Improving Biological Joint Moment Estimation During Real-World Tasks with EMG and Instrumented Insoles.
IEEE transactions on bio-medical engineering(2024)
摘要
OBJECTIVE:Real-time measurement of biological joint moment could enhance clinical assessments and generalize exoskeleton control. Accessing joint moments outside clinical and laboratory settings requires harnessing non-invasive wearable sensor data for indirect estimation. Previous approaches have been primarily validated during cyclic tasks, such as walking, but these methods are likely limited when translating to non-cyclic tasks where the mapping from kinematics to moments is not unique.
METHODS:We trained deep learning models to estimate hip and knee joint moments from kinematic sensors, electromyography (EMG), and simulated pressure insoles from a dataset including 10 cyclic and 18 non-cyclic activities. We assessed estimation error on combinations of sensor modalities during both activity types.
RESULTS:Compared to the kinematics-only baseline, adding EMG reduced RMSE by 16.9% at the hip and 30.4% at the knee (p<0.05) and adding insoles reduced RMSE by 21.7% at the hip and 33.9% at the knee (p<0.05). Adding both modalities reduced RMSE by 32.5% at the hip and 41.2% at the knee (p<0.05) which was significantly higher than either modality individually (p<0.05). All sensor additions improved model performance on non-cyclic tasks more than cyclic tasks (p<0.05).
CONCLUSION:These results demonstrate that adding kinetic sensor information through EMG or insoles improves joint moment estimation both individually and jointly. These additional modalities are most important during non-cyclic tasks, tasks that reflect the variable and sporadic nature of the real-world.
SIGNIFICANCE:Improved joint moment estimation and task generalization is pivotal to developing wearable robotic systems capable of enhancing mobility in everyday life.
更多查看译文
关键词
deep learning,electromyography (EMG),human kinetics,inertial measurement units (IMUs),joint moments,machine learning,pressure insoles,temporal convolutional network
AI 理解论文
溯源树
样例
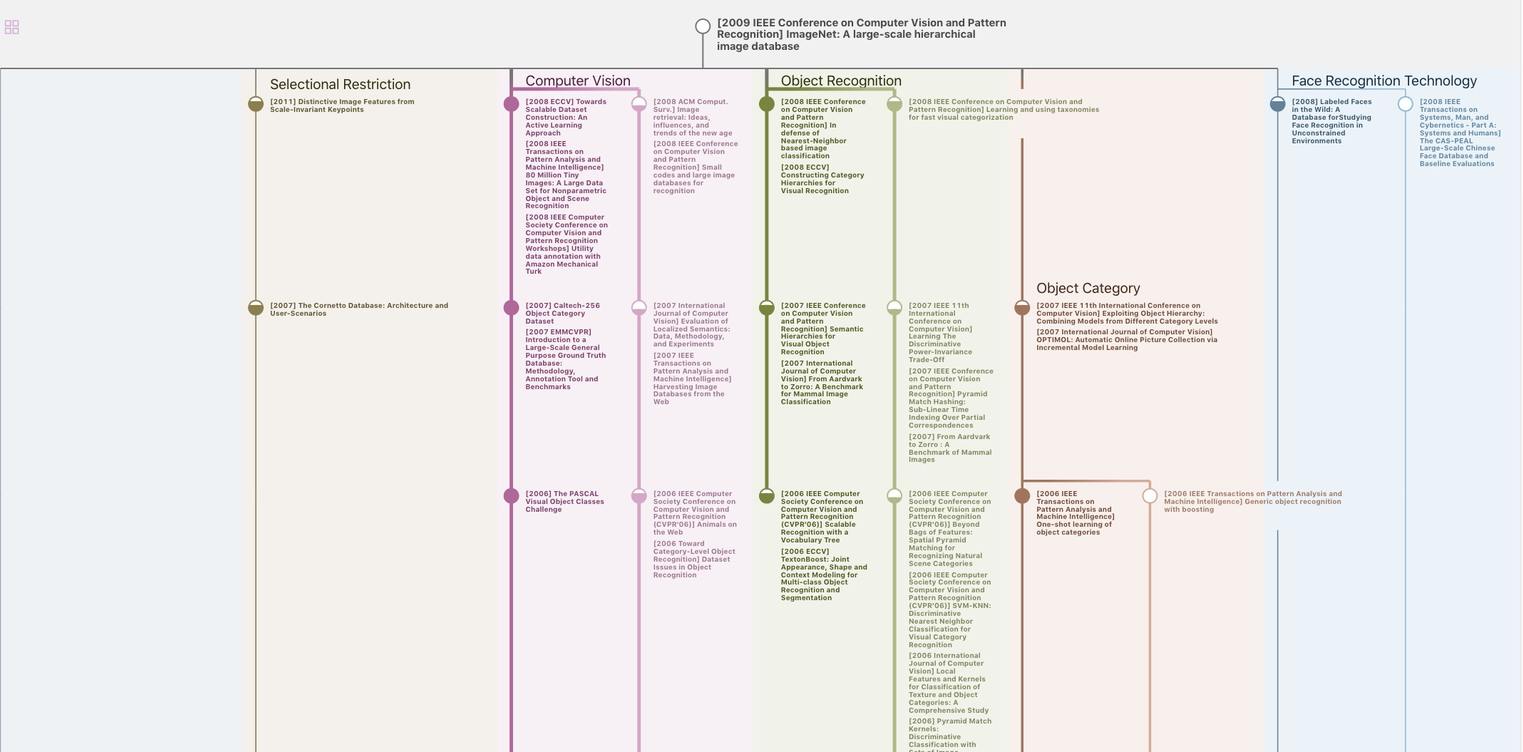
生成溯源树,研究论文发展脉络
Chat Paper
正在生成论文摘要