data and achieve improved forecasting accuracy.
更多查看译文
关键词
time series,missing data,neural networks,GAN,Bayesian
AI 理解论文
溯源树
样例
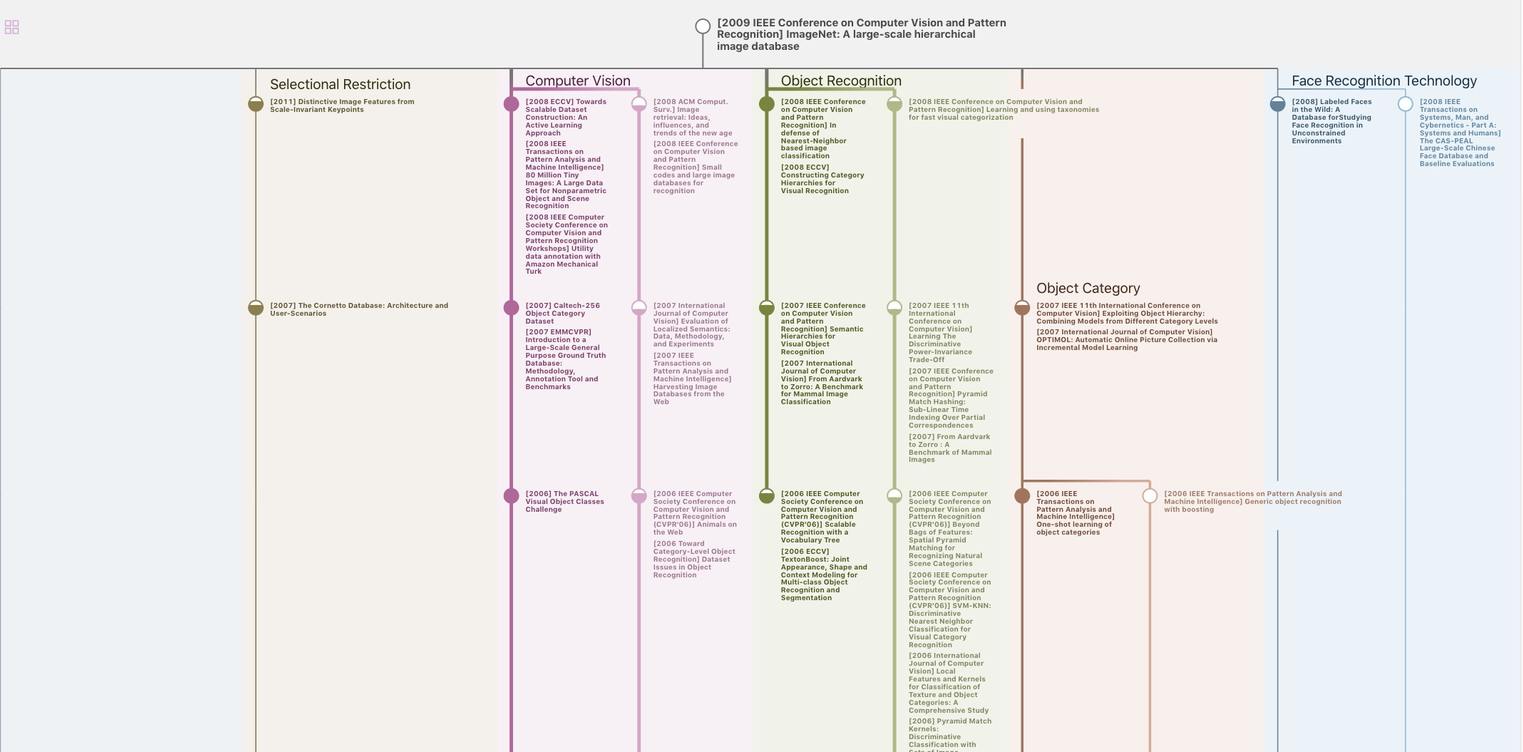
生成溯源树,研究论文发展脉络
Chat Paper
正在生成论文摘要