Predicting Chronic Liver Disease Using Boosting Technique
2023 International Conference on Artificial Intelligence for Innovations in Healthcare Industries (ICAIIHI)(2023)
摘要
Liver disease has become a major health crisis globally. Machine learning methodologies are increasingly being applied to predict and diagnose various diseases. This paper uses five boosting algorithms (XGBoost, CatBoost, LightGBM, AdaBoost, and gradient boosting) to predict liver disease. Several preprocessing procedures are utilised to enhance the prediction performance, in addition to the appropriate tuning of hyperparameters and selection of features. The model's performance is assessed using various metrics, including accuracy, precision, recall, fl-score, misclassification rate, AVC-ROC, and runtime. Among the five methods evaluated, gradient boosting emerged as the best performer, attaining the highest scores in nearly all performance metrics. It achieved an AVC-ROC of 86%, an accuracy of 87.43%, a precision of 86%, and a recall of 88.5%.
更多查看译文
关键词
Liver disease,disease prediction,ensemble learning,boosting techniques
AI 理解论文
溯源树
样例
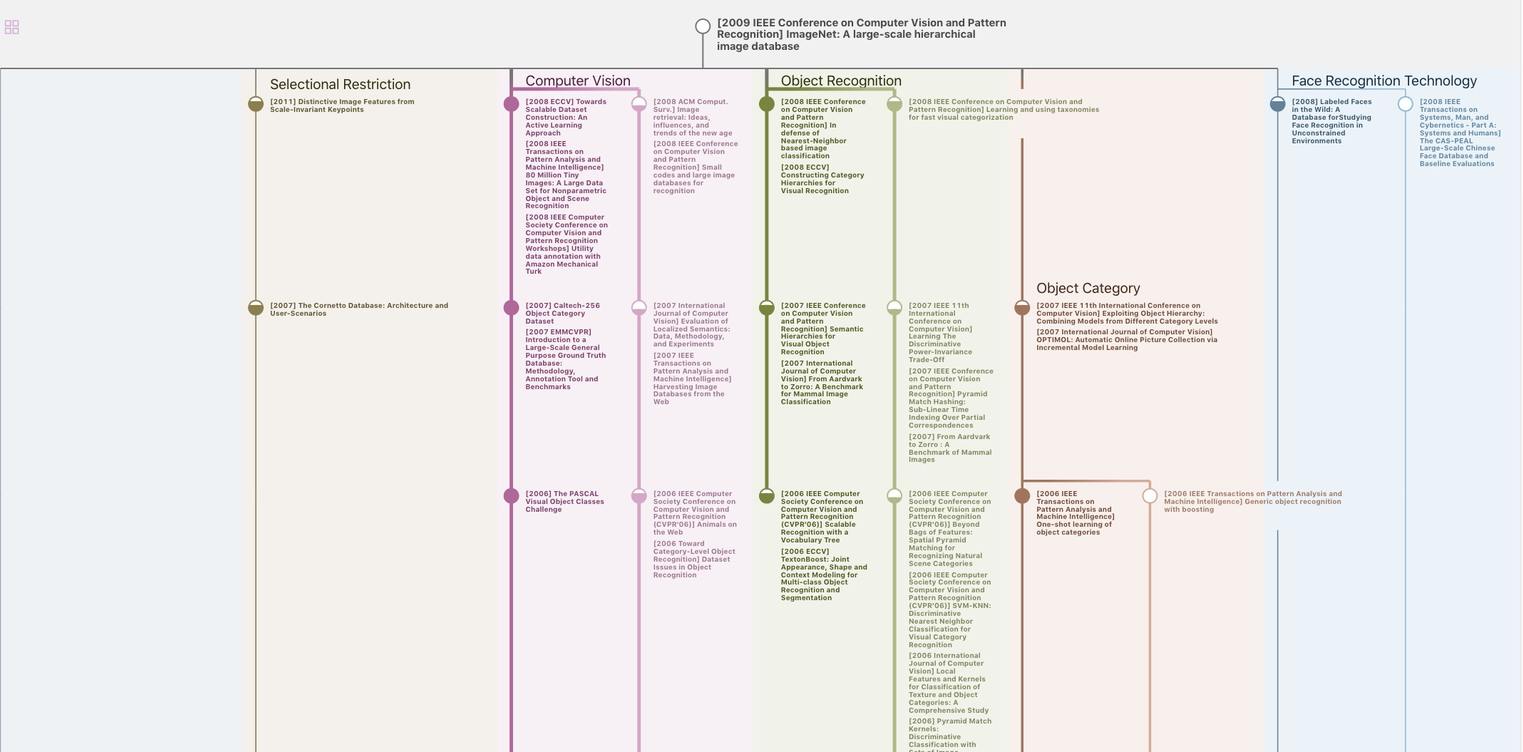
生成溯源树,研究论文发展脉络
Chat Paper
正在生成论文摘要