Quantum molecular docking with quantum-inspired algorithm
arxiv(2024)
摘要
Molecular docking (MD) is a crucial task in drug design, which predicts the
position, orientation, and conformation of the ligand when bound to a target
protein. It can be interpreted as a combinatorial optimization problem, where
quantum annealing (QA) has shown promising advantage for solving combinatorial
optimization. In this work, we propose a novel quantum molecular docking (QMD)
approach based on QA-inspired algorithm. We construct two binary encoding
methods to efficiently discretize the degrees of freedom with exponentially
reduced number of bits and propose a smoothing filter to rescale the rugged
objective function. We propose a new quantum-inspired algorithm, hopscotch
simulated bifurcation (hSB), showing great advantage in optimizing over
extremely rugged energy landscapes. This hSB can be applied to any formulation
of objective function under binary variables. An adaptive local continuous
search is also introduced for further optimization of the discretized solution
from hSB. Concerning the stability of docking, we propose a perturbation
detection method to help ranking the candidate poses. We demonstrate our
approach on a typical dataset. QMD has shown advantages over the search-based
Autodock Vina and the deep-learning DIFFDOCK in both re-docking and
self-docking scenarios. These results indicate that quantum-inspired algorithms
can be applied to solve practical problems in the drug discovery even before
quantum hardware become mature.
更多查看译文
AI 理解论文
溯源树
样例
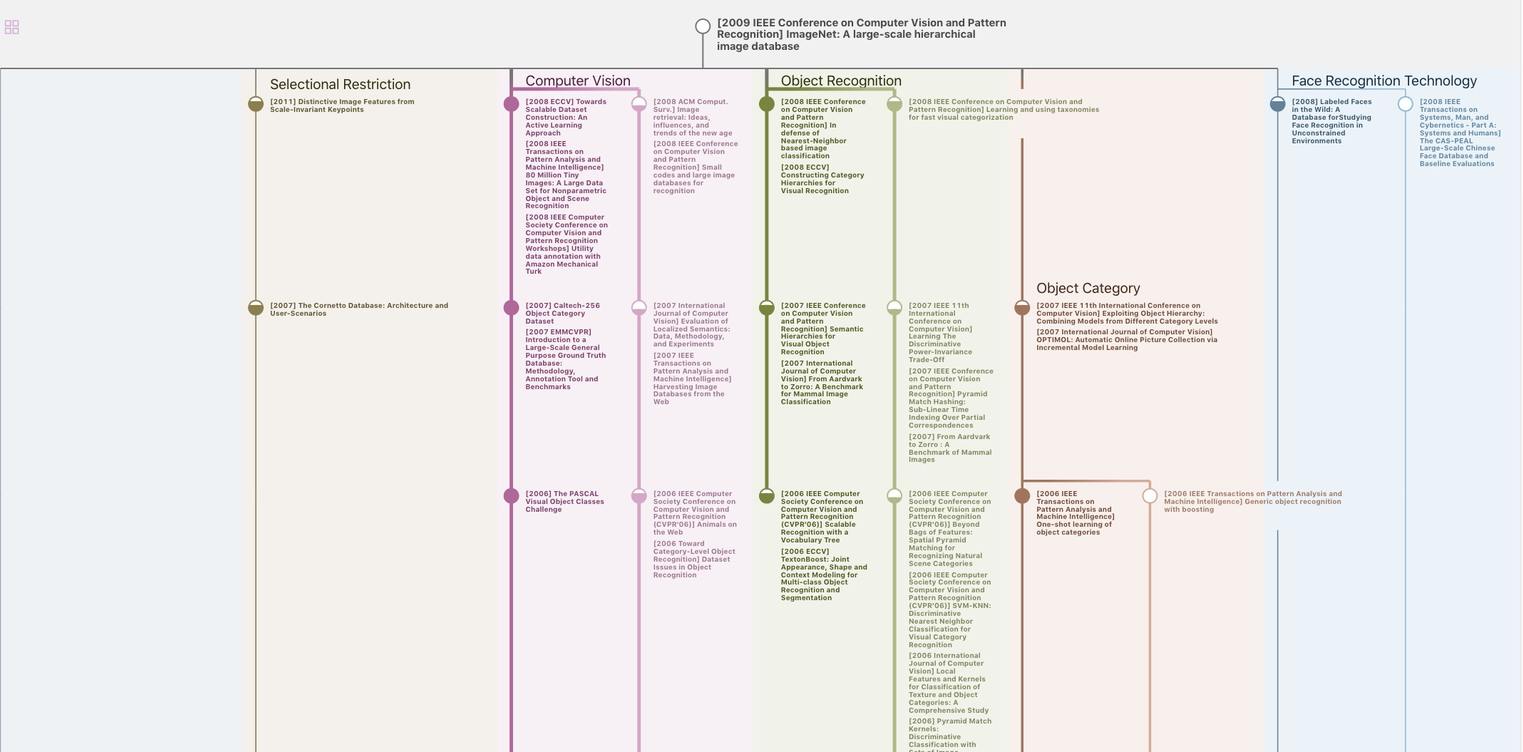
生成溯源树,研究论文发展脉络
Chat Paper
正在生成论文摘要