A Scalable Vertical Federated Learning Framework for Analytics in the Cybersecurity Domain.
International Euromicro Conference on Parallel, Distributed and Network-Based Processing(2024)
摘要
This paper presents a Scalable Vertical Federated Learning (SVFL) framework designed to address the task of clas-sification in the cybersecurity domain. SVFL combines vertical federated learning (VFL) with scalable computing architectures, enabling efficient analysis of large-scale and sensitive cybersecu-rity datasets while maintaining data confidentiality. The frame-work is adaptable to diverse use cases, scalable for increasing data volumes, and robust in dynamic and adversarial environments. In addition, adopting the VFL paradigm ensures a good trade-off between privacy and performance. Experimental results demon-strate the framework's effectiveness in enhancing collaborative threat detection and prevention, offering a promising solution for advancing cybersecurity analytics. Preliminary experiments conducted on a well-known cybersecurity dataset show that the accuracy of the systems does not degrade excessively compared to a baseline owning all the data locally.
更多查看译文
关键词
Federated Learning,Green AI,Cybersecurity,Classification
AI 理解论文
溯源树
样例
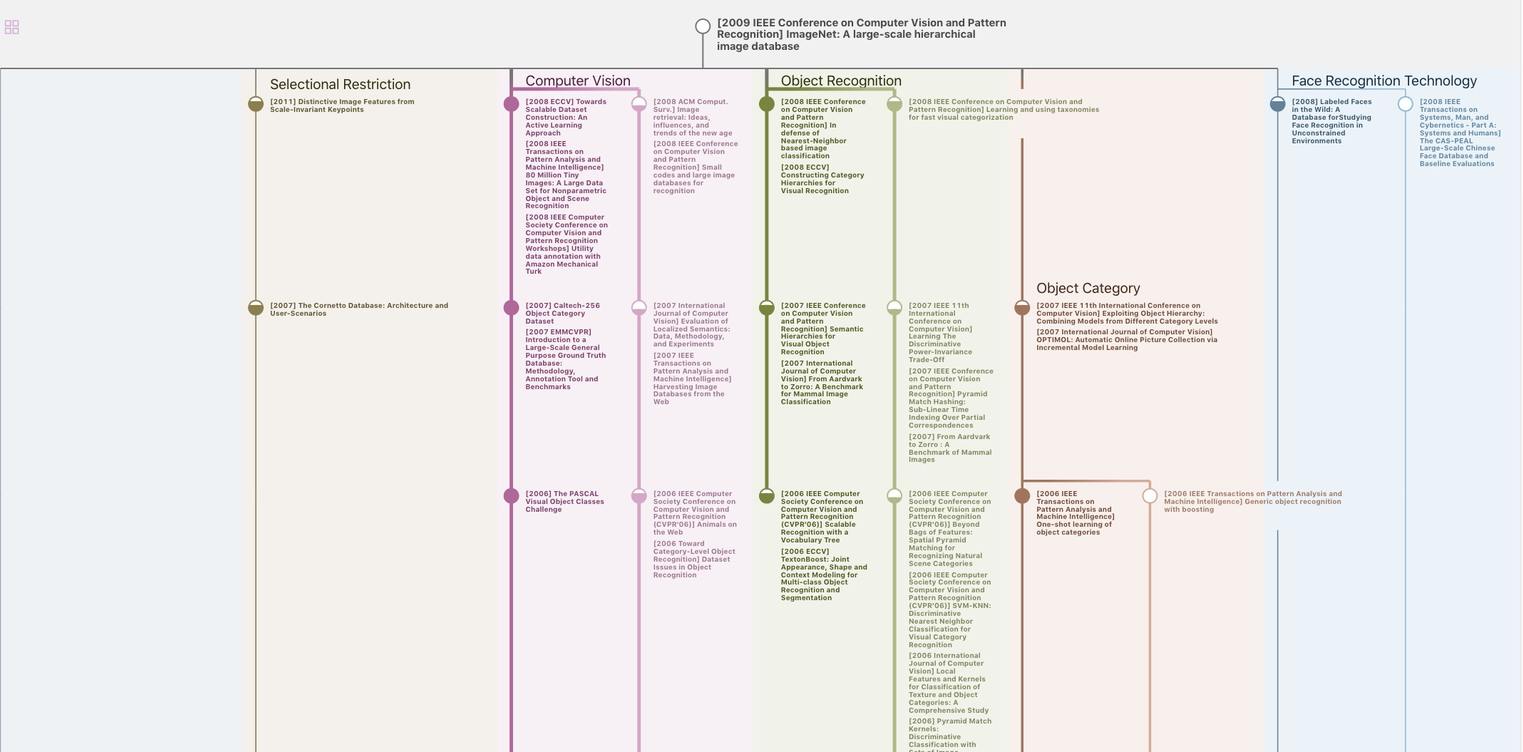
生成溯源树,研究论文发展脉络
Chat Paper
正在生成论文摘要