Semi-supervised multiview fuzzy broad learning
Information Sciences(2024)
摘要
Semi-supervised learning models often rely on restricted assumptions, and can easily suffer from covariate shift or noise. Few studies have investigated the use of fuzzy rule-based methods in the semi-supervised discipline. To improve model accuracy against covariate shift and to introduce fuzzy methods for interpretability, we first build a semi-supervised fuzzy broad learning model named MT-FBLS, which employs a Mean-Teacher framework. Then, a trusted multiview semi-supervised classification method, termed TMSSC, is proposed by integrating the MT-FBLS with a multiview fusion network to enhance the robustness of the model. Under the Mean-Teacher framework, MT-FBLS involves the Takagi-Sugeno-Kang fuzzy model which can effectively deal with imprecision and uncertainty, and broad learning system which has strong learning ability and high computational efficiency. TMSSC utilizes a trusted mechanism to blend multiple views, so as to enhance its learning ability in semi-supervised scenarios. Experiments on the benchmark datasets demonstrate that the proposed methods have better anti-noise ability, competitive classification accuracy, as well as fast running speed.
更多查看译文
关键词
Semi-supervised multi-view classification,Evidential multiview fusion,TSK fuzzy system,Fuzzy broad learning system
AI 理解论文
溯源树
样例
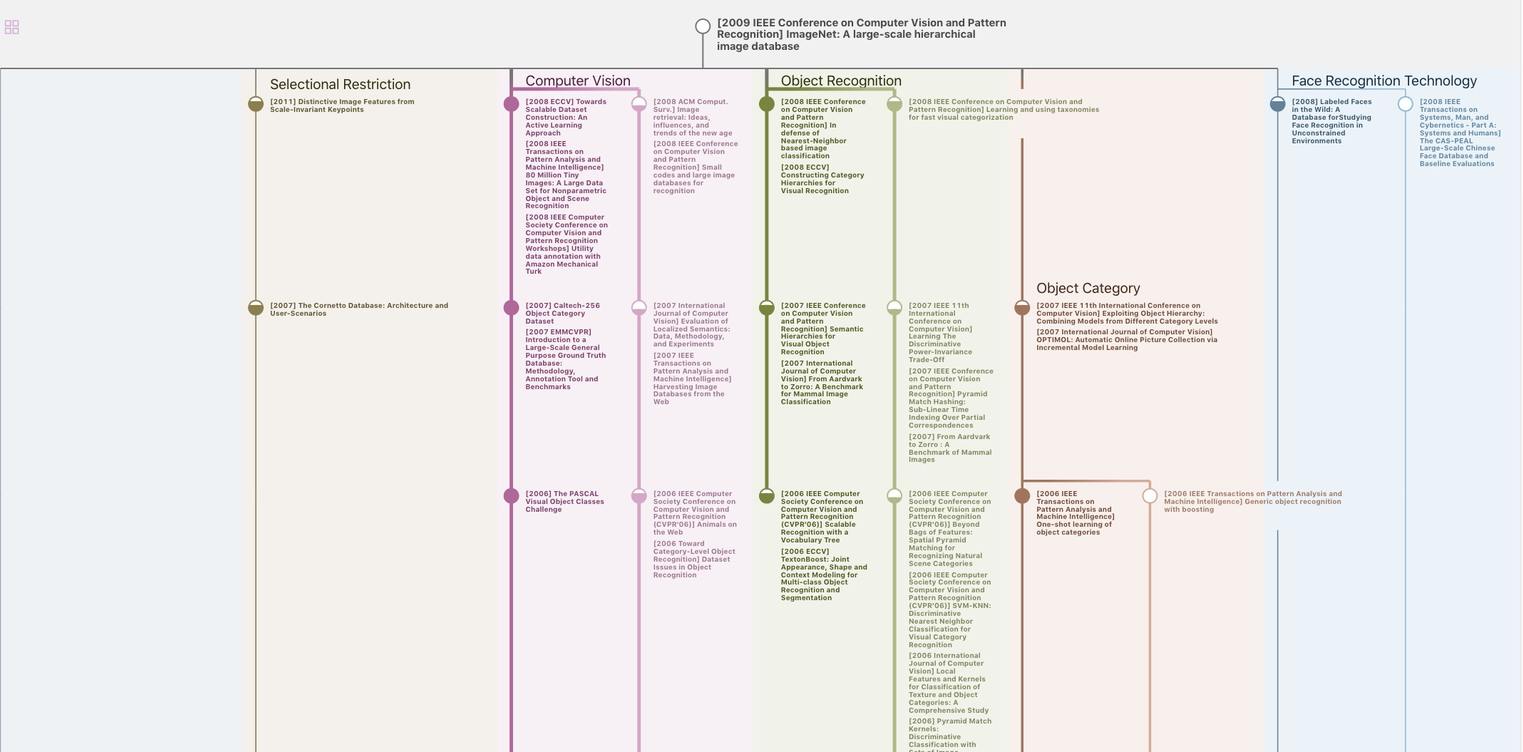
生成溯源树,研究论文发展脉络
Chat Paper
正在生成论文摘要