Evaluating the Explainability of Attributes and Prototypes for a Medical Classification Model
CoRR(2024)
摘要
Due to the sensitive nature of medicine, it is particularly important and
highly demanded that AI methods are explainable. This need has been recognised
and there is great research interest in xAI solutions with medical
applications. However, there is a lack of user-centred evaluation regarding the
actual impact of the explanations. We evaluate attribute- and prototype-based
explanations with the Proto-Caps model. This xAI model reasons the target
classification with human-defined visual features of the target object in the
form of scores and attribute-specific prototypes. The model thus provides a
multimodal explanation that is intuitively understandable to humans thanks to
predefined attributes. A user study involving six radiologists shows that the
explanations are subjectivly perceived as helpful, as they reflect their
decision-making process. The results of the model are considered a second
opinion that radiologists can discuss using the model's explanations. However,
it was shown that the inclusion and increased magnitude of model explanations
objectively can increase confidence in the model's predictions when the model
is incorrect. We can conclude that attribute scores and visual prototypes
enhance confidence in the model. However, additional development and repeated
user studies are needed to tailor the explanation to the respective use case.
更多查看译文
AI 理解论文
溯源树
样例
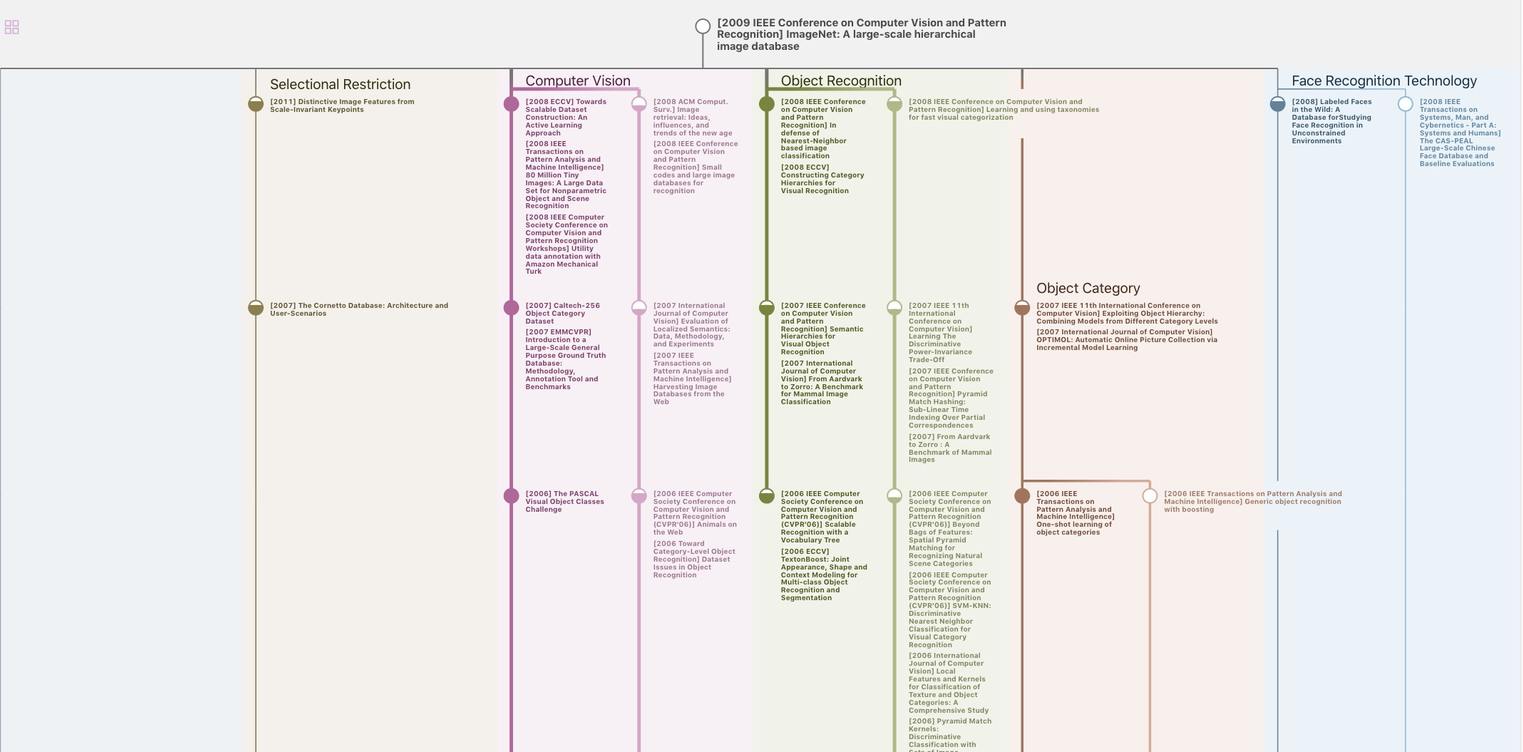
生成溯源树,研究论文发展脉络
Chat Paper
正在生成论文摘要