Predicting congenital syphilis: Using machine learning to enhance disease management and control
crossref(2024)
摘要
Objective Sexually Transmitted Infections (STIs) present significant challenges to global public health, affecting physical and mental well-being and straining healthcare systems and economies. This study aims to enhance the predictive performance of models for congenital syphilis prediction by incorporating additional information obtained during gestational follow-up. Building upon the work of Teixeira et al. [[1][1]], which utilizes clinical and sociodemographic data, our model was enriched with results from venereal disease research laboratory (VDRL) and rapid tests for congenital syphilis conducted on pregnant women.
Method The dataset utilized in this study comprised 47,604 records spanning the period from 2013 to 2022, with 27 attributes collected from pregnant women enrolled in the Mãe Coruja Pernambucana Program in Pernambuco, Brazil. Among these attributes, we included clinical and sociodemographic factors, as well as results from venereal disease research laboratory (VDRL) and rapid tests for congenital syphilis.
Results Our proposed model surpassed Teixeira’s models exhibiting higher specificity (94.74%) and a slight increase in sensitivity (70.37%).
Conclusions Our study highlights the value of incorporating additional information from VDRL and rapid tests into models for predicting congenital syphilis. The combined approach involving both clinical, sociodemographic, and test result data enhances the accuracy of predictions thereby facilitating better informed healthcare decisions at different stages of pregnancy. This approach also holds significant potential in combating and managing congenital syphilis by providing assistance to health system decision makers and public policymakers. As a result, it can ultimately enhance the overall outcomes of maternal and child health and contribute to disease control.
### Competing Interest Statement
The authors have declared no competing interest.
### Funding Statement
The author(s) received no specific funding for this work.
### Author Declarations
I confirm all relevant ethical guidelines have been followed, and any necessary IRB and/or ethics committee approvals have been obtained.
Yes
The details of the IRB/oversight body that provided approval or exemption for the research described are given below:
We declare that the research has been approved by the Brazilian Human Research Ethics Board (Comitê de Ética em Pesquisa [CEP]) under number 12438019.2.0000.5208 and all methods were performed in accordance with the Brazilian regulations that do not require consent for studies using unidentified data from the Brazilian data health systems.
I confirm that all necessary patient/participant consent has been obtained and the appropriate institutional forms have been archived, and that any patient/participant/sample identifiers included were not known to anyone (e.g., hospital staff, patients or participants themselves) outside the research group so cannot be used to identify individuals.
Yes
I understand that all clinical trials and any other prospective interventional studies must be registered with an ICMJE-approved registry, such as ClinicalTrials.gov. I confirm that any such study reported in the manuscript has been registered and the trial registration ID is provided (note: if posting a prospective study registered retrospectively, please provide a statement in the trial ID field explaining why the study was not registered in advance).
Yes
I have followed all appropriate research reporting guidelines, such as any relevant EQUATOR Network research reporting checklist(s) and other pertinent material, if applicable.
Yes
All data are available from the Mendeley Data database (accession number(s) doi:10.17632/3zkcvybvkz.2).
[1]: #ref-1
更多查看译文
AI 理解论文
溯源树
样例
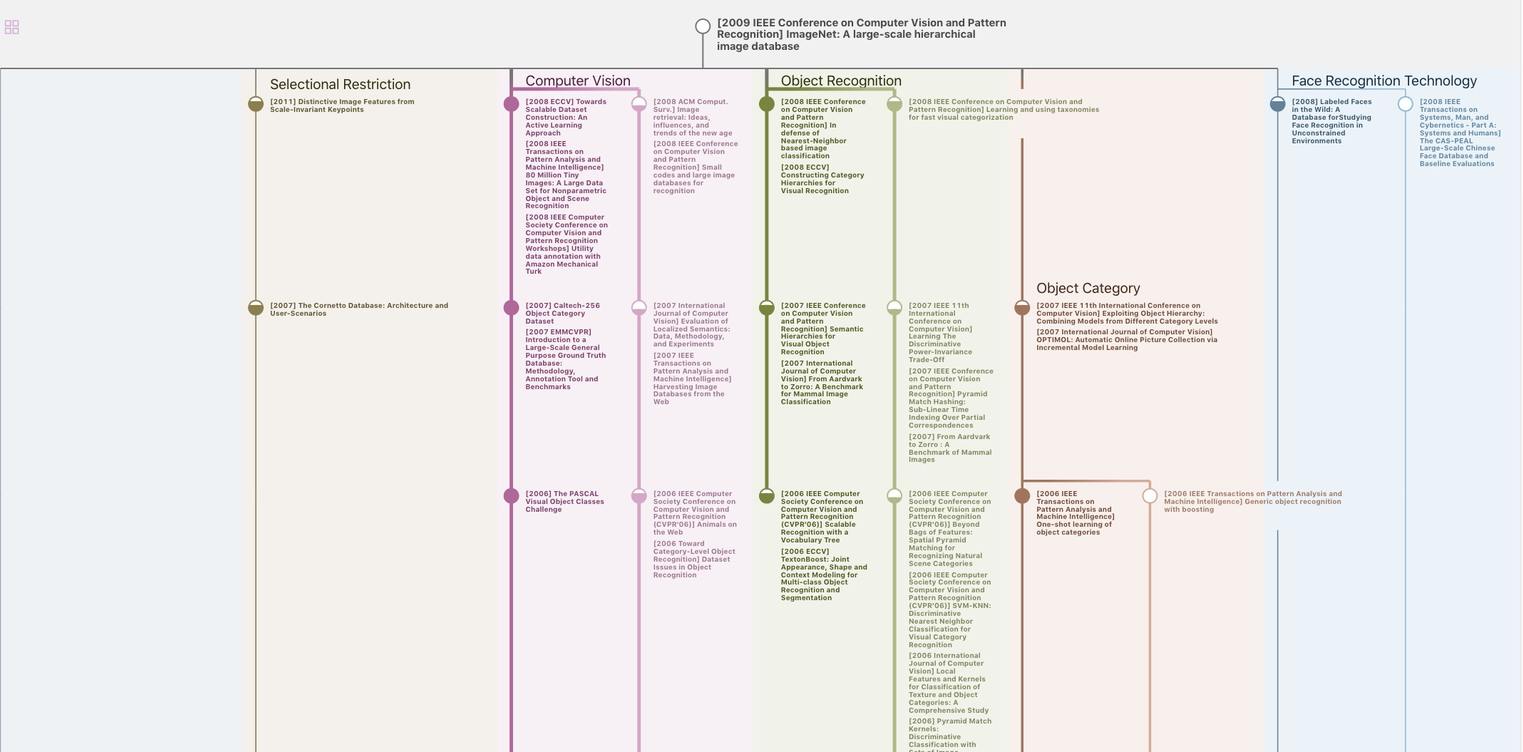
生成溯源树,研究论文发展脉络
Chat Paper
正在生成论文摘要