An energy-efficient unrelated parallel machine scheduling problem with learning effect of operators and deterioration of jobs
International Journal of Environmental Science and Technology(2024)
摘要
In the realm of energy-efficient production scheduling, numerous multi-objective optimization models and metaheuristic solutions have been proposed. However, there remains a gap in the literature concerning the consideration of job deterioration and the learning effects of operators. To address this research gap, this study presents a redefined energy-efficient unrelated parallel machine scheduling problem, incorporating both job deterioration and learning effects of operators. Additionally, our proposed model aims to simultaneously minimize multiple objectives, including makespan, total weighted flow time, tardiness, and energy consumption. By employing the concept of multi-objective optimization, we solve small instances using an augmented epsilon-constraint method, while larger instances are tackled using popular multi-objective metaheuristics, such as the non-dominated sorting genetic algorithm (NSGA-II) and multi-objective particle swarm optimization (MOPSO). The effectiveness of our solution algorithms is assessed through various multi-objective metrics, and extensive sensitivity analyses are conducted on the optimization model. Ultimately, this study concludes by offering comprehensive insights for practical implementation in production scheduling systems. Our findings contribute significantly to the advancement of energy-efficient production scheduling studies, highlighting the importance of considering job deterioration and the learning effects of operators in real-world scenarios.
更多查看译文
关键词
Energy-efficient scheduling,Unrelated parallel machine,Learning effect,Deterioration of jobs,Metaheuristic algorithms
AI 理解论文
溯源树
样例
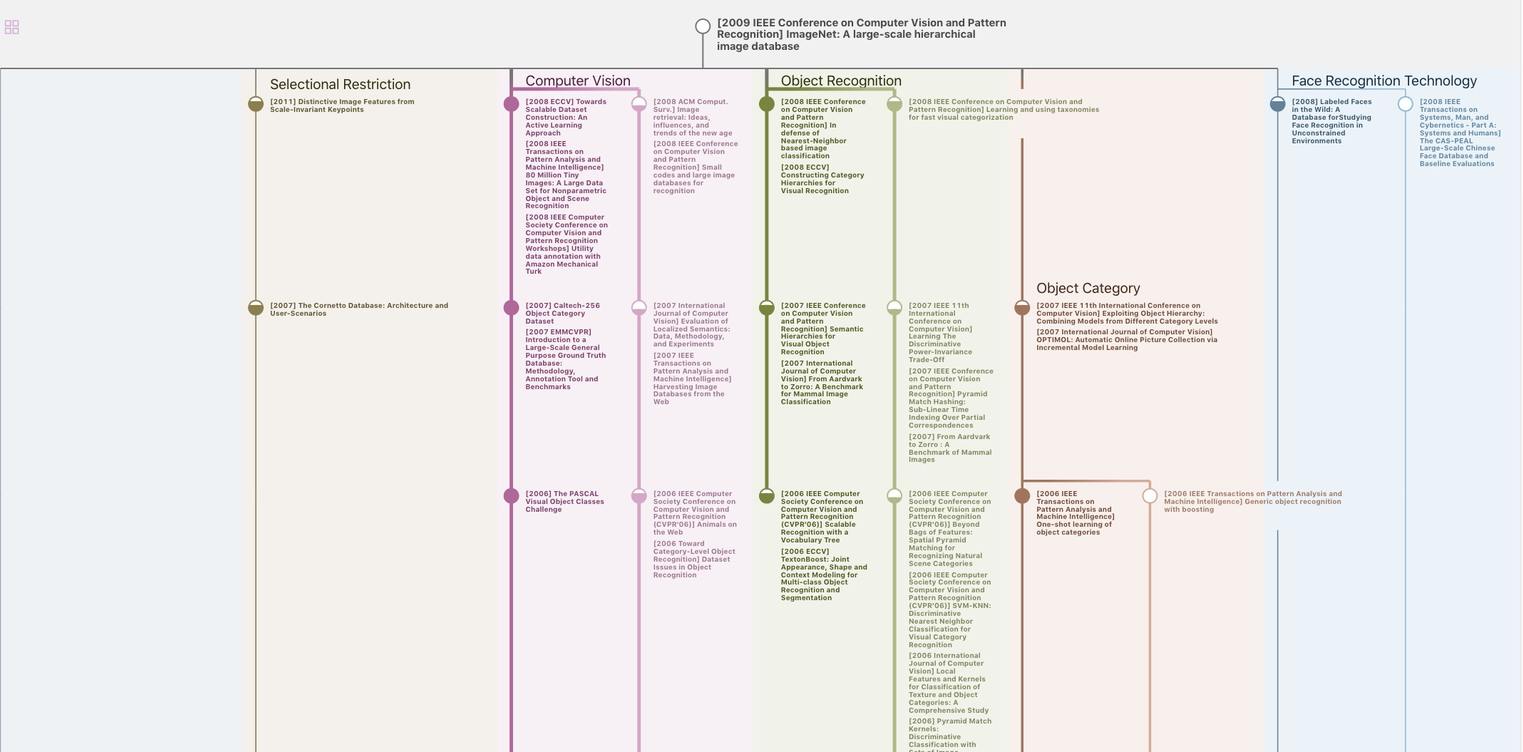
生成溯源树,研究论文发展脉络
Chat Paper
正在生成论文摘要