Linear Parameter-Varying Model Predictive Control for Intelligent Energy Management in Battery/Supercapacitor Electric Vehicles
IEEE Access(2024)
摘要
This paper presents a supervisory control strategy to intelligently split power within a hybrid energy storage system (HESS) of battery and supercapacitor (SC) in electric vehicles (EVs). The intelligent energy management strategy (IEMS) is based on linear parameter-varying model predictive control (LPV-MPC) with the main aim of mitigating battery degradation. The battery RC model is considered in the control-oriented model, where the increment of the battery current is selected as the control variable, resulting in a linear state-space model dependent on the HESS parameters, namely, the battery state-of-charge (SoC) and SC state-of-voltage (SoV). The cost function minimizes the power loss in the battery and squared error of the SoV across the prediction horizon. A new SoV control strategy is proposed based on the upcoming acceleration, providing opportunities for the efficient utilization of the SC and extending the battery lifespan. Constrained optimization is transformed into a quadratic programming problem, which can be easily solved in real time. The superiority of the proposed method in assessing battery degradation was verified by comparing different strategies using five evaluation factors under two drive cycles. Compared with the LPV-MPC, where SoV remained fixed, the proposed method demonstrates reductions of up to 18.82% in the battery current root-mean-square, 30.26% and 25.85% in the discharge and charge peak current, 9.71% in the ampere-hour throughput, 4.78% in the capacity loss, and 29.06% in the energy loss. To validate the real time performance of the proposed method, it is implemented in the real-time digital simulator (RTDS).
更多查看译文
关键词
Batteries,electric vehicle,energy management,hybrid energy storage system,linear systems,model predictive control
AI 理解论文
溯源树
样例
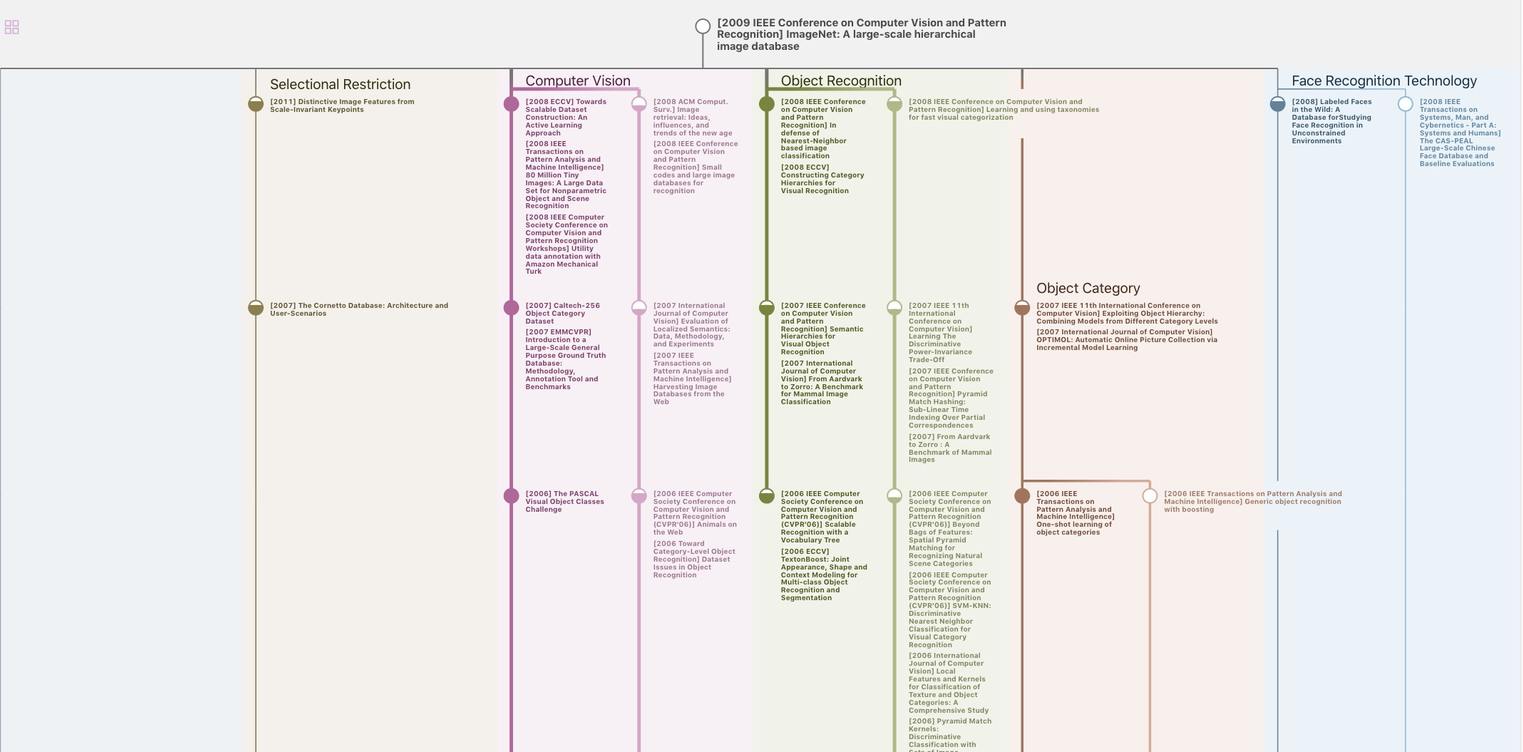
生成溯源树,研究论文发展脉络
Chat Paper
正在生成论文摘要