A lightweight Transformer-based neural network for large-scale masonry arch bridge point cloud segmentation
COMPUTER-AIDED CIVIL AND INFRASTRUCTURE ENGINEERING(2024)
摘要
Transformer architecture based on the attention mechanism achieves impressive results in natural language processing (NLP) tasks. This paper transfers the successful experience to a 3D point cloud segmentation task. Inspired by newly proposed 3D Transformer neural networks, this paper introduces a new Transformer-based module, which is called Local Geo-Transformer. To alleviate the heavy memory consumption of the original Transformer, Local Geo-Transformer only performs the attention mechanism in local regions. It is designed to mitigate the information loss caused by the subsampling of point clouds for segmentation. Global Geo-Transformer is proposed to exploit the global relationships between features with the lowest resolution. The new architecture is validated on a masonry bridge dataset developed by the authors for their earlier work on a previous segmentation network called BridgeNet. The new version of the network with Transformer architecture, BridgeNetv2, outperforms BridgeNet in all metrics. BridgeNetv2 is also shown to be lightweight and memory efficient, well-adapted to large-scale point cloud segmentation tasks in civil engineering.
更多查看译文
AI 理解论文
溯源树
样例
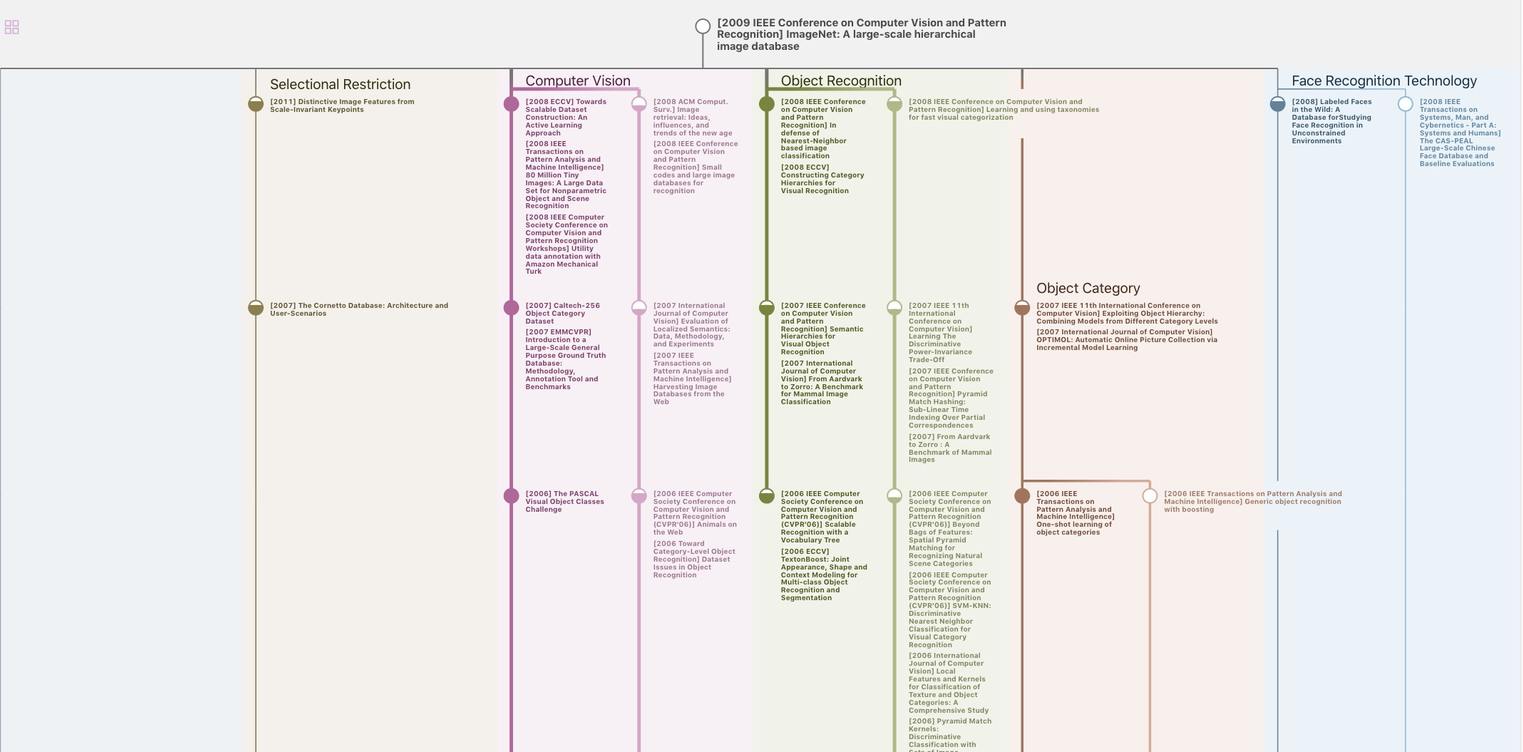
生成溯源树,研究论文发展脉络
Chat Paper
正在生成论文摘要