A Deep Learning Localization Method for Acoustic Source via Improved Input Features and Network Structure
Remote Sensing(2024)
摘要
Shallow water passive source localization is an essential problem in underwater detection and localization. Traditional matched-field processing (MFP) methods are sensitive to environment mismatches. Many neural network localization methods still have room for improvement in accuracy if they are further adjusted to underwater acoustic characteristics. To address these problems, we propose a deep learning localization method via improved input features and network structure, which can effectively estimate the depth and the closest point of approach (CPA) range of the acoustic source. Firstly, we put forward a feature preprocessing scheme to enhance the localization accuracy and robustness. Secondly, we design a deep learning network structure to improve the localization accuracy further. Finally, we propose a method of visualizing the network to optimize the estimated localization results. Simulations show that the accuracy of the proposed method is better than other compared features and network structures, and the robustness is significantly better than that of the MFP methods. Experimental results further prove the effectiveness of the proposed method.
更多查看译文
关键词
shallow water,neural network,feature preprocessing,matched-field processing,network visualization
AI 理解论文
溯源树
样例
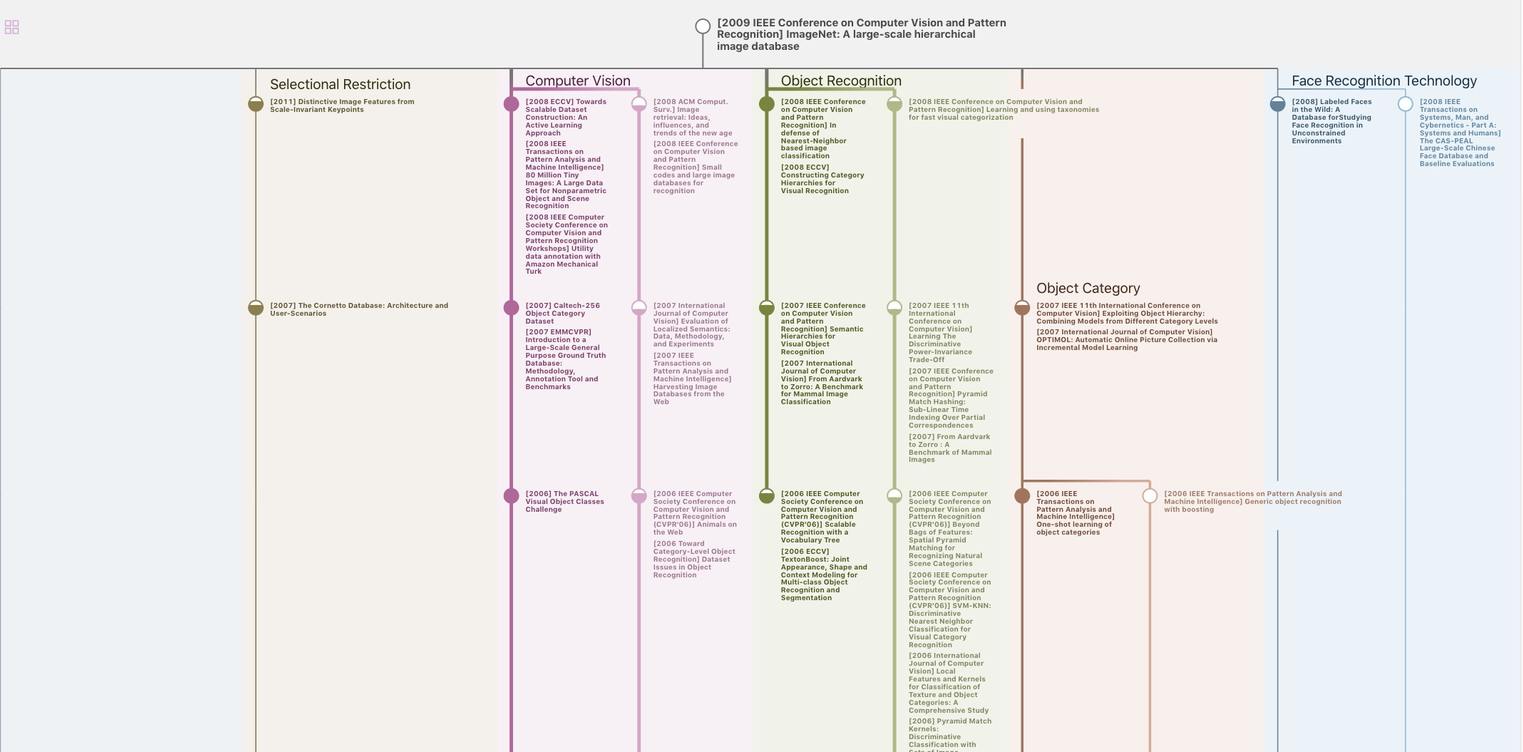
生成溯源树,研究论文发展脉络
Chat Paper
正在生成论文摘要